Identifying Surface-Enhanced Raman Spectra with a Raman Library Using Machine Learning
ACS nano(2023)
摘要
Since its discovery, surface-enhanced Raman spectroscopy (SERS) has shown outstanding promise of identifying trace amounts of unknown molecules in rapid, portable formats. However, the many different types of nanoparticles or nanostructured metallic SERS substrates created over the past few decades show substantial variability in the SERS spectra they provide. These inconsistencies have even raised speculation that substrate-specific SERS spectral libraries must be compiled for practical use of this type of spectroscopy. Here, we report a machine learning (ML) algorithm that can identify chemicals by matching their SERS spectra to those of a standard Raman spectral library. We use an approach analogous to facial recognition that utilizes feature extraction in the presence of multiple nuisance variables for spectral recognition. The key element is a metric we call "Characteristic Peak Similarity" (CaPSim) that focuses on the characteristic peaks in the SERS spectra. It has the flexibility to accommodate substrate-specific variability when quantifying the degree of similarity to a Raman spectrum. Analysis shows that CaPSim substantially outperforms existing spectral matching algorithms in terms of accuracy. This ML-based approach could greatly facilitate the spectroscopic identification of molecules in fieldable SERS applications.
更多查看译文
关键词
surface-enhanced Raman scattering, machinelearning, nanoparticles, polycyclic aromatic hydrocarbons, characteristic peak similarity
AI 理解论文
溯源树
样例
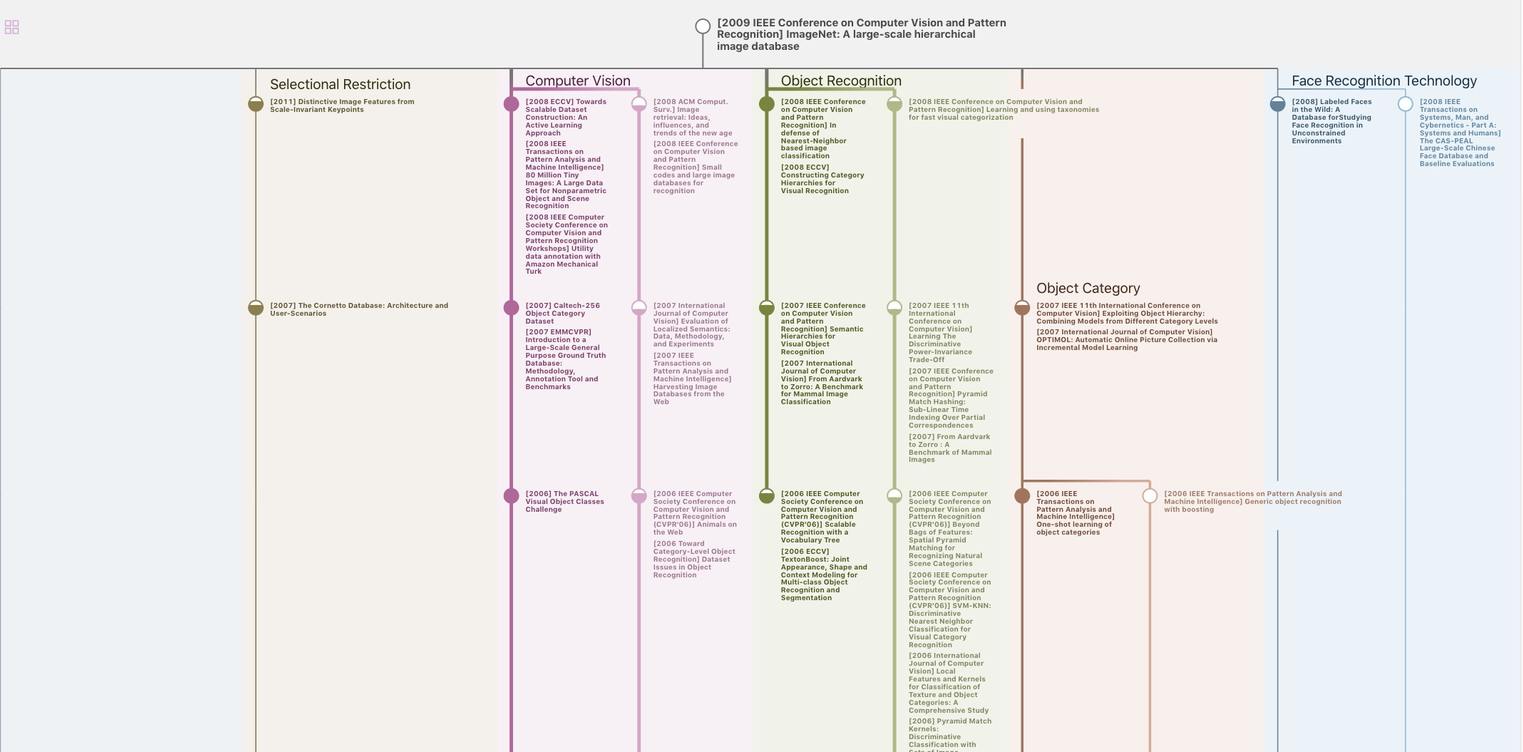
生成溯源树,研究论文发展脉络
Chat Paper
正在生成论文摘要