Assessing vulnerability of coastal aquifer to seawater intrusion using Convolutional Neural Network
MARINE POLLUTION BULLETIN(2023)
摘要
This study examined coastal aquifer vulnerability to seawater intrusion (SWI) in the Shiramin area in northwest Iran. Here, six types of hydrogeological data layers existing in the traditional GALDIT framework (TGF) were used to build one vulnerability map. Moreover, a modified traditional GALDIT framework (mod-TGF) was prepared by eliminating the data layer of aquifer type from the GALDIT model and adding the data layers of aquifer media and well density. To the best of our knowledge, there is a research gap to improve the TGF using deep learning algorithms. Therefore, this research adopted the Convolutional Neural Network (CNN) as a new deep learning algorithm to improve the mod-TGF framework for assessing the coastal aquifer vulnerability. Based on the findings, the CNN model could increase the performance of the mod-TGF by >30 %. This research can be a reference for further aquifer vulnerability studies.
更多查看译文
关键词
Coastal aquifer,Vulnerability,Convolutional Neural Network (CNN),Deep learning,GALDIT,Seawater intrusion (SWI)
AI 理解论文
溯源树
样例
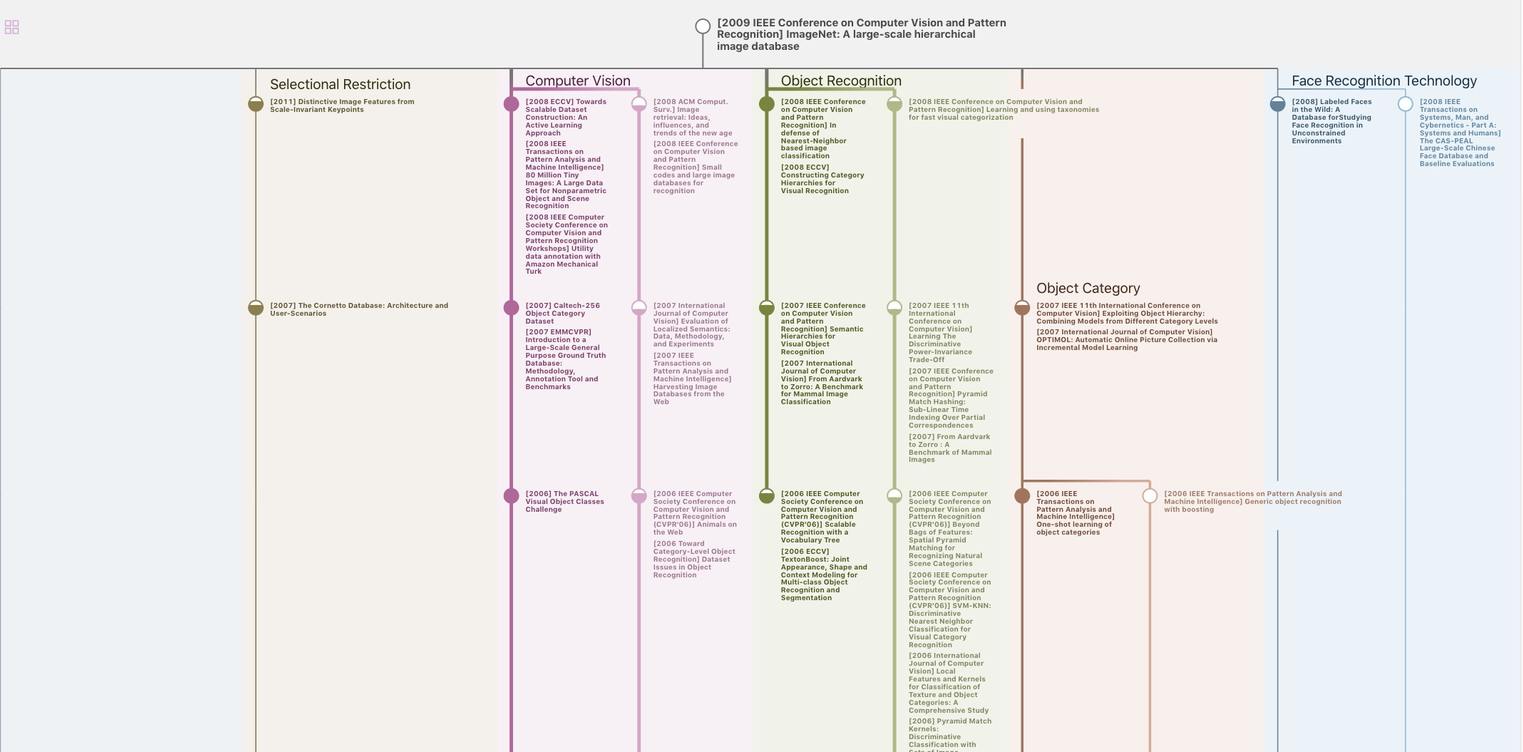
生成溯源树,研究论文发展脉络
Chat Paper
正在生成论文摘要