A comprehensive study on developing an intelligent framework for identification and quantitative evaluation of the bearing defect size
RELIABILITY ENGINEERING & SYSTEM SAFETY(2024)
摘要
An intelligent framework is necessary to detect and analyze bearing defects in rotating machinery to prevent unexpected downtime and achieve performance per Industry 4.0 standards. This study presents a framework to detect faults and precisely quantify their size. The framework triggers an AI model to identify the defect and another model for quantitative evaluation of defect size. After analyzing the various classification and regression models, it has been found that The k-nearest neighbor (KNN) algorithm is suggested as the most effective AI model for identifying bearing defects. The ensemble tree is the most effective AI model for defect quantification. The results showed that the proposed algorithm can estimate the defect width reasonably. The maximum error in estimating the inner race, outer race, and roller defect widths was 2.474%, 14.534%, and 5.517%, respectively. The AI model's capacity to identify bearing defects of different sizes, which were not included in the training dataset, was also tested. The test yielded successful results. By using this framework, industries can prevent unexpected downtime, reduce maintenance costs, and enhance the performance and reliability of rotating machinery.
更多查看译文
关键词
Intelligent framework,Quantitative evaluation,Bearing,Race defect size,Artificial intelligence,Vibration
AI 理解论文
溯源树
样例
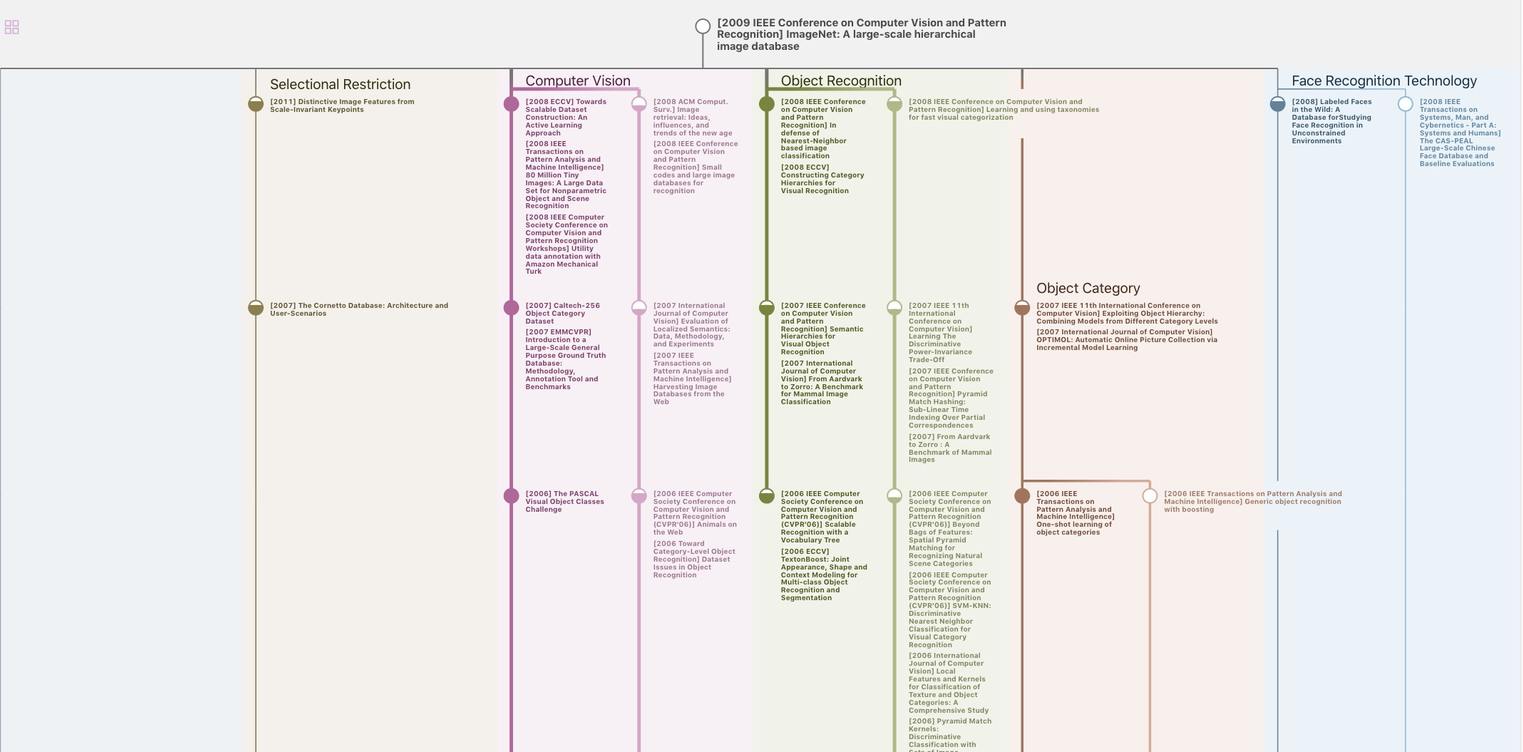
生成溯源树,研究论文发展脉络
Chat Paper
正在生成论文摘要