SAUNet: Spatial-Attention Unfolding Network for Image Compressive Sensing
MM '23: Proceedings of the 31st ACM International Conference on Multimedia(2023)
摘要
Image Compressive Sensing (CS) enables compressed capture of natural images via a spatial multiplexing camera and accurate reconstruction from few measurements via an advanced algorithm. Deep learning, especially deep unfolding, has recently achieved impressive success in image CS reconstruction. However, existing learning-based methods have been developed for block (usually with 33 X 33 pixels) CS instead of full image CS. Apart from the difficulties in hardware implementation, block CS breaks the global pixel interactions, limiting the overall performance. In this paper, we propose the first two-dimensional deep unfolding framework, and further develop a Spatial-Attention Unfolding Network (SAUNet) for full image CS reconstruction by alternately performing a spatially-adaptive gradient descent module and a cross-stage multi-scale denoising module. The gradient descent module has the spatial self-adaptation to the degradation of in-process image. The denoising module is a three-level U-shaped structure powered by Convolutional Self-Attention (CSA) mechanism. Inspired by Transformer, CSA is designed to adaptively aggregate spatially local information and adaptively recalibrate channel-wise global information with only normal convolutional operator. Extensive experiments demonstrate that SAUNet outperforms the state-of-the-art methods by a large margin. The source code and pre-trained models are available at https://github.com/pwangcs/SAUNet.
更多查看译文
AI 理解论文
溯源树
样例
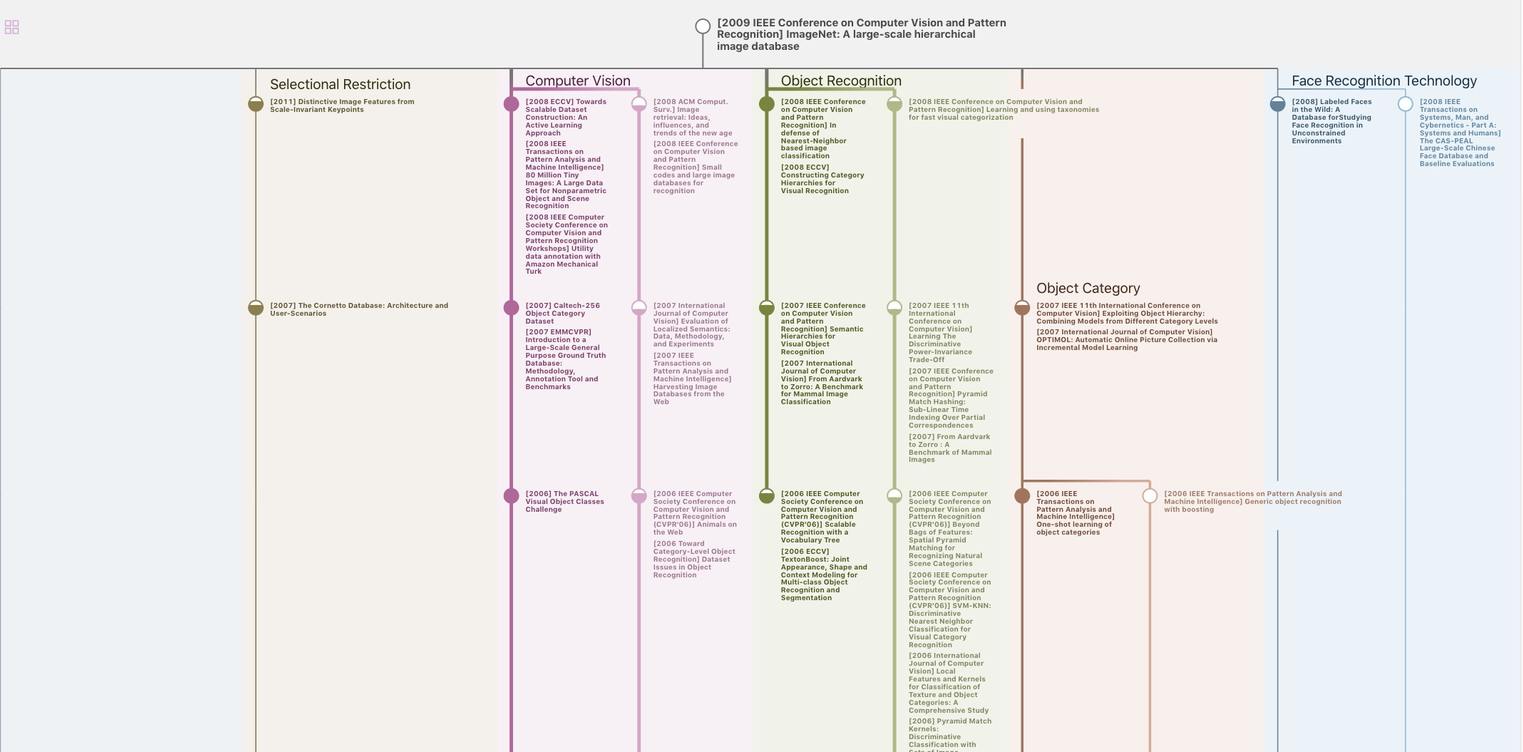
生成溯源树,研究论文发展脉络
Chat Paper
正在生成论文摘要