The Blessing of Randomness: SDE Beats ODE in General Diffusion-based Image Editing
arXiv (Cornell University)(2023)
摘要
We present a unified probabilistic formulation for diffusion-based image
editing, where a latent variable is edited in a task-specific manner and
generally deviates from the corresponding marginal distribution induced by the
original stochastic or ordinary differential equation (SDE or ODE). Instead, it
defines a corresponding SDE or ODE for editing. In the formulation, we prove
that the Kullback-Leibler divergence between the marginal distributions of the
two SDEs gradually decreases while that for the ODEs remains as the time
approaches zero, which shows the promise of SDE in image editing. Inspired by
it, we provide the SDE counterparts for widely used ODE baselines in various
tasks including inpainting and image-to-image translation, where SDE shows a
consistent and substantial improvement. Moreover, we propose SDE-Drag – a
simple yet effective method built upon the SDE formulation for point-based
content dragging. We build a challenging benchmark (termed DragBench) with
open-set natural, art, and AI-generated images for evaluation. A user study on
DragBench indicates that SDE-Drag significantly outperforms our ODE baseline,
existing diffusion-based methods, and the renowned DragGAN. Our results
demonstrate the superiority and versatility of SDE in image editing and push
the boundary of diffusion-based editing methods.
更多查看译文
关键词
Diffusion models,Stochastic differential equations,Image dragging,Image editing,Score-based models
AI 理解论文
溯源树
样例
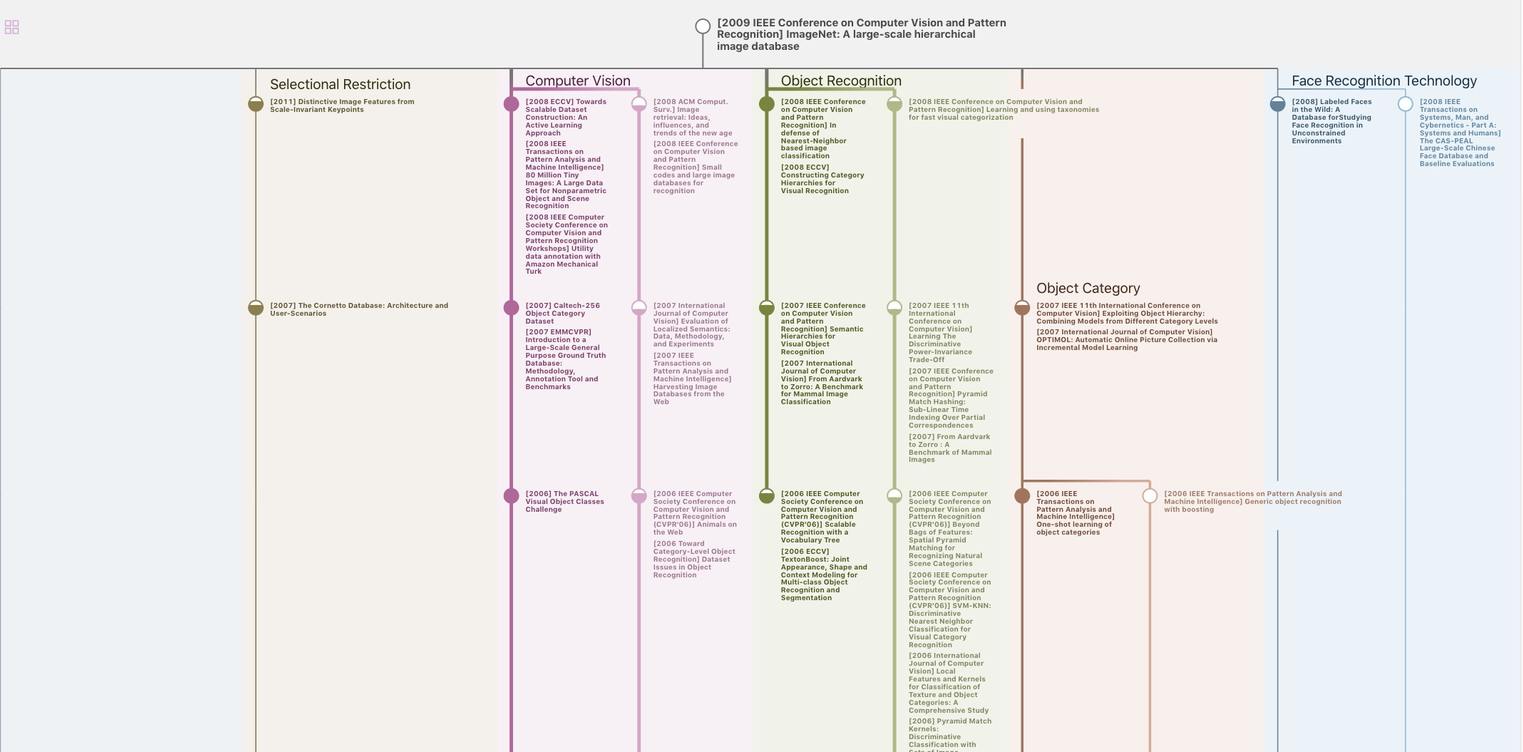
生成溯源树,研究论文发展脉络
Chat Paper
正在生成论文摘要