Performance of Hyperparameters in Prediction with Deep Neural Networks of Electrical Properties of Nano-Coated Glasses
2023 Innovations in Intelligent Systems and Applications Conference (ASYU)(2023)
摘要
Nano-coated glasses are widely used in different fields such as industry, transportation and architectural structures. After these glasses are obtained by various thin film coating applications, they are subjected to the heat treatment process (tempering, bending, etc.), which is considered as a secondary process. Both of these two-stage processes lead to changes in the electrical and mechanical properties of the final product. While electrical properties such as transmittance, coated surface reflection and uncoated surface reflection values obtained as a result of the first stage can be calculated by analytical methods; the chaotic nature of the second stage does not allow these parameters to be calculated with similar methods. Therefore, in this study, a multi-input-multi-output deep neural network structure was designed for the estimation of the electrical properties of the nano-coated glass type produced for commercial use in architectural fields. Moreover, The dataset with 64 different coating types was augmented by adding noise technique and the performances of the hyperparameters in prediction success were compared. The performance of the network structure was measured by the mean absolute error, mean squared error, and coefficient of determination metrics. The designed network structure was tested on 16 samples and according to the results obtained, it was observed that the best performance was achieved with the Adadelta learning algorithm and ReLU activation function on the augmented data set.
更多查看译文
关键词
thin film coating,deep neural networks,machine learning
AI 理解论文
溯源树
样例
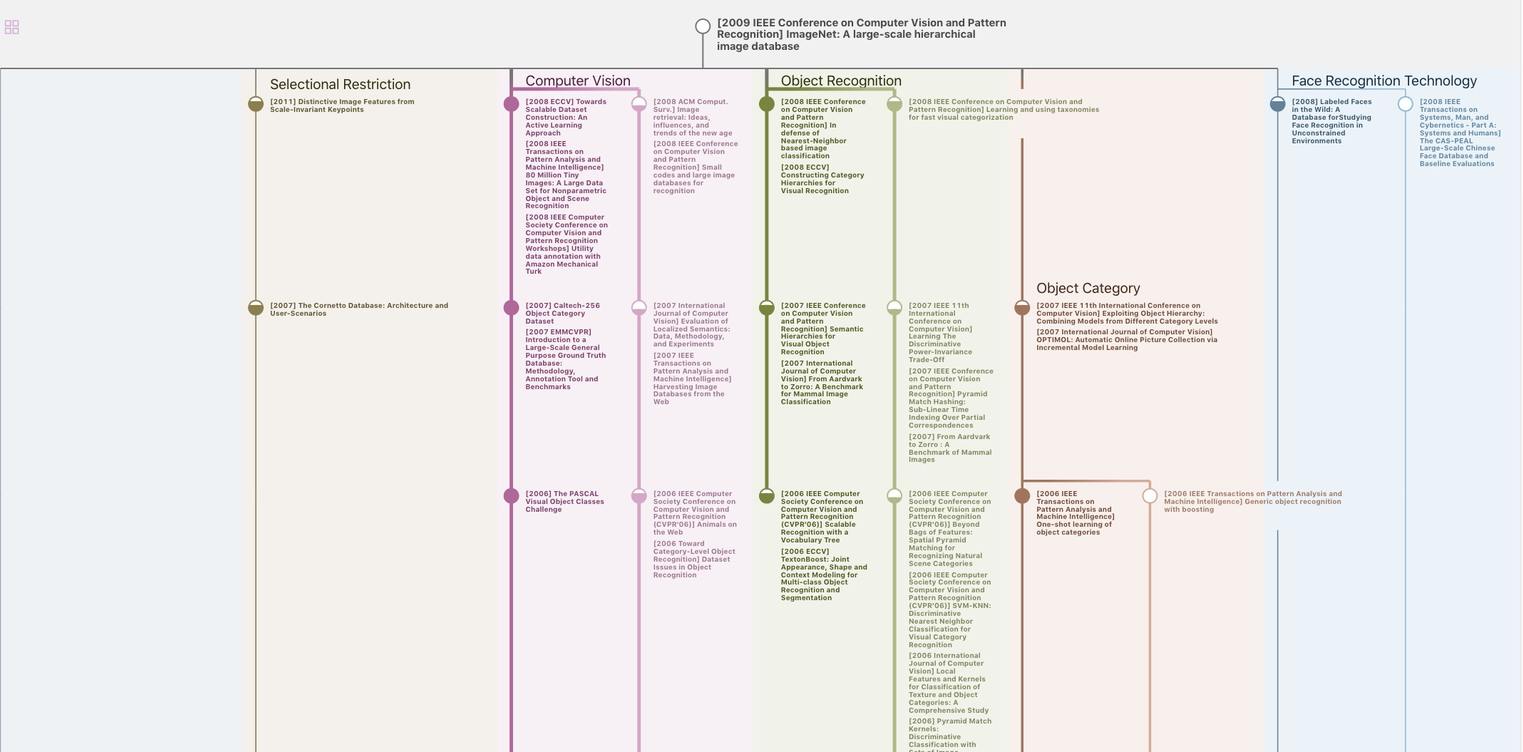
生成溯源树,研究论文发展脉络
Chat Paper
正在生成论文摘要