Privacy-Preserving Domain Adaptation for Intracranial EEG Classification via Information Maximization and Gaussian Mixture Model
IEEE SENSORS JOURNAL(2023)
摘要
Automated deep learning methods for classifying intracranial electroencephalography (iEEG) recordings into three categories (artifacts, pathological activities, and physiological activities) have gained increasing attention. However, most efforts have paid little attention to individual differences, where domain shift issues frequently arise when deploying models trained on prior patients (source domain) to other individuals (target domain). While unsupervised domain adaptation methods can be used to address the domain shift issues in iEEG classification, their application requires access to source data, which raises privacy concerns. To concurrently account for individual differences and patient data privacy, we propose a novel privacy-preserving domain adaptation (PPDA) approach, which only needs the source model for domain adaptation without accessing the source data. PPDA assumes that once a model with adaptive ability is obtained in the target domain, the ideal outputs of the model for each target sample should be deterministic, i.e., entropy minimization. Thus, PPDA uses information maximization (IM) to encourage the generation of one-hot predictions for target outputs, where the target samples with the same prediction are clustered in the feature space and assigned to a certain class. Then, the Gaussian mixture model (GMM)-based pseudolabeling is used to mitigate the occurrence of misclassification by IM and reduce the adverse effects of inaccurate pseudo labels, which improves the adaptation performance. Experimental results on two public iEEG datasets and representative models demonstrate the generality and superiority of our method. It is a promising solution to reduce individual differences and preserve patient privacy for iEEG classification.
更多查看译文
关键词
Adaptation models,Data models,Feature extraction,Data privacy,Predictive models,Brain modeling,Sensors,Domain adaptation,Gaussian mixture model (GMM),information maximization (IM),intracranial electroencephalogram classification,patient privacy-preserving
AI 理解论文
溯源树
样例
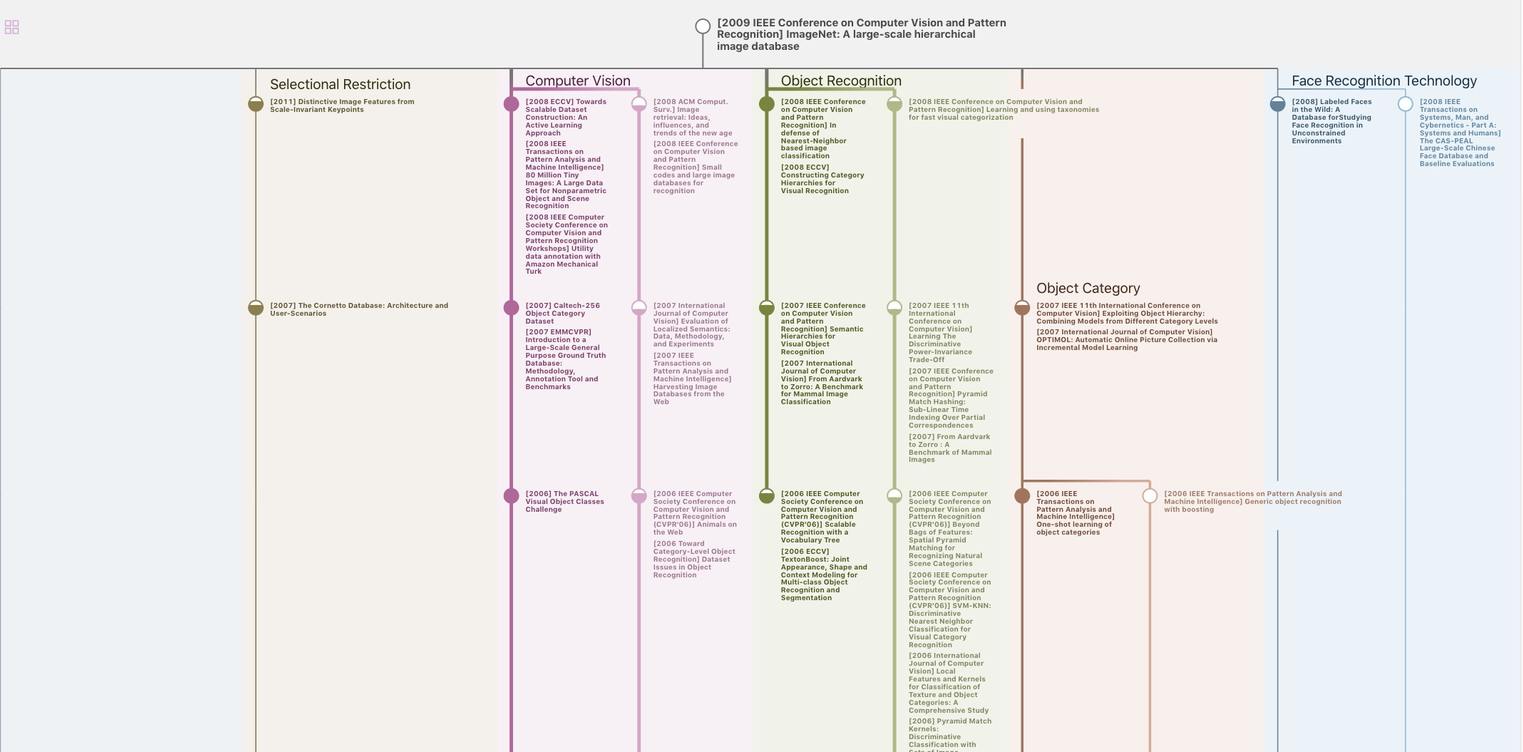
生成溯源树,研究论文发展脉络
Chat Paper
正在生成论文摘要