Teaching Machine Learning with Industrial Projects in a Joint Computer Science Master Course: Experiences, Challenges, Perspectives
2023 IEEE 2ND GERMAN EDUCATION CONFERENCE, GECON(2023)
摘要
Teaching machine learning is not only a balancing act between an appropriate amount of theory and practice. Beside relevant classic methods, it further needs to mind the state-of-the-art in a rapidly evolving field. Project-based learning approaches can aid translation of theory into practice, and serve as playground for modern methods. In addition, they foster advancement of various skills, crucial for working in teams. Ideally, projects topically align with study programs they are offered to, and address current and relevant problems. Joint courses offered to different programs thus display a demanding environment. The paper at hand presents insights into an implemented approach for a respective course environment, involving industrial projects of small extent with differentiated topics for various computer science Master programs. Students get in touch with industrial partners and apply in vogue methods, while traversing the Cross-Industry Standard Process for Data Mining (CRISP-DM), respectively large parts of its counterpart for Machine Learning with Quality Assurance Methodology (CRISP-ML(Q)). Gathered experiences from student surveys from two iterations are reported, and challenges associated with conduction of the approach and project fulfillment are outlined. Further, perspectives on future course and approach adjustments are presented. A discussion states limitations in regards to its implementation and potential scope, and addresses emerging trends to mind. It concludes that once implemented, the approach aids program-specific teaching, proves scalable regarding provision to further programs, and can adapt to current progress in the field.
更多查看译文
关键词
project-based learning,project-based teaching,industrial projects,machine learning education,joint graduate course,CRISP-DM,CRISP-ML(Q)
AI 理解论文
溯源树
样例
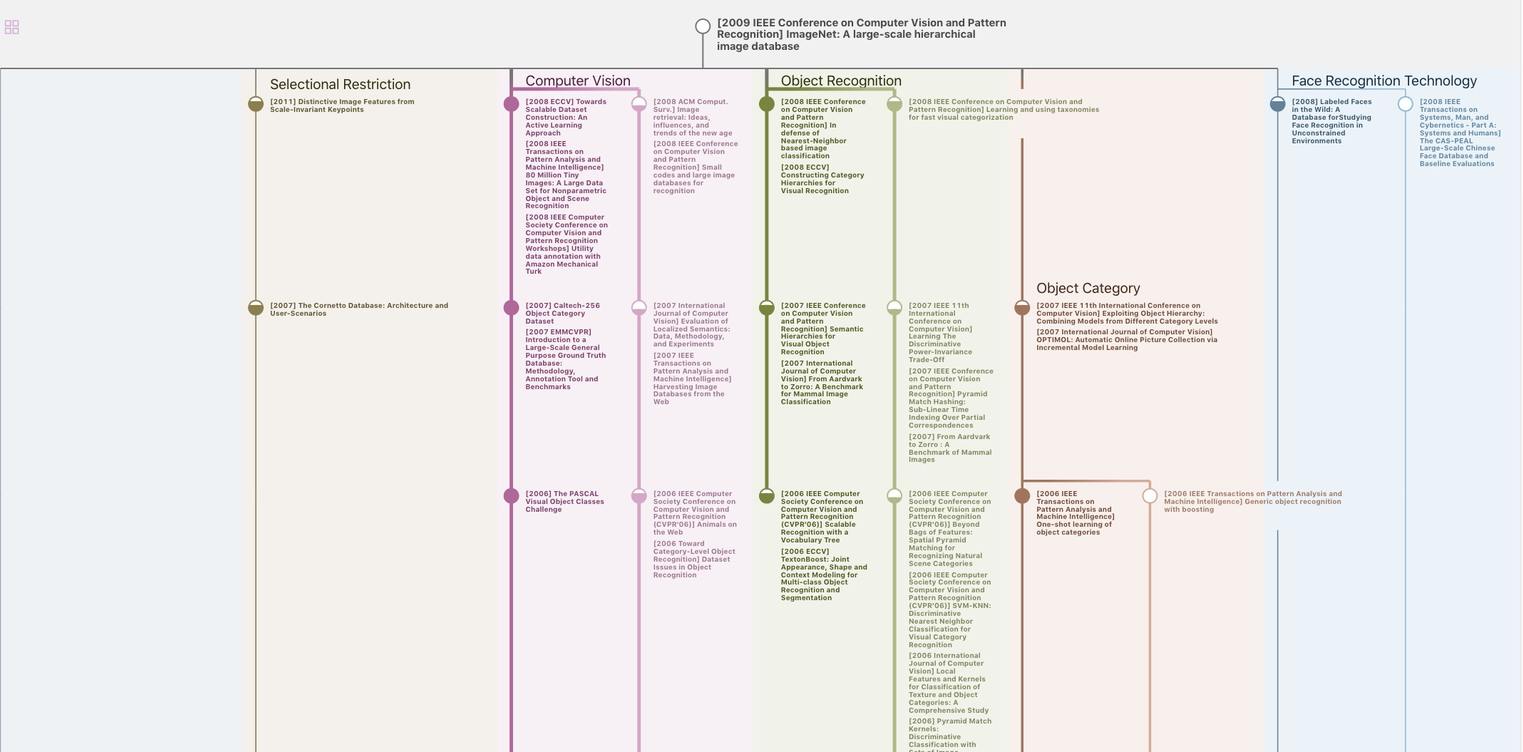
生成溯源树,研究论文发展脉络
Chat Paper
正在生成论文摘要