Multi-teacher Self-training for Semi-supervised Node Classification with Noisy Labels
MM '23: Proceedings of the 31st ACM International Conference on Multimedia(2023)
摘要
Graph neural networks (GNNs) have achieved promising results for semi-supervised learning tasks on the graph-structured data. However, most existing methods assume that the training data are with correct labels, but in the real world, the graph-structured data often carry noisy labels to reduce the effectiveness of GNNs. To address this issue, this paper proposes a new label correction method, called multi-teacher self-training (MTS-GNN for short), to conduct semi-supervised node classification with noisy labels. Specifically, we first save the parameters of the model training in the earlier iterations as teacher models, and then use them to guide the processes, including model training, noisy label removal, and pseudo-label selection, in the later iterations of the training process of semi-supervised node classification. As a result, based on the guidance of the teacher models, the proposed method achieves the model effectiveness by solving the over-fitting issue, improves the accuracy of noisy label removal and the quality of pseudo-label selection. Extensive experimental results on real datasets show that our method achieves the best effectiveness, compared to state-of-the-art methods.
更多查看译文
AI 理解论文
溯源树
样例
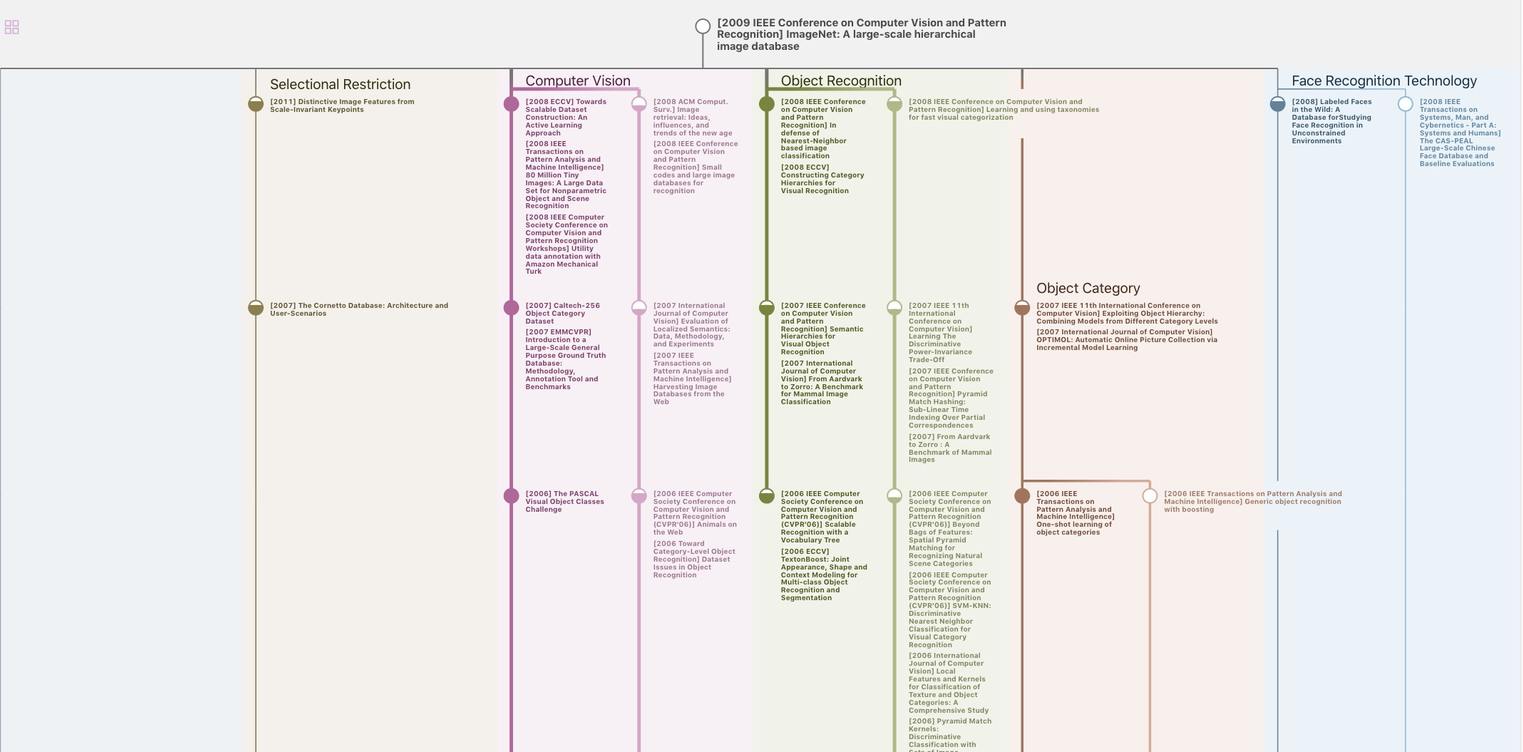
生成溯源树,研究论文发展脉络
Chat Paper
正在生成论文摘要