Role-oriented representation learning via fusioning local and higher-order feature
KNOWLEDGE-BASED SYSTEMS(2023)
摘要
Role-oriented representation learning extracts structural features by capturing the structural similarities of nodes to generate representations that preserve the structural equivalence, which is crucial for addressing the role discovery task of real networks. However, most studies directly combine different types of structural features and ignore the correlations among features, which is not enough to accurately portray node roles. Therefore, we propose a novel role-oriented network representation learning method RFLH that mines the correlations among features by normalizing flows to improve the performance on the role discovery tasks. We utilize the Variational Auto-Encoder (VAE) to generate the original representation distribution and learn the structural features of nodes by constraint of local and higher-order features, where local features are learned by the traditional variational module, while higher-order features are learned by the flow-based variational module that introduces normalizing flows to map the original representation distribution to a complex distribution. Moreover, we deduce the new loss by introducing the Jacobian determinant to Evidence Lower Bound (ELBO). Finally, we fuse local and higher-order features into the node representations, which are concatenated by the representations sampled from the original distribution and the new distribution, to generate the final node representations. Extensive experimental results on several real network datasets demonstrate that our model achieves superior performance compared to typical baseline methods.
更多查看译文
关键词
Representation learning,Role discovery,Local and higher-order features,Normalizing flows
AI 理解论文
溯源树
样例
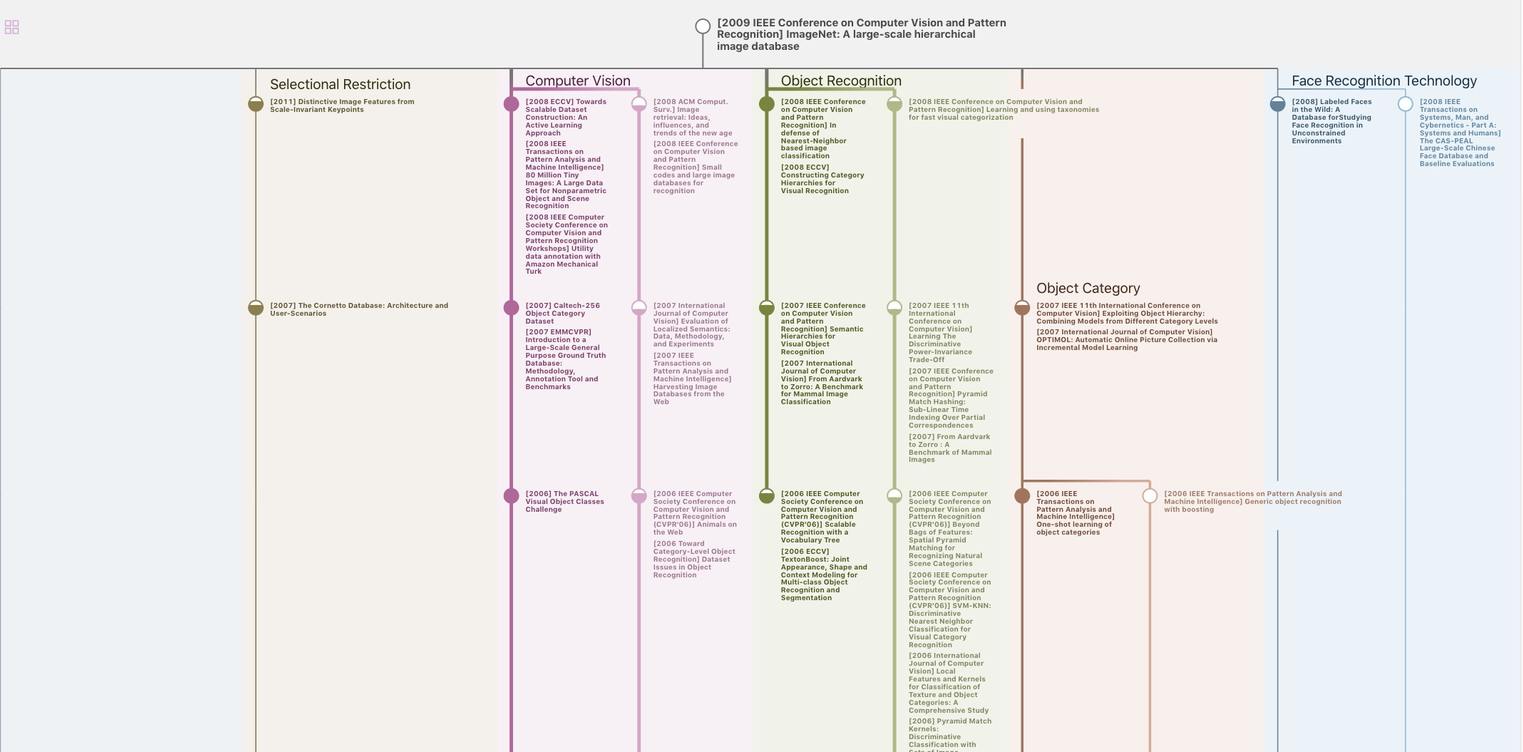
生成溯源树,研究论文发展脉络
Chat Paper
正在生成论文摘要