Near-end Intelligibility Improvement Through Voice Transformation in Transfer Learning Framework
2023 31st European Signal Processing Conference (EUSIPCO)(2023)
摘要
In recent works, using voice transformation functions (VTF) in optimal shifting of formants has improved near-end speech intelligibility. Though these VTFs are promising, they are computationally expensive to optimize and generate unwanted artifacts during voice modification. Additionally, they were specific to the environmental condition they were optimized for. For the applicability of this approach to different languages without re-optimization, transfer learning (TL) was used to shape the parameters of VTF to accommodate the target language [1]. However, TL across noises and TL across languages and noises (simultaneously) was not viable due to the dependency on pitch information of source and target noises. Hence in this work, a statistical Gaussian Transformation Function (GTF) is developed with parameters optimized for specific environmental conditions. Defined by just three parameters, the optimization time came down, and the intelligibility surpassed the previously used VTF. Additionally, GTF allows TL across both noises and languages simultaneously, with fewer artifacts while shifting the formants.
更多查看译文
关键词
Speech Intelligibility,Gaussian Transformation Function,CLPSO,STOI,Transfer Learning
AI 理解论文
溯源树
样例
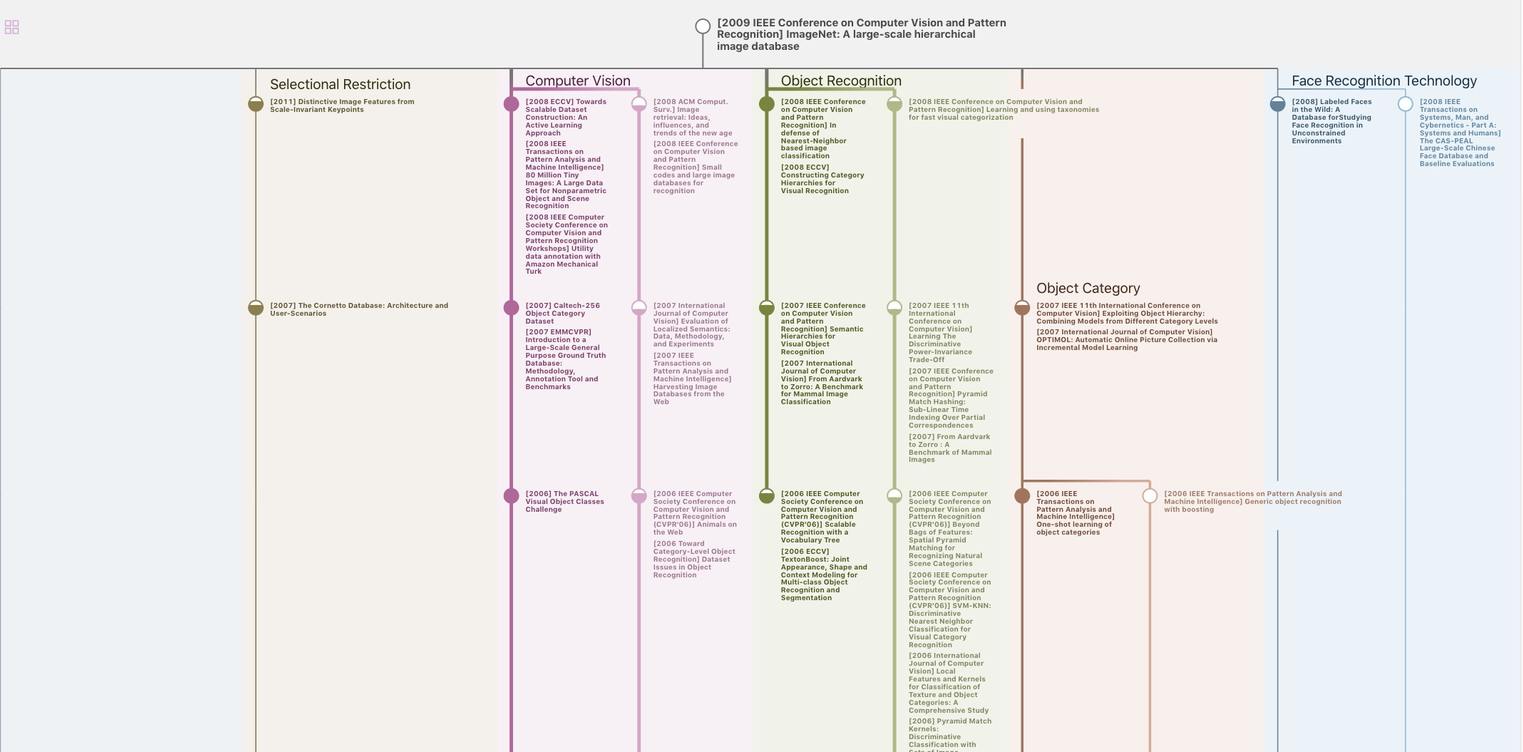
生成溯源树,研究论文发展脉络
Chat Paper
正在生成论文摘要