Damage identification in concrete structures using a hybrid time–frequency decomposition of acoustic emission responses
Journal of Civil Structural Health Monitoring(2024)
摘要
Concrete structures are subjected to various levels of damage during their life cycle due to exposure to different environmental and loading conditions. Hence, damage severity prediction and localization in concrete elements remain critical tasks to provide optimal maintenance in a timely manner, leading to reduced maintenance costs and avoiding any catastrophic failure that may cause serious loss and danger to the infrastructure owners. The Acoustic Emission (AE) technique is one of the powerful nondestructive testing (NDT) methodologies that can be used to predict the severity of damage and localize it in different systems. This paper proposes a damage identification approach by combining Multivariate Empirical Mode Decomposition (MEMD) and the Gaussian Mixture Model (GMM). Unlike existing methods that rely on a single AE measurement, MEMD is used to extract the key AE components that belong to cracks using AE data collected from multiple sensors. MEMD is an extended version of EMD that can analyze multichannel AE signals collected from different sensors. The statistical features (e.g., mean, root mean square, kurtosis, skewness, and crest factor) of AE components obtained from MEMD are then subsequently estimated. The selected features are used in the GMM model to predict the severity and qualitative location of the damage. The proposed method is validated using a suite of experimental studies and AE data collected from a full-scale concrete dam located in Ontario, Canada. The results show that the proposed approach can accurately predict the severity of damage and identify the potential location of damage and prove that the proposed method can be suitable for robust damage identification in concrete structures.
更多查看译文
关键词
Acoustic emission,Damage severity,Damage localization,Concrete structures,MEMD,GMM
AI 理解论文
溯源树
样例
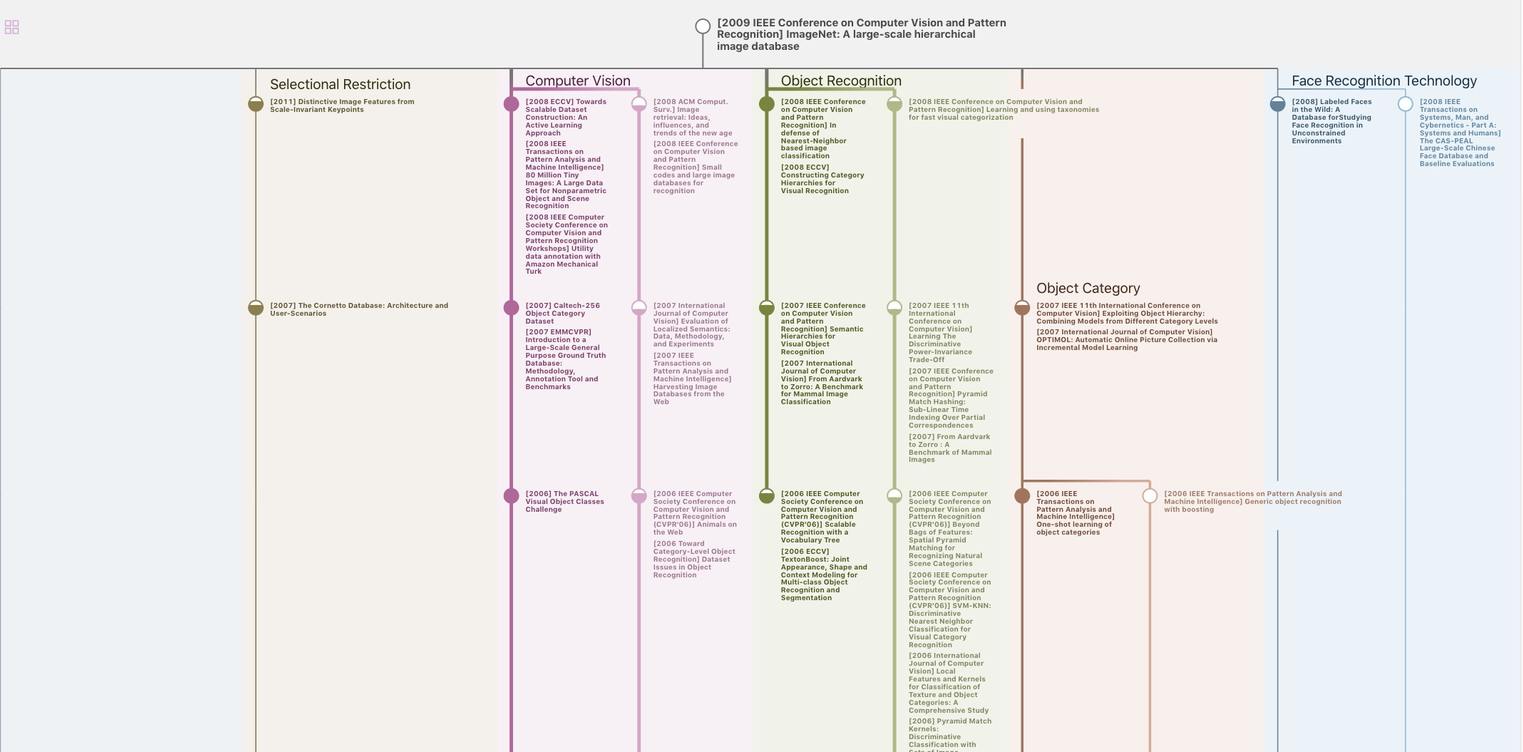
生成溯源树,研究论文发展脉络
Chat Paper
正在生成论文摘要