Named Entity Recognition for Epidemiological Investigation in COVID-19
2023 IEEE International Conference on Intelligence and Security Informatics (ISI)(2023)
摘要
The COVID-19 pandemic has had a global impact on communities, economies, and healthcare systems. To control the virus's spread, numerous epidemiological investigations have been made available online, leading to a growing demand for automated tools to extract valuable information from case reports and reduce the burden on news reporters. In response to this growing need, we have meticulously curated a comprehensive data set of COVID-19 epidemiological investigation corpora, specifically designed for named entity recognition (NER) applications. This data set enables researchers and analysts to efficiently identify and extract key information from the case reports, streamlining the process of understanding and communicating the findings. To further enhance the effectiveness of NER in the context of epidemiological investigations, we evaluated and compared the performance of three cutting-edge, pre-trained model-based methods: BERT-BiLSTM-CRF, ERNIE-BiLSTM- CRF and ALBERT-BiLSTM-CRF. All techniques demonstrated impressive performance in recognizing named entities within the case reports, showcasing their potential to revolutionize the way in which epidemiological data is analyzed and disseminated. By leveraging these advanced NER techniques, we aim to facilitate more accurate and timely reporting, ultimately contributing to better-informed decision-making processes and improved public health outcomes.
更多查看译文
关键词
Named Entity Recognition,COVID-19,ERNIE,BERT,ALBERT,Conditional Random Field
AI 理解论文
溯源树
样例
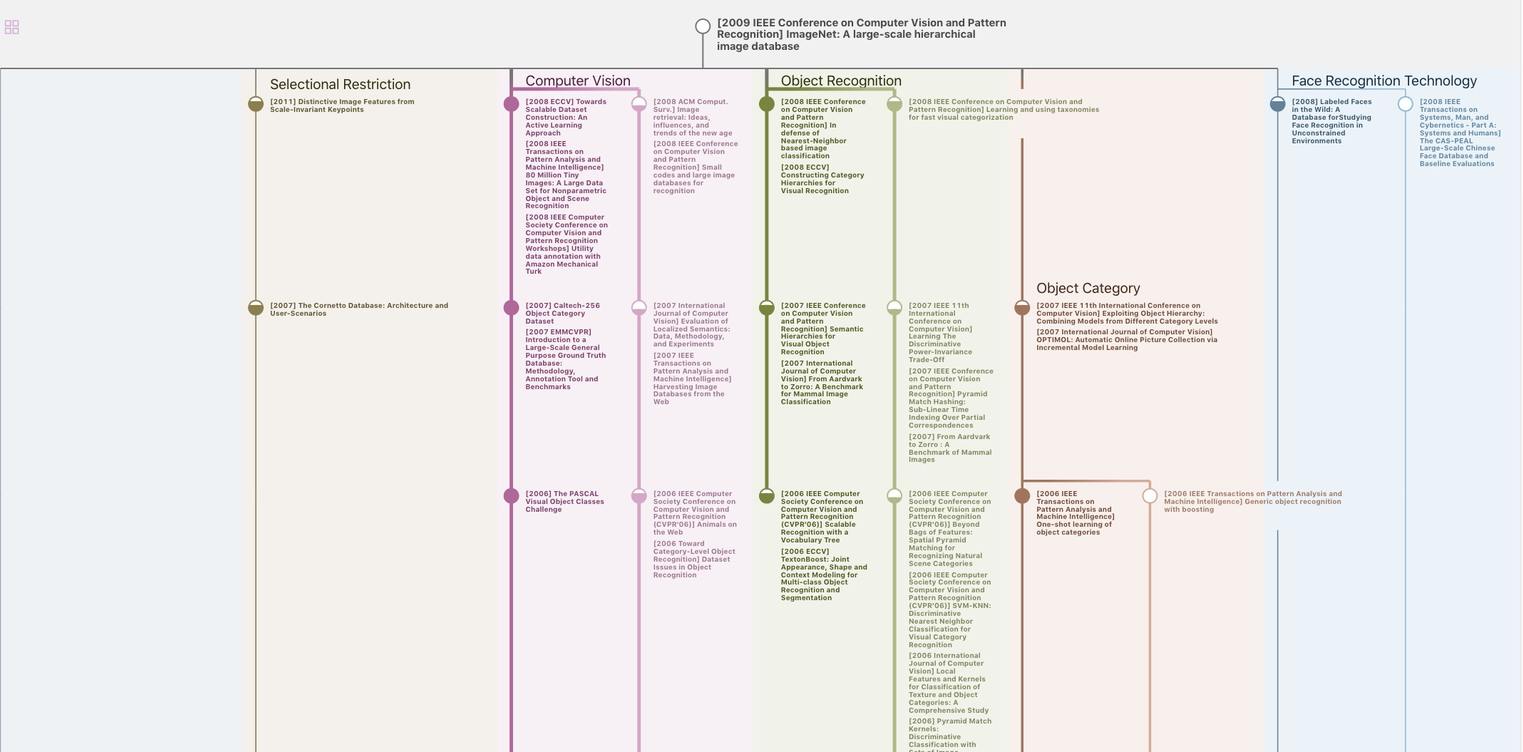
生成溯源树,研究论文发展脉络
Chat Paper
正在生成论文摘要