Machine Failure Prediction: : A Comparative Anomaly Detection
2023 Innovations in Intelligent Systems and Applications Conference (ASYU)(2023)
摘要
Anomaly detection techniques seek to uncover unusual changes in the expected behavior of target indicators and, when used for intrusion detection, suspect assaults whenever the mentioned deviations are found. This technique is crucial in identifying and flagging abnormal instances in various domains. Several anomaly detection algorithms have been suggested, tested experimentally, and assessed in qualitative and quantitative surveys in the literature. However, there is a scarcity of comparative research, and methodological shortcomings are observed in existing studies. This paper investigates the performance of ten popular anomaly detection models for feature correlation analysis for predictive maintenance to detect machine failure with the most known approaches. The models considered are Local Outlier Factor (LOF), K-Nearest Neighbors (KNN), Support Vector Machines, Elliptic Envelope, Isolation Forest, Decision Tree, Extra Trees, Random Forest, AdaBoost, and Gradient Boosting. We evaluate the models using two scenarios: one with two correlated features and another with all features focused on correlated features. The evaluation metrics used for comparison are assessed by GridSearchCV and RandomizedSearchCV and compared to the cross-validation methods.
更多查看译文
关键词
Anomaly Detection,Machine Failure Prediction,Ensemble Models,Data Scaling,Cross-Validation,Hyperparameter Tuning
AI 理解论文
溯源树
样例
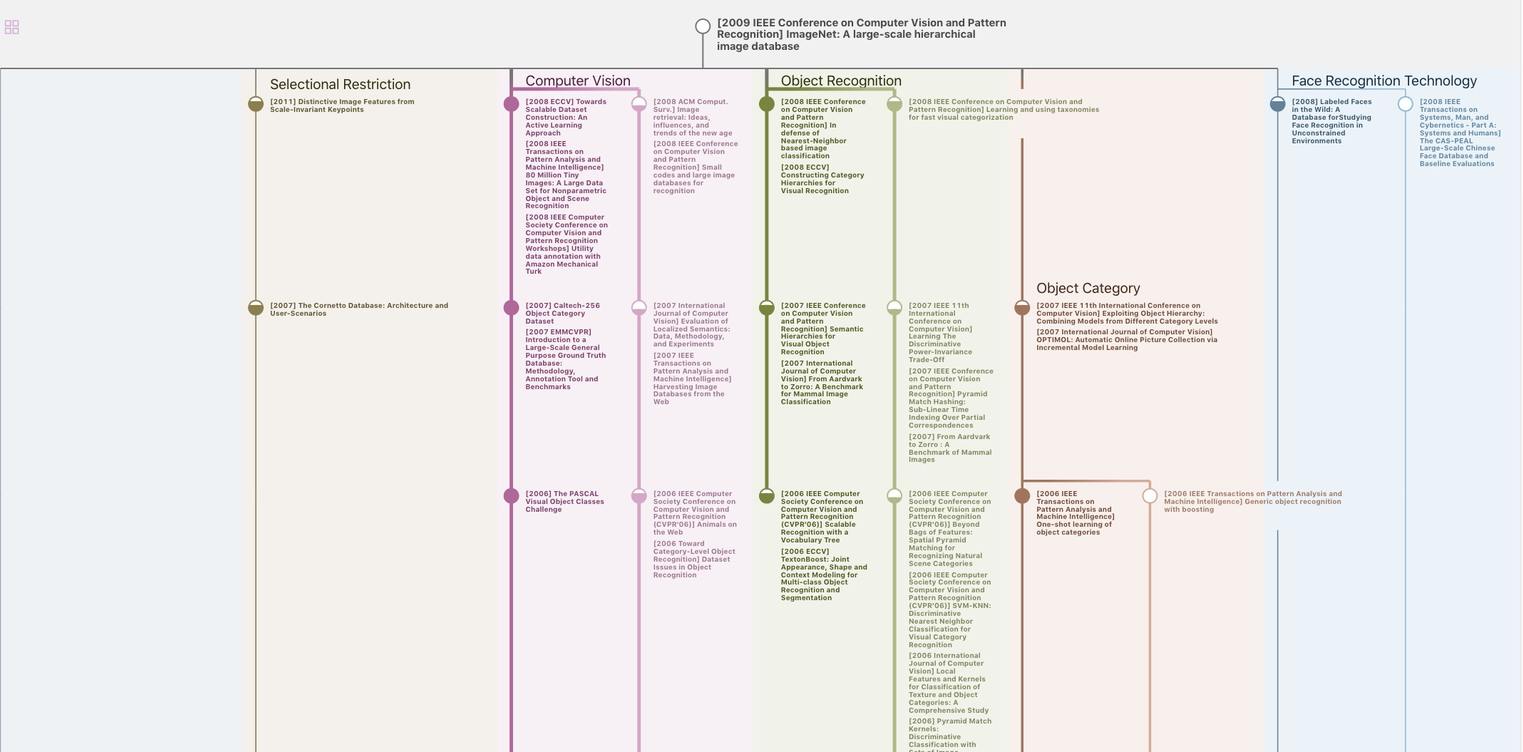
生成溯源树,研究论文发展脉络
Chat Paper
正在生成论文摘要