Dual Semantic Enhanced Event Causality Identification with Derivative Temporal Prompt
2023 5th International Conference on Data-driven Optimization of Complex Systems (DOCS)(2023)
摘要
Event causality identification (ECI) is a crucial task in natural language processing (NLP) that aims to identify the causal relation between a pair of events in a sentence. However, the existing methods still face two main challenges: a deficiency in causal reasoning capabilities, particularly in detecting implicit causality, and a scarcity of adequately annotated data for training causal relation patterns. In this paper, we propose an innovative approach to address these challenges. On the one hand, we introduce a derivative temporal relation identification task to enhance the model's capability to capture temporal information, which can be utilized to improve the identification of implicit causality. On the other hand, our method incorporates dual semantic information to enhance the representation of events. Firstly, we leverage semantic knowledge relevant to event pairs from external knowledge bases. Secondly, we utilize a dependency-based semantic enhancement module to extract comprehensive semantic information from events within the sentence. We evaluate our method on two benchmark datasets, and the results demonstrate its superior performance compared to previous state-of-the-art methods.
更多查看译文
关键词
event causality identification,semantic enhancement,derivative task,dependency parsing
AI 理解论文
溯源树
样例
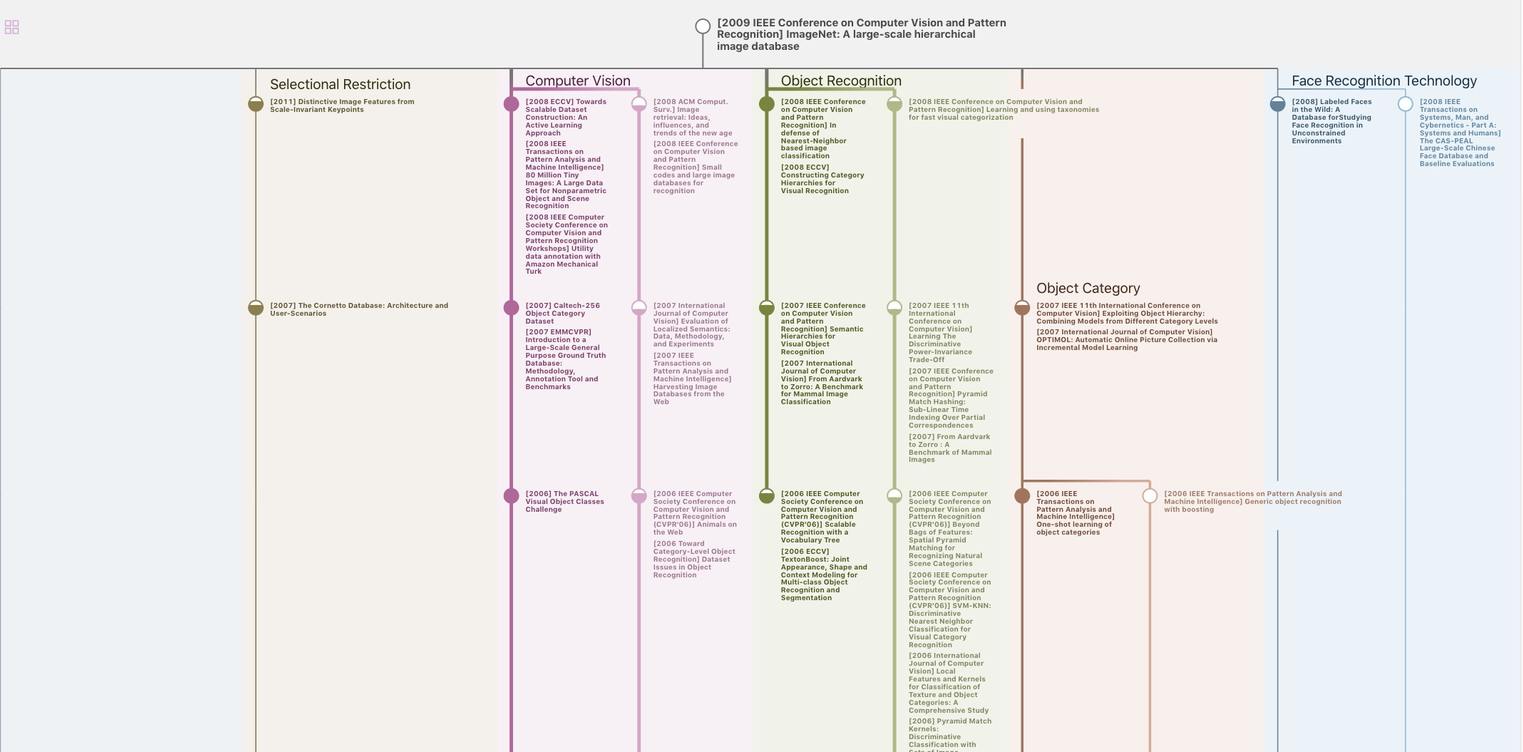
生成溯源树,研究论文发展脉络
Chat Paper
正在生成论文摘要