Low-dose CT image quality evaluation method based on radiomics and deep residual network with attention mechanism
EXPERT SYSTEMS WITH APPLICATIONS(2024)
摘要
Low-dose computed tomography (CT) produces less radiation but may degrade image quality. Research on automatic evaluation and classification of low-dose CT image quality is necessary, which is helpful to clinicians. A low-dose CT image quality evaluation method is proposed based on radiomics and deep learning. After pre-training on the ImageNet dataset, the trained deep learning model is then transferred to the target dataset of low-dose CT image. Region of interest is obtained through a deep residual network with attention mechanism. Pyradiomics is used to extract radiomics features of ROI, and T-test and LASSO algorithm are used for feature screening. Finally, support vector machine, random forest and logistic regression models are adopted to classify and predict the quality of low-dose CT image based on the selected radiomics features. Experimental results show that the trained deep residual network model with attention module can achieve 92% recognition accuracy of region of interest, and the evaluation accuracy of the three machine learning models achieve 86.6% (support vector machine), 84.5% (random forest) and 61.7% (logistic regression), respectively. Transfer learning can effectively reduce the required number of samples for the training of deep learning model and avoid overfitting. After the introduction of attention module in deep residual network model, accuracy rises faster, and the final accuracy is slightly higher than that of deep residual network model without attention module, but the improvement is slightly lower than expected. Radiomics features of region of interest reflect the quality differences of low-dose CT images which can be employed for the quality evaluation of low-dose CT image.
更多查看译文
关键词
Low-dose CT,Radiomics,Deep learning,Attention mechanism,Machine learning
AI 理解论文
溯源树
样例
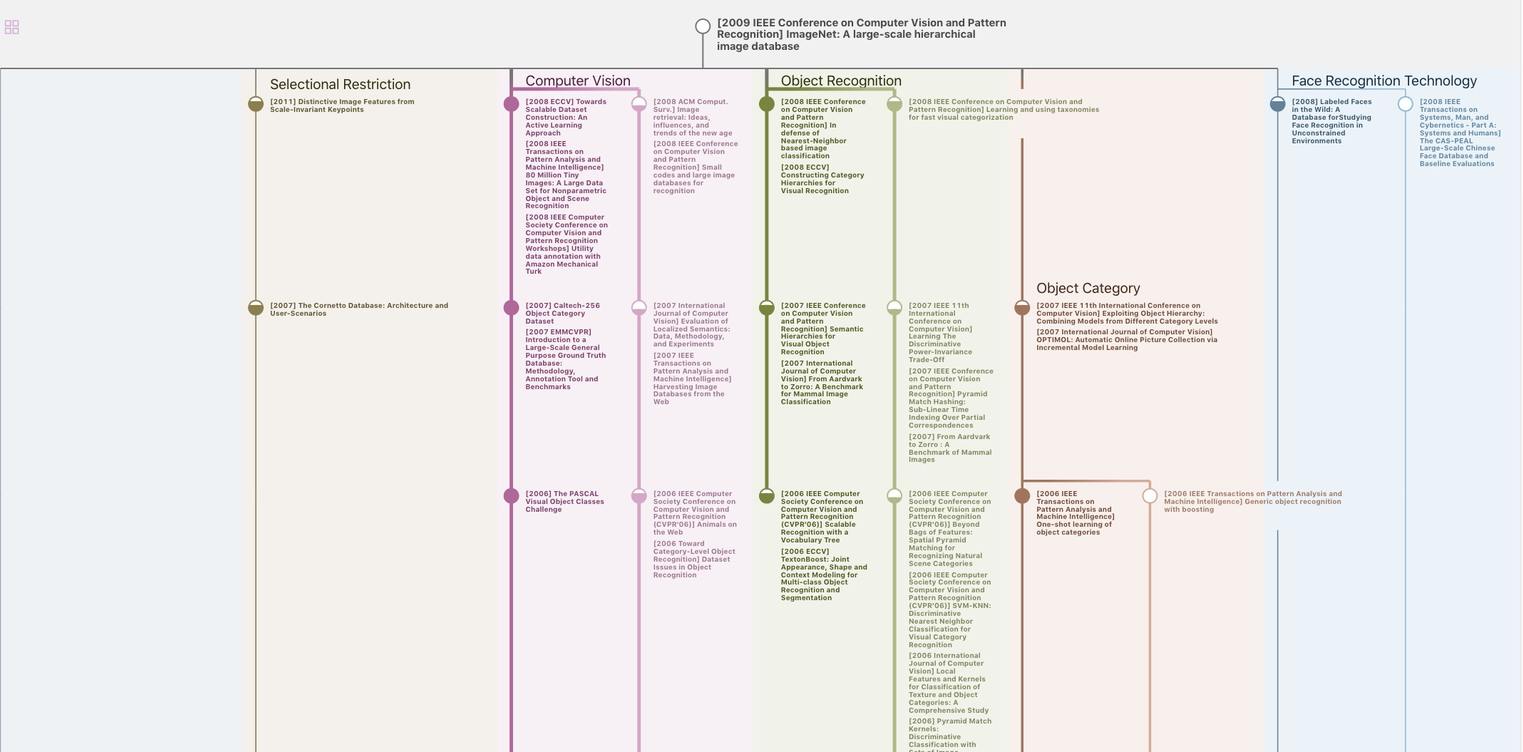
生成溯源树,研究论文发展脉络
Chat Paper
正在生成论文摘要