Latent space representations of cosmological fields
arXiv (Cornell University)(2023)
摘要
We investigate the possibility of learning the representations of
cosmological multifield dataset from the CAMELS project. We train a very deep
variational encoder on images which comprise three channels, namely gas density
(Mgas), neutral hydrogen density (HI), and magnetic field amplitudes (B). The
clustering of the images in feature space with respect to some
cosmological/astrophysical parameters (e.g. $\Omega_{\rm m}$) suggests that the
generative model has learned latent space representations of the high
dimensional inputs. We assess the quality of the latent codes by conducting a
linear test on the extracted features, and find that a single dense layer is
capable of recovering some of the parameters to a promising level of accuracy,
especially the matter density whose prediction corresponds to a coefficient of
determination $R^{2}$ = 0.93. Furthermore, results show that the generative
model is able to produce images that exhibit statistical properties which are
consistent with those of the training data, down to scales of $k\sim 4h/{\rm
Mpc}.$
更多查看译文
关键词
latent space representations
AI 理解论文
溯源树
样例
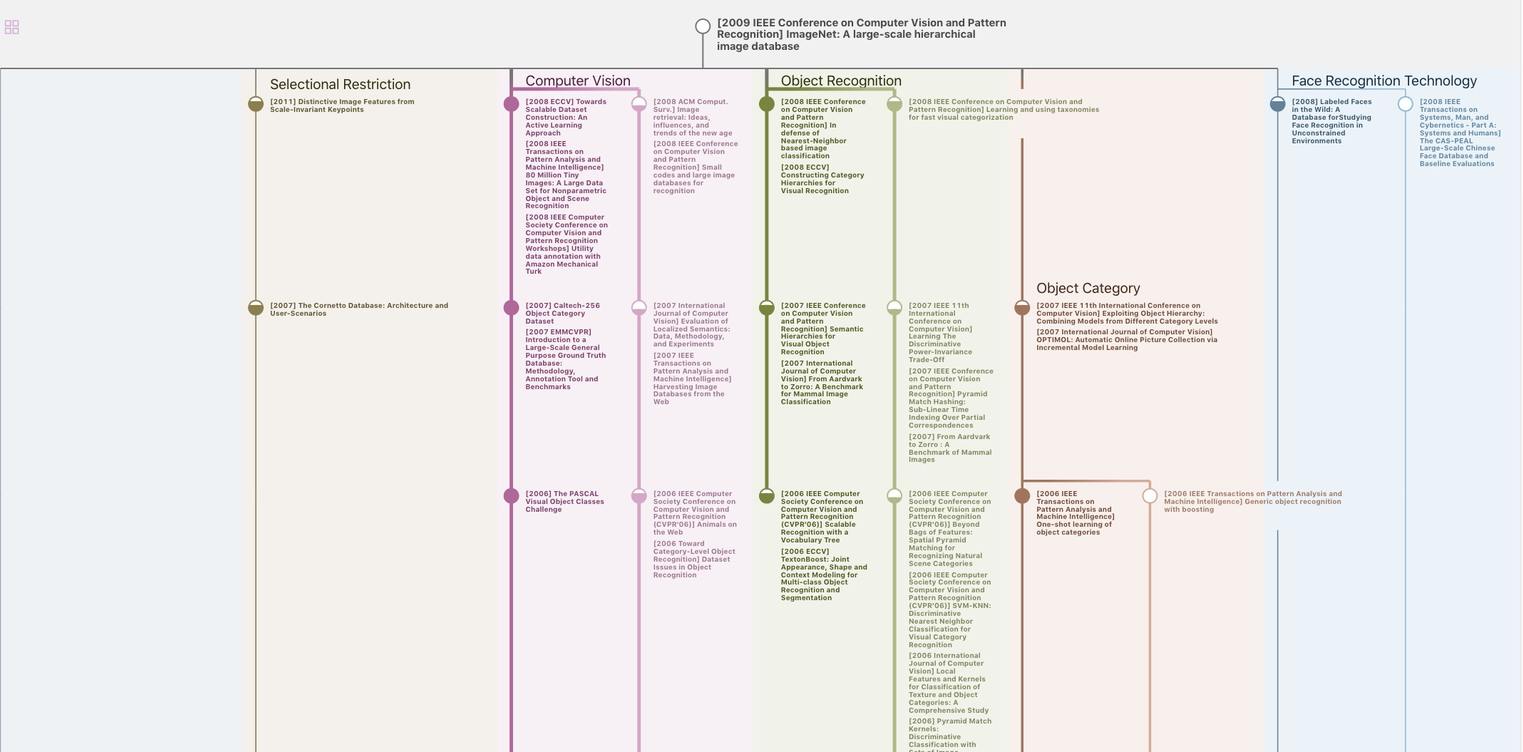
生成溯源树,研究论文发展脉络
Chat Paper
正在生成论文摘要