Bridging the Gap: Addressing Discrepancies in Diffusion Model Training for Classifier-Free Guidance.
CoRR(2023)
摘要
Diffusion models have emerged as a pivotal advancement in generative models,
setting new standards to the quality of the generated instances. In the current
paper we aim to underscore a discrepancy between conventional training methods
and the desired conditional sampling behavior of these models. While the
prevalent classifier-free guidance technique works well, it's not without
flaws. At higher values for the guidance scale parameter $w$, we often get out
of distribution samples and mode collapse, whereas at lower values for $w$ we
may not get the desired specificity. To address these challenges, we introduce
an updated loss function that better aligns training objectives with sampling
behaviors. Experimental validation with FID scores on CIFAR-10 elucidates our
method's ability to produce higher quality samples with fewer sampling
timesteps, and be more robust to the choice of guidance scale $w$. We also
experiment with fine-tuning Stable Diffusion on the proposed loss, to provide
early evidence that large diffusion models may also benefit from this refined
loss function.
更多查看译文
关键词
diffusion model training
AI 理解论文
溯源树
样例
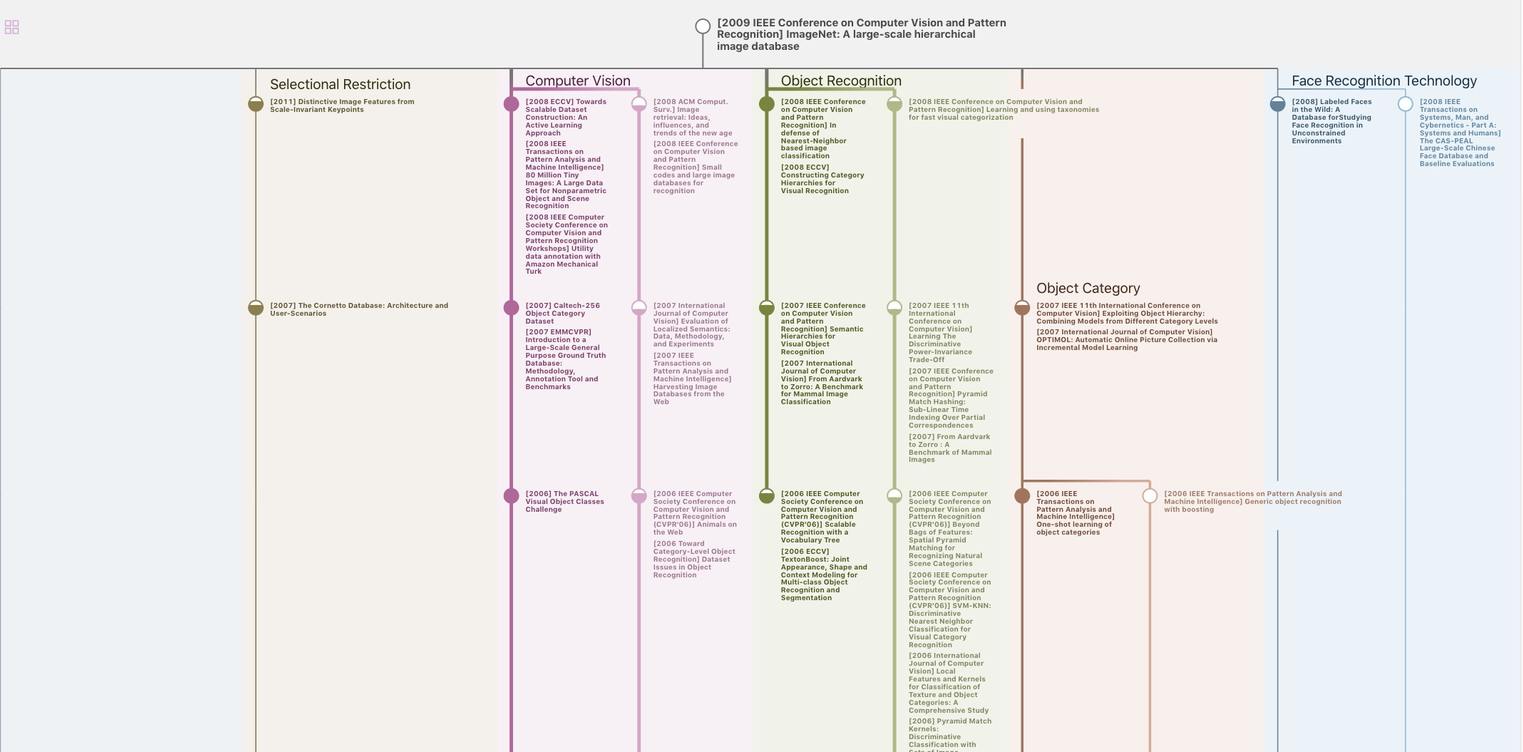
生成溯源树,研究论文发展脉络
Chat Paper
正在生成论文摘要