Multi-label Ophthalmological Disease Classification Using Vision Transformers
2023 5th Novel Intelligent and Leading Emerging Sciences Conference (NILES)(2023)
摘要
Immediate diagnosis and timely treatment of fundus diseases are crucial in preventing permanent vision loss. Fundus imaging, a primary method in retinal imaging, effectively captures and investigates anatomical features and abnormalities in the human eye, and aids in observing and identifying various ophthalmological diseases. These diseases are indicated by variations in or surrounding structures such as the optic disk and the blood vessels. Given the complex nature of these diseases, it’s common for fundus images to show a patient having multiple conditions in one or both eyes. This work aims to develop and suggest various methods for detecting and classifying ophthalmological diseases using multiple deep learning vision transformers. The experiments were conducted and models tested using the ODIR-2019 dataset, which contains fundus images of both left and right eyes. The dataset includes eight categories, and the goal was to implement a transformer based approach for multi-label classification. The study used pre-trained Swin Transformer V2 and Data Efficient Image Transformer (DeiT) in its proposed approaches. After various preprocessing and fine-tuning techniques, the results showed that the transformer approach outperformed state-of-the-art CNN models in terms of accuracy. It achieved an accuracy of 93.1% and AUC of 94.4% for multi-label ophthalmological disease classification.
更多查看译文
关键词
Medical Imaging Processing and Analysis,Multi-label classification,ODIR-2019,Disease classification,Deep learning,Transformers
AI 理解论文
溯源树
样例
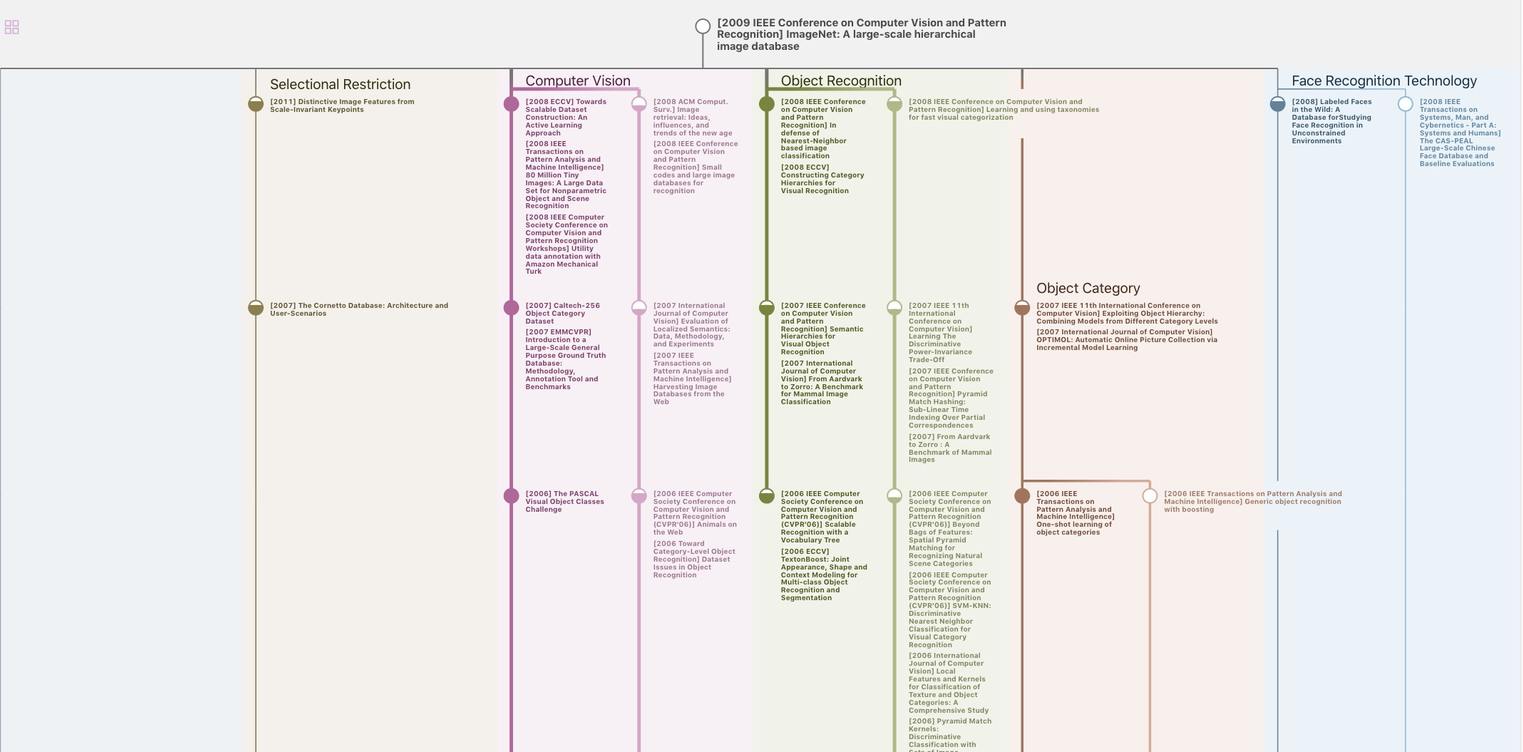
生成溯源树,研究论文发展脉络
Chat Paper
正在生成论文摘要