A new model predictive control approach integrating physical and data-driven modelling for improved energy performance of district heating substations
ENERGY AND BUILDINGS(2023)
摘要
District heating (DH) substations play a crucial role in ensuring the efficient and effective distribution of thermal energy necessary to provide space heating for buildings. However, optimizing their operation for energy savings while still ensuring indoor comfort poses significant challenges due to the complex dynamics of building demand and the inertia of building envelopes. To address these challenges, this study introduces a novel model predictive control (MPC) approach that combines a reduced-order physical model with a machine learning-based datadriven model to jointly optimize the operation parameters of a DH substation. In this approach, a reduced-order physical model is first used to capture essential operational principles and energy behaviors of the DH substations and generate candidate solutions for the control of the DH substations. Then, a data-driven model is constructed by integrating a Long Short-Term Memory model and a Back-propagation Neural Network, leveraging historical operational data of the DH substation concerned. The data-driven model is further formulated into a data-driven MPC framework to identify optimal control solutions from all candidates provided by the physical model. To evaluate the proposed approach, a data-driven surrogate model is developed using real operational data. Comparative analysis against the original fuzzy rule-based control strategy and a pure data-driven strategy demonstrates a substantial reduction in heat consumption of 4.77% and 19.47%, respectively. Moreover, compared with using a reduced-order physical model alone, this approach achieves additional benefits in reducing the energy consumption of the DH substation and minimizing indoor temperature fluctuations within the end-users.
更多查看译文
关键词
Model predictive control,District heating system,Machine learning,Physical modelling
AI 理解论文
溯源树
样例
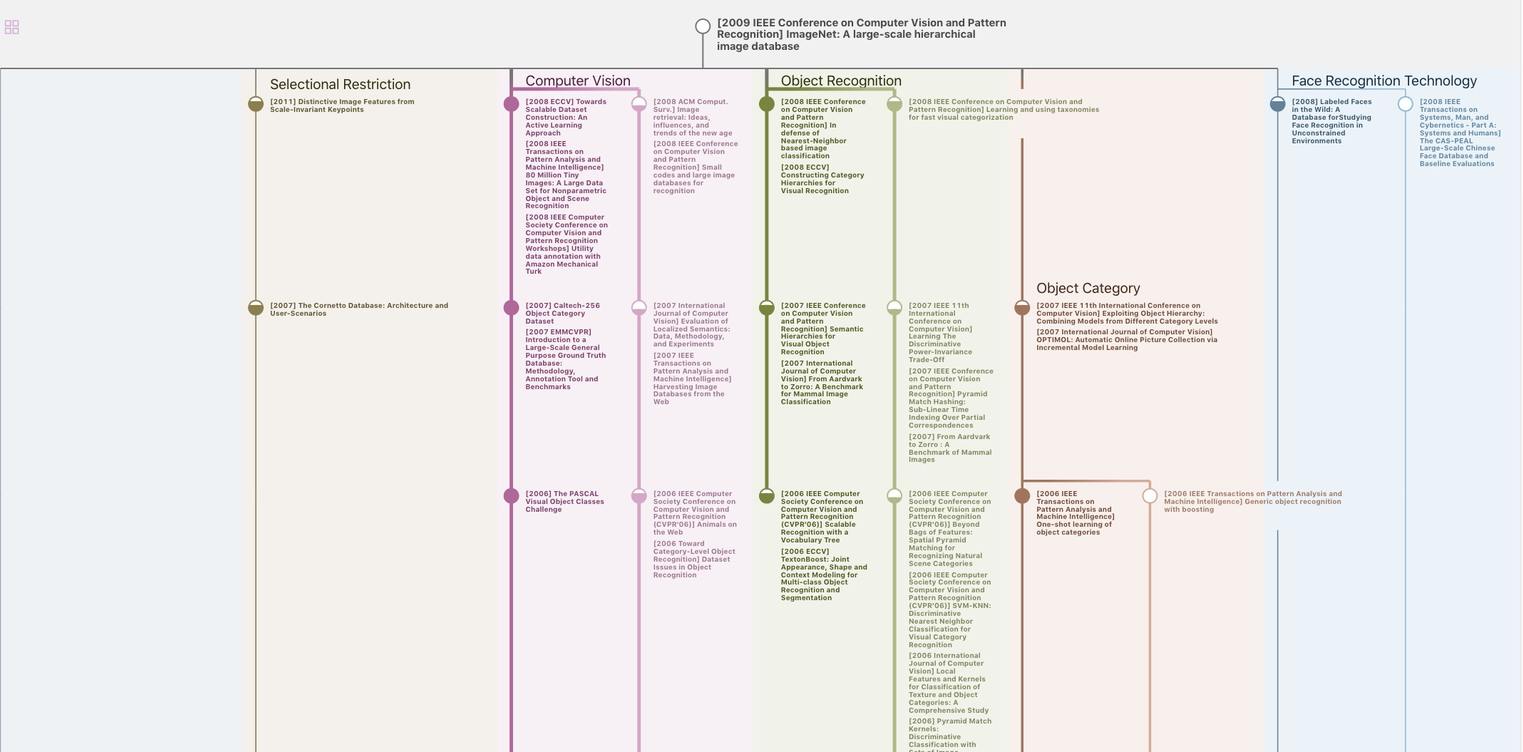
生成溯源树,研究论文发展脉络
Chat Paper
正在生成论文摘要