Localized keyhole pore prediction during laser powder bed fusion via multimodal process monitoring and X-ray radiography
ADDITIVE MANUFACTURING(2023)
摘要
Systematic fault detection and control during laser powder bed fusion (L-PBF) has been a long-standing objective for system manufacturers and researchers in the additive manufacturing (AM) industry. This manuscript investigates a data fusion approach for detection of keyhole porosity formation during laser irradiation of Ti-6Al-4V substrates by concurrent recording of thermally induced optical emission measured using both off-axis and coaxial photodiode sensors, and acoustic emission. Subsurface defect formation was monitored via high-speed synchrotron X-ray imaging at 20,000 frames per second, enabling temporal registration of keyhole pore formation events to the monitoring signals at a resolution of 50 mu s. We developed data fusion machine learning (ML) models for localized prediction of keyhole pore formation at various time scales ranging from 0.5 ms to 2 ms. The signal segments were featurized using two independent approaches: (1) power spectral density (PSD) and (2) highly comparative time series analysis (HCTSA) framework. The extracted features from different sensor mo-dalities were fused together to construct a multimodal feature space and sequential feature selection was used to determine the most informative features for training the ML models. The predictive performance was evaluated for three classifying algorithms: Support Vector Machine (SVM), K-Nearest Neighbor (KNN), and Gaussian Naive Bayes (GNB). As a result, pore formation events were predicted with up to 0.95 F1-score, 1.0 recall and 0.94 accuracy. The most heavily weighted features indicate that model performance is chiefly governed by the acoustic monitoring signal, with a secondary contribution from the optical emission sensors.
更多查看译文
关键词
Laser powder bed fusion,In situ monitoring,X-ray radiography,Keyhole pore identification,Sensor fusion
AI 理解论文
溯源树
样例
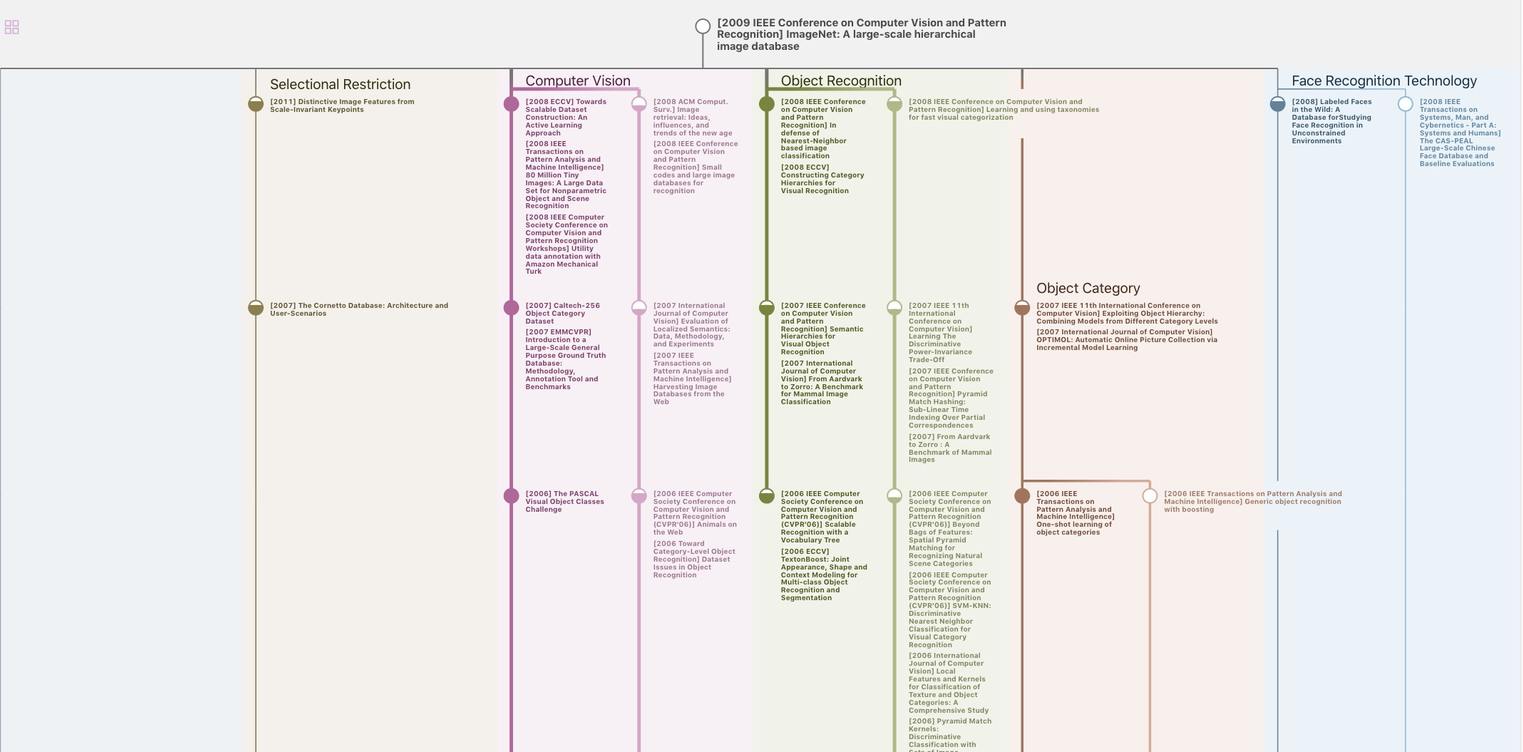
生成溯源树,研究论文发展脉络
Chat Paper
正在生成论文摘要