Federated Learning Optimization for Energy Communities in Smart Cities
2023 IEEE International Smart Cities Conference (ISC2)(2023)
摘要
The enhancement of the parameterization of a Federated Learning (FL) framework for energy communities in Smart Cities (SCs) is crucial to its effectiveness. FL is a framework that allows community members to collaborate to develop better prediction models without the need to share personal critical data information and, therefore, ensure data privacy. In the context of SCs, energy communities are more susceptible to exposing one of the disadvantages of FL: local prediction models lose the capacity of adaptation for each member. This happens because the hyperparameters of such models are equal for all. Since the net demand profiles are strongly influenced by the loads in each building, each prediction model should be parameterized building by building. This work proposes a methodology with two stages using a metaheuristic approach adapted to the context of energy communities in SCs to enhance the FL prediction system. The first stage is the computation of the local hyperparameters, over the local prediction models. Then, the second stage ensures a trade-off between the adaptivity and local accuracy of the prediction models. This methodology was tested using a FL framework customized to the main features of energy communities. A community of 200 buildings, with an integrated system of local renewable generation, was used to test the proposed approach, showing an improvement in the reliability of the day-ahead prediction of the net demand.
更多查看译文
关键词
Federated Learning,Transactive Energy Communities,Smart Cities,Smart Energy Systems,Particle Swarm Optimization
AI 理解论文
溯源树
样例
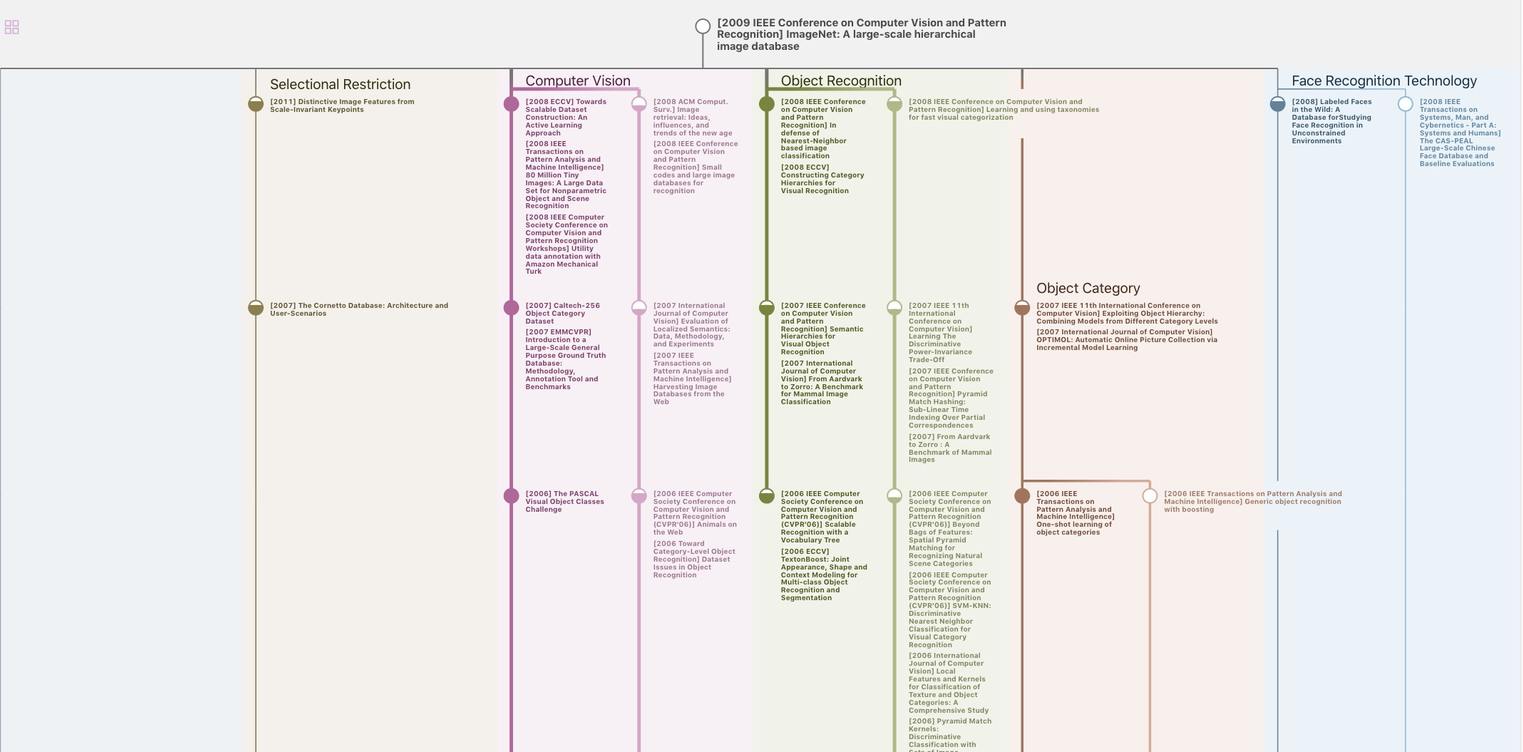
生成溯源树,研究论文发展脉络
Chat Paper
正在生成论文摘要