Direct Against Indirect Short-Term Net Load Forecasting Using Machine Learning Principles for Renewable Microgrids
ISC2(2023)
摘要
Net load forecasting (NLF) is a key component for the efficient operation and management of microgrids at high shares of renewables. Depending on the forecasting strategy followed, NLF is classified as direct or indirect. In this paper, a performance comparison was conducted between indirect and direct short-term NLF (STNLF) strategies in renewable microgrids. A STNLF model was constructed by utilizing Bayesian neural network (BNN) principles applied to datasets obtained from the University of Cyprus microgrid and buildings. For the indirect STNLF, historical load and photovoltaic (PV) generation data, along with weather and categorical time-related data were used as inputs to develop the optimized BNN models for load and PV generation forecasting. The direct STNLF model achieved lower error (3.98% at the microgrid level) compared to the indirect one.
更多查看译文
关键词
machine learning,microgrid,net load forecasting,photovoltaic
AI 理解论文
溯源树
样例
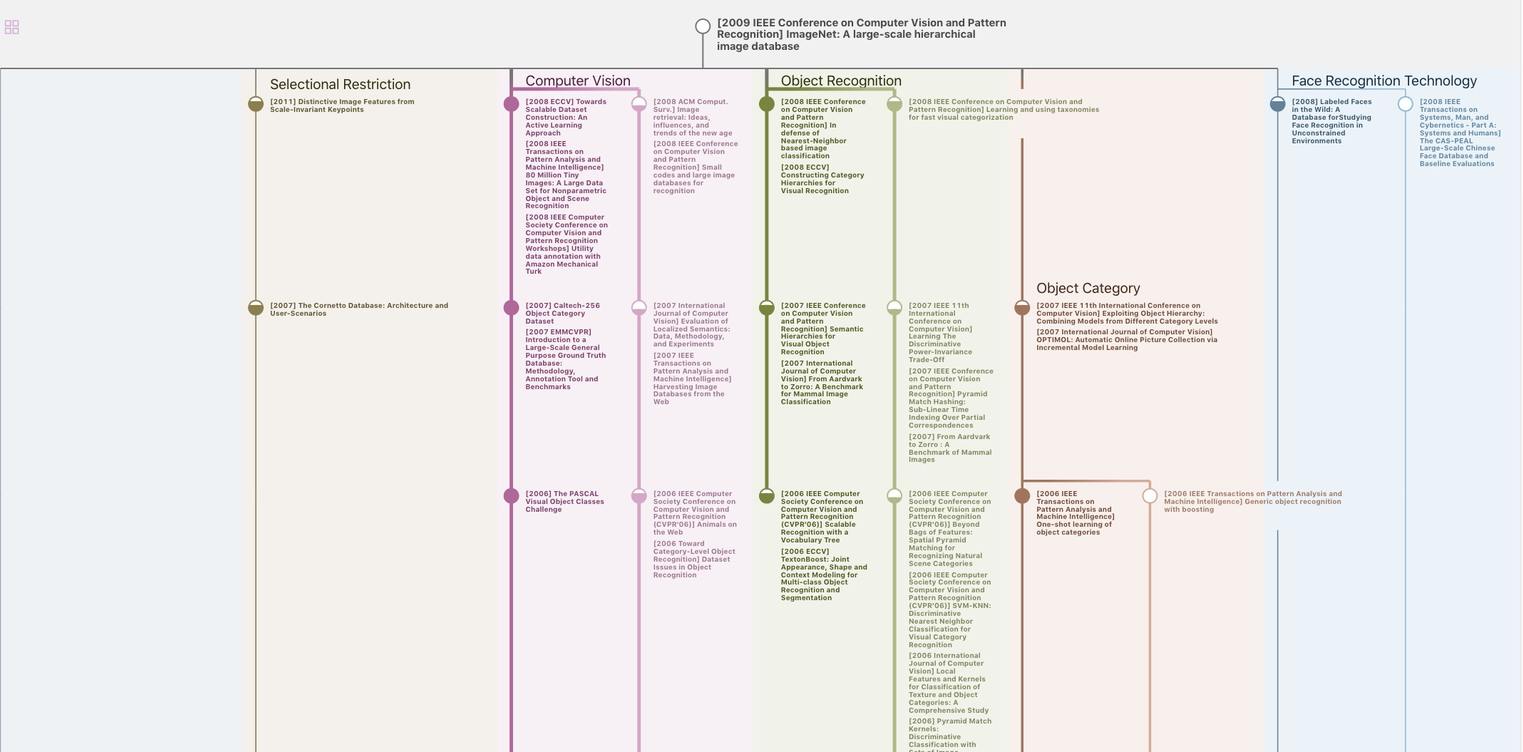
生成溯源树,研究论文发展脉络
Chat Paper
正在生成论文摘要