A Constrained Multi-Objective Evolutionary Algorithm Based on Early Convergence Followed by Diversity
2023 5th International Conference on Data-driven Optimization of Complex Systems (DOCS)(2023)
摘要
Many real-world optimization problems can be formulated as a kind of constrained multi-objective optimization problems (CMOPs). The main difficulty in solving these problems is to take feasibility, convergence and diversity into account simultaneously. To address this issue, this paper proposes a push and pull search algorithm based on early convergence followed by diversity (PPS-CFD). The proposed algorithm is composed of three different stages, each respectively focusing on convergence, diversity and feasibility. In the first stage, the population rapidly converges to the unconstrained Pareto front (UPF) in
$M$
directions, where
$M$
is the number of objectives of CMOPs. In the second stage, the population further converges to the UPF and meanwhile its diversity is enhanced. In the last stage, constraints are taken into account to pull the population from the UPF to the constrained Pareto front (CPF). In addition, a search strategy based on objective space division is proposed at the last two stages. Finally, the proposed PPS-CFD is tested on fourteen benchmark problems, compared with other six algorithms, to demonstrate its superiority.
更多查看译文
关键词
Constrained multi-objective optimization,push and pull search framework,objective space division
AI 理解论文
溯源树
样例
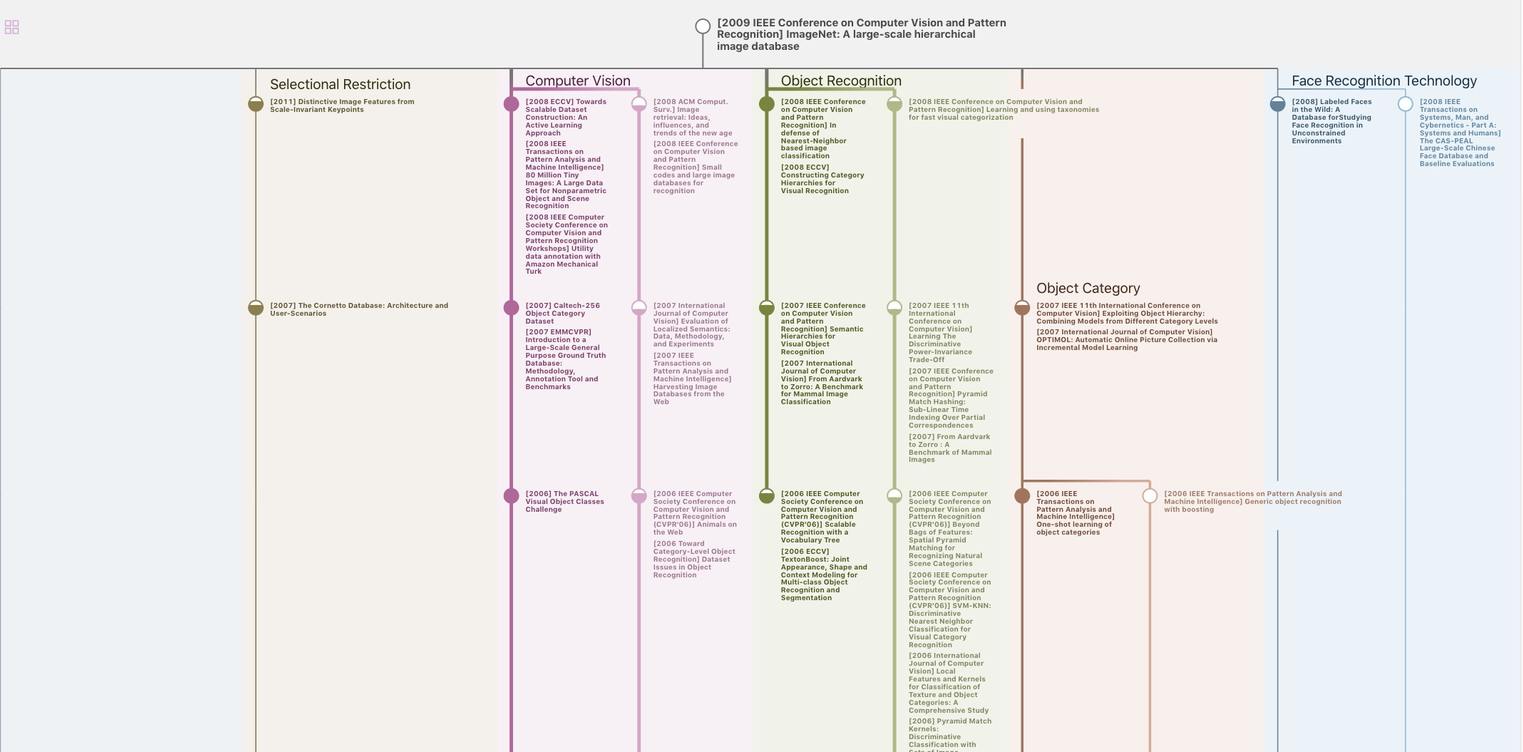
生成溯源树,研究论文发展脉络
Chat Paper
正在生成论文摘要