Measurement of adverse cosmesis in breast cancer: A deep learning approach
EXPERT SYSTEMS WITH APPLICATIONS(2024)
摘要
Background and Purpose: Adverse cosmesis or poor aesthetic appearance of the breast after breast conservation surgery (BCS) and/or radiation therapy (RT) is highly correlated with quality-of-life (QOL) in breast cancer patients. Existing methods for objective assessment of cosmesis utilize human-designed features that are calculated using outlines and/or key-points from breasts. These are complicated and time consuming to obtain. Our purpose is to develop and validate a deep neural network (DNN)-based approach for classifying adverse cosmesis from frontal digital images of patients who have undergone BCS and RT using an approximate region-of-interest (ROI) containing the breast.Materials and Methods: We used 6 datasets (5 from BCS/RT-related clinical trials) to conduct our study. Training subsets (80% of patients) from 3 datasets (RAPID, OPAR, and PORTO) were used to train 3 independent support vector classifiers for classifying adverse cosmesis using the features extracted from preprocessed, approximate breast ROIs passed through a pretrained DNN, called ResNet-18. The trained classifiers/models were evaluated through 5 experiments: holdout validation, treatment-arm specific validation in RAPID, external validation (included datasets from the remaining 3 trials), assessment of the effect of preprocessing, and comparison with BCCT.core software. Area-under-the receiver operating characteristics (AUC) was used as the primary evaluation measure. Treatment-arm specific probability values were compared by the Mann-Whitney U test.Results: AUC (0.78, 0.88, 0.94) values increased from RAPID to OPAR and PORTO respectively for holdout validation. The median probability of adverse cosmesis was higher in the same treatment arm in accordance with the interim results of the RAPID trial and differed significantly between the treatment arms (p = 0.006). For external validation, best AUC values were obtained by the model trained in RAPID (AUCs > 0.78). Preprocessing improved the performance to a large extent (average AUC: 0.87 vs 0.79 for internal validation, 0.78 vs 0.64 for external validation). AUCs of the individual models using DNN-based features were higher in 7 of 9 validation tests and statistically similar to the human-designed, dimensionless features extracted from BCCT.core software.Conclusion: Appropriately preprocessed, approximate breast ROIs can be represented by DNN-based features to identify adverse cosmesis from frontal images of breast cancer patients treated with BCS/RT. Adaptations to this approach may be necessary for its applicability to images with non-frontal views and different quality. This work serves as a foundation for developing robust DNN-based platforms for evaluating cosmesis in clinical trials.
更多查看译文
关键词
Adverse cosmesis,Quality-of-life,Image processing,Deep neural networks,External validation
AI 理解论文
溯源树
样例
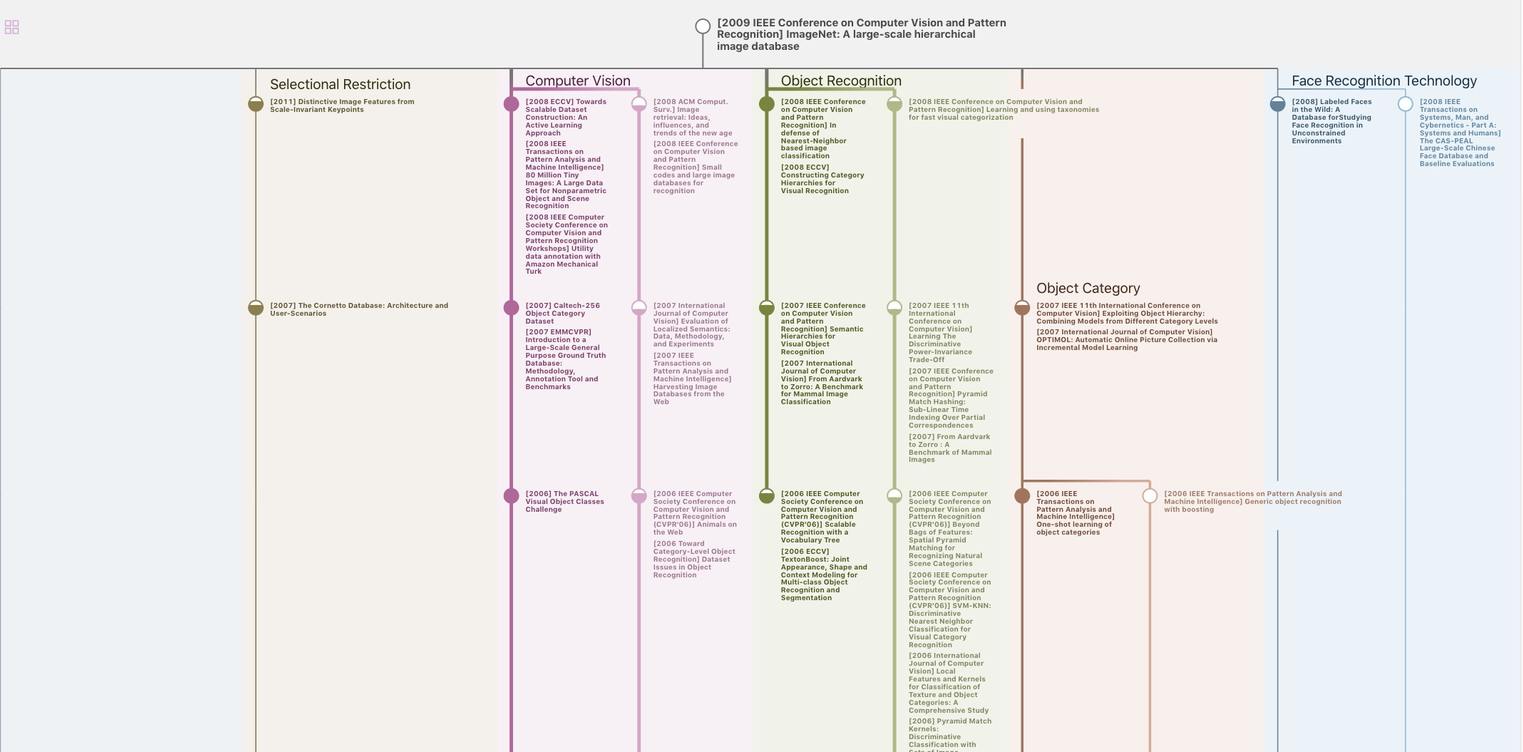
生成溯源树,研究论文发展脉络
Chat Paper
正在生成论文摘要