Continuous Training and Fine-tuning for Domain-Specific Language Models in Medical Question Answering.
CoRR(2023)
摘要
Large language models exhibit promising general capabilities but often lack specialized knowledge for domain-specific tasks. Developing domain experts from a base model enables a range of applications without prohibitive training costs. This work demonstrates a method using continuous training and instruction fine-tuning to rapidly adapt Llama 2 base models to the Chinese medical domain. We first conduct continuous training on 1B tokens from Chinese medical references to teach relevant vocabulary and knowledge. The models are then fine-tuned on 54K examples sourced from the Chinese National Medical Licensing Examination. Experiments on Chinese medical data confirm the effectiveness of this approach, producing a model comparable to GPT-3.5-turbo while using way less computational resource. The resulting domain-specific model could be useful for various Chinese medical applications. More broadly, this provides a template for domain-specific training of large language models in areas where pre-trained models lack the required expertise, such as law, science, and engineering.
更多查看译文
关键词
medical question answering,language models
AI 理解论文
溯源树
样例
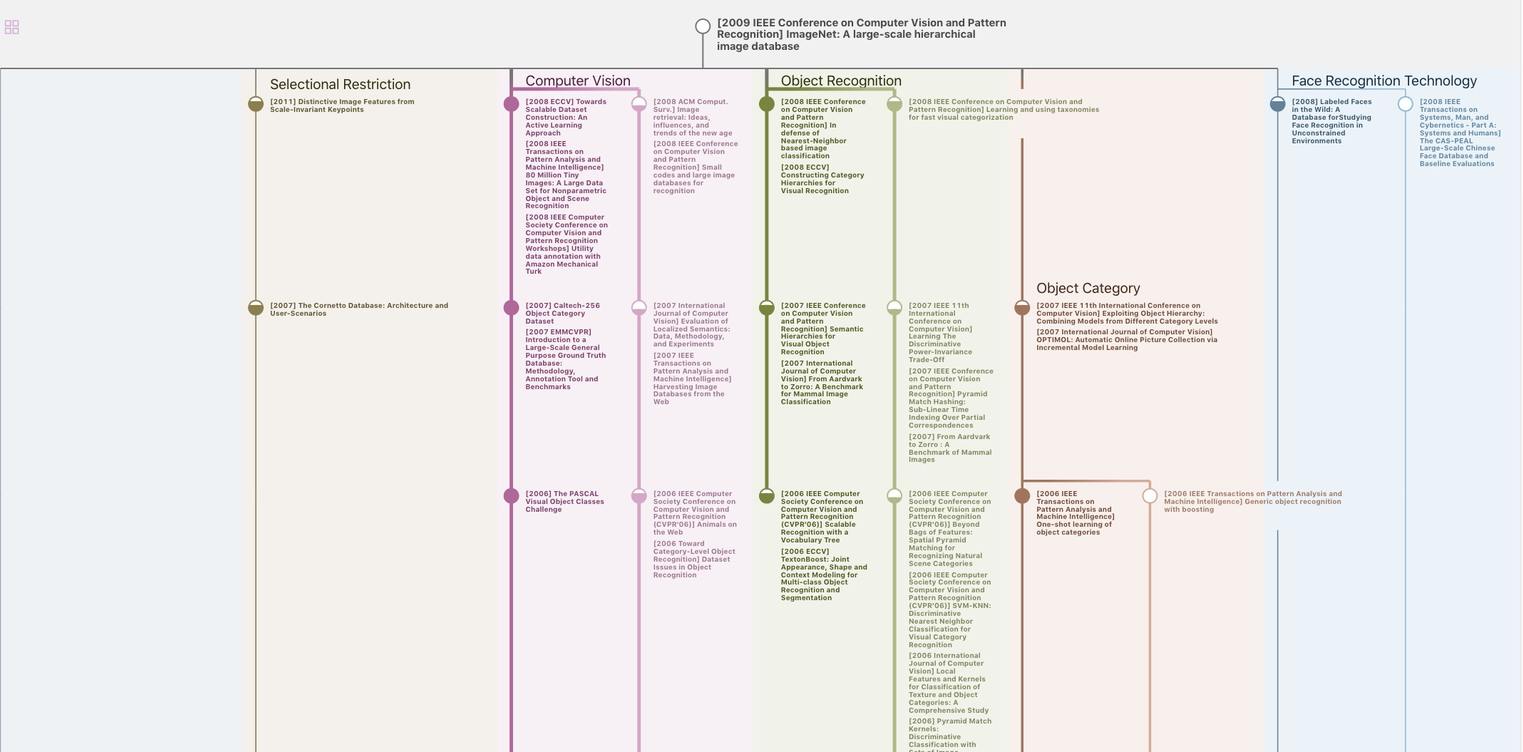
生成溯源树,研究论文发展脉络
Chat Paper
正在生成论文摘要