Probabilistic runoff forecasting considering stepwise decomposition framework and external factor integration structure
EXPERT SYSTEMS WITH APPLICATIONS(2024)
摘要
As changes in external weather introduce significant uncertainty into runoff prediction, probabilistic runoff forecasting becomes increasingly important for water resource managers. However, limited research has been conducted on the rational employment of decomposition techniques in probabilistic prediction, especially when considering external factors effectively. To fill the gap, this paper proposes an innovative framework for probabilistic runoff forecasting. It first utilizes the stepwise decomposition (SD) technique to obtain training and test samples. Then an integration structure considering external factors is designed to enhance the learning ability of the neural network for various features and decomposition sequences. The boundary correction process utilizes the dynamic time warping (DTW) method to optimize the boundaries of the obtained samples in SD. This comprehensive framework is named SDIFC. Building upon this framework, we achieve a synergistic integration of three distinct methods: seasonal-trend decomposition procedures based on loess (STL), least absolute shrinkage and selection operator (LASSO), and quantile regression long short-term memory (QRLSTM), named STL-LASSO-QRLSTM. Multiple experiments and evaluations demonstrate that the three methods are very well adapted to the SDIFC framework. The proposed forecasting method not only captures the runoff trend accurately but also quantifies the uncertainty in the prediction process. The proposed method exhibits significant advantages in probabilistic forecasting, achieving an average improvement of 28.1%, 35.5%, and 27.3% in continuous rank probability score (CRPS) when compared to the model without SDIFC, the model using SD in other frameworks, and the model without LASSO, respectively.
更多查看译文
关键词
Runoff forecasting,Probabilistic forecasting,Stepwise decomposition,Boundary correction,External factor integration structure
AI 理解论文
溯源树
样例
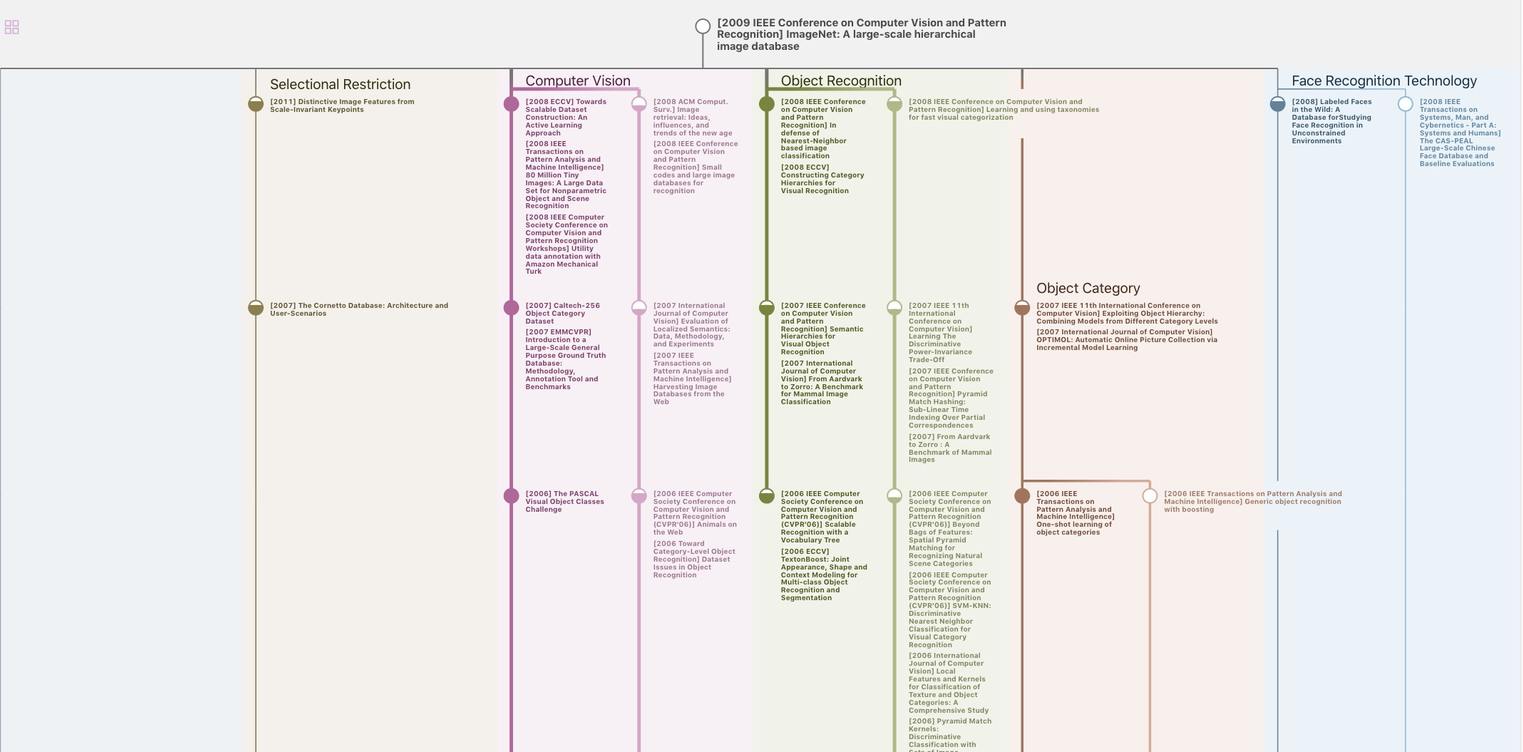
生成溯源树,研究论文发展脉络
Chat Paper
正在生成论文摘要