Learning implicit labeling-importance and label correlation for multi-label feature selection with streaming labels
PATTERN RECOGNITION(2024)
摘要
Multi-label feature selection plays an increasingly important role in alleviating the high dimensionality of multi-label learning tasks. Most extant methods posit that the learning task is performed in an environment where the label space is statically known. In reality, however, the environment is open and the labels may arrive dynamically, which is coined as streaming labels. Streaming labels-based multi-label feature selection suffers from many challenges derived from label space: (1) The label space expands dynamically; (2) Newly arrived labels exhibit complex relationships, often involving label correlation and labeling-importance. To cope with this challenge, in this paper, an intuitive yet effective algorithm named LLSL, i.e. learning implicit labeling-importance and label correlation for multi-label feature selection with streaming labels, is proposed. To be specific, the implicit labeling-importance with respect to streaming labels is firstly formalized by conducting the nearest neighbor reconstruction on feature space. Secondly, label correlation is seamlessly integrated into the objective function of feature relevance by designing the feature relevance influence factor. Based on the above, we build a feature conversion, which can realize the fusion of label-specific features for each streaming label. Finally, extensive experiments conducted on fifteen benchmark datasets provide clear evidence that LLSL has superior performance compared to three established streaming label-based MFS algorithms and seven static label space-based MFS algorithms.
更多查看译文
关键词
Multi-label feature selection,Streaming labels,Labeling-importance,Label correlation
AI 理解论文
溯源树
样例
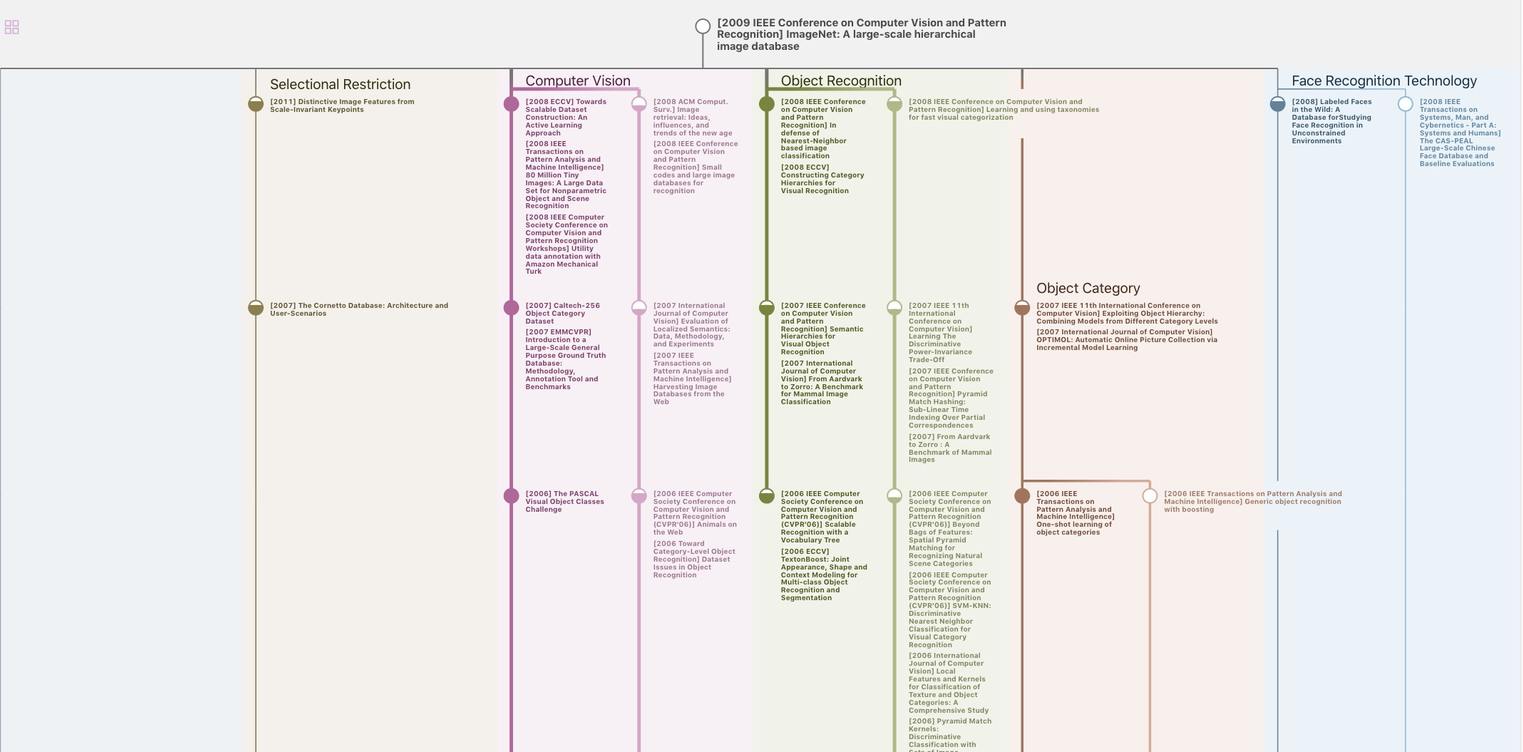
生成溯源树,研究论文发展脉络
Chat Paper
正在生成论文摘要