Diffused Fourier Network for Video Action Segmentation
MM '23: Proceedings of the 31st ACM International Conference on Multimedia(2023)
摘要
Video action segmentation aims to densely cast each video frame into a set of pre-defined human action categories. This work proposes a novel model, dubbed as diffused Fourier network (DFN) for video action segmentation. It advances the research frontier by addressing several central bottlenecks in the existing methods for video action segmentation. First, capturing long-range dependence among video frames is known to be crucial for precisely estimating the temporal boundaries for actions. Rather than relying on compute-intensive self-attention modules or stacking multi-rate dilated convolutions as in previous models (e.g., ASFormer), we devise Fourier token mixer over shiftable temporal windows in the video sequence, which harnesses the parameter-free and light-weighted Fast Fourier Transform (FFT) for efficient spectral-temporal feature learning. Essentially, even simple spectral operations (e.g., pointwise product) bring global receptive field across the entire temporal window. The proposed Fourier token mixer thus provides a low-cost alternative for existing practice. Secondly, the results of action segmentation tend to be fragmented, primarily due to the noisy per-frame action likelihood, known as over-segmentation in the literature. Inspired by the recently-proposed diffusion models, we treat over-segments as noises corrupting the true temporal boundaries, and conduct denoising via a recurrent execution of a parameter-sharing module, akin to the backward denoising process in the diffusion models. Comprehensive experiments on three video benchmarks (GTEA, 50salads and Breakfast) have clearly validated that the proposed method can strike an excellent balance between computations / parameter count and accuracy.
更多查看译文
AI 理解论文
溯源树
样例
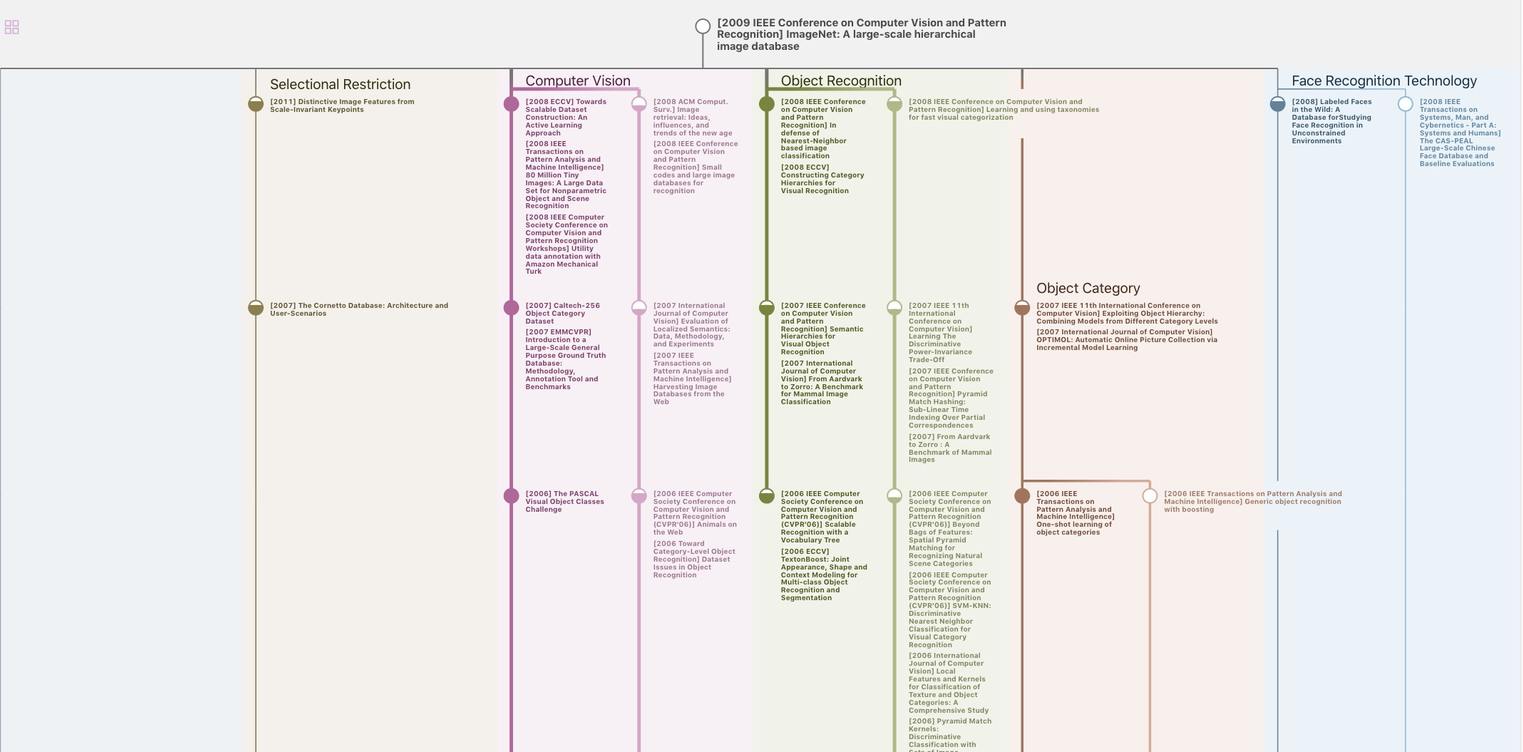
生成溯源树,研究论文发展脉络
Chat Paper
正在生成论文摘要