Degradation prediction of proton exchange membrane fuel cells with model uncertainty quantification
RENEWABLE ENERGY(2023)
摘要
This study presents a new approach for state of health (SOH) estimation and degradation trend prediction of proton exchange membrane fuel cells (PEMFCs) considering model uncertainty quantification. The model uncertainty is considered as completely unknown in this paper, and the Bayesian framework-based model uncertainty and state simultaneous estimation (Bayes-MUSE) method is proposed to quantify the model uncertainty from the perspective of the form of its effect on the system state transfer, and this method of quantifying model uncertainty is first applied to PEMFC long-term aging prediction and RUL estimation. The proposed Bayes-MUSE method is compared with extended Kalman filter (EKF), Unscented Kalman filter (UKF) and Unscented Particle filter (UPF) methods in terms of SOH estimation, noise immunity, aging prediction performance and RUL estimation using two aging test datasets, and the method is verified to have excellent noise immunity and the highest long-term aging prediction and RUL estimation accuracy. Compared with EKF, UKF and UPF methods, the proposed Bayes-MUSE method has a maximum improvement of 93.95% and 52.2% in RUL estimation accuracy of the two data sets under quasi-dynamic and static conditions, respectively.
更多查看译文
关键词
Aging prediction,Remaining useful life estimation,Fuel cell,Model uncertainty,Bayesian framework
AI 理解论文
溯源树
样例
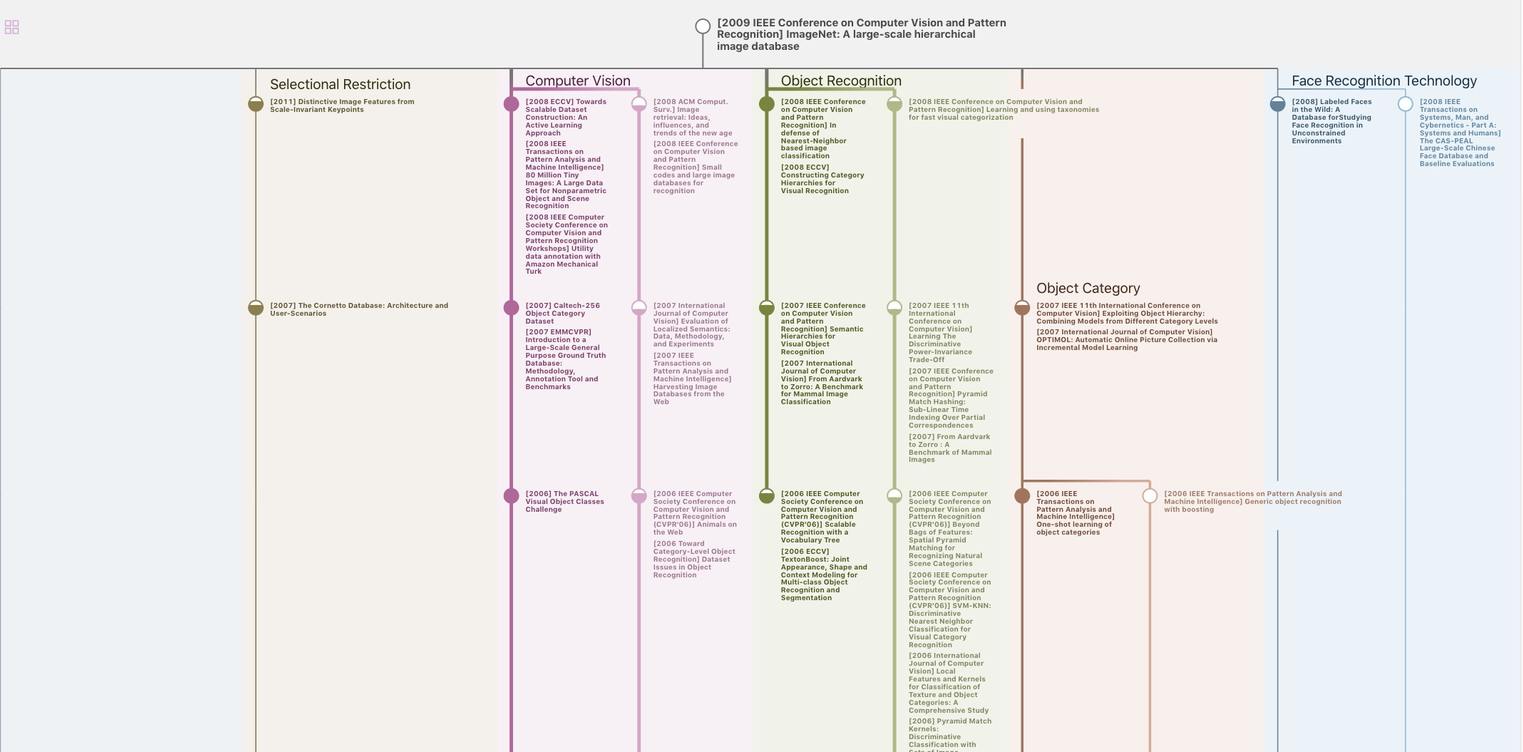
生成溯源树,研究论文发展脉络
Chat Paper
正在生成论文摘要