Routability-Driven Power Distribution Network Synthesis with IR-Drop Budgeting
2023 ACM/IEEE 5th Workshop on Machine Learning for CAD (MLCAD)(2023)
摘要
Power distribution network (PDN) interferes with signal routing, in particular when the network is dense to support low supply voltage (Vdd) or tight IR-drop constraint. We address post-placement PDN synthesis with a goal of minimizing PDN strap width sums while IR-drop constraint is respected. The problem can be formulated as mixed-integer quadratically constrained programming (MIQCP) as we present. But PDN synthesis with MIQCP is not scalable if the network is formulated as a whole. Our solution consists of two steps: (1) A conditional generative adversarial network (CGAN) model is developed. It outputs a map of IR-drop budgets (intermediate target voltages) with routing congestion map and current map taken as inputs. (2) MIQCP is applied for each PDN metal layer one by one, with much lower computational complexity. Experiments on a few test circuits indicate that the proposed approach leads to 40% reduction in congestion overflow, compared to state-of-the-art.
更多查看译文
关键词
power distribution network,IR-drop,machine learning
AI 理解论文
溯源树
样例
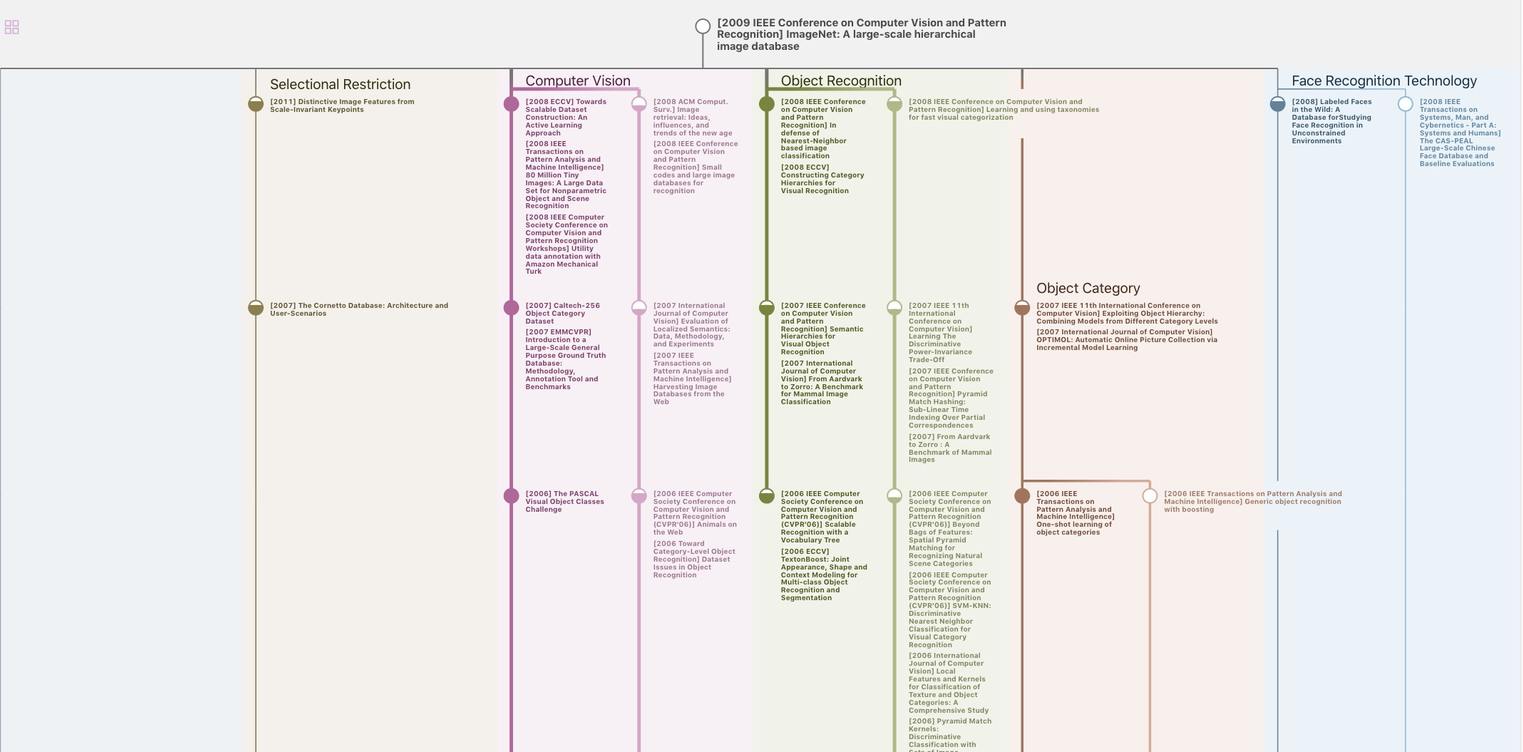
生成溯源树,研究论文发展脉络
Chat Paper
正在生成论文摘要