Hierarchical Prompt Learning Using CLIP for Multi-label Classification with Single Positive Labels
MM '23: Proceedings of the 31st ACM International Conference on Multimedia(2023)
摘要
Collecting full annotations to construct multi-label datasets is difficult and labor-consuming. As an effective solution to relieve the annotation burden, single positive multi-label learning (SPML) draws increasing attention from both academia and industry. It only annotates each image with one positive label, leaving other labels unobserved. Therefore, existing methods strive to explore the cue of unobserved labels to compensate for the insufficiency of label supervision. Though achieving promising performance, they generally consider labels independently, leaving out the inherent hierarchical semantic relationship among labels which reveals that labels can be clustered into groups. In this paper, we propose a hierarchical prompt learning method with a novel Hierarchical Semantic Prompt Network (HSPNet) to harness such hierarchical semantic relationships using a large-scale pretrained vision and language model, i.e., CLIP, for SPML. We first introduce a Hierarchical Conditional Prompt (HCP) strategy to grasp the hierarchical label-group dependency. Then we equip a Hierarchical Graph Convolutional Network (HGCN) to capture the high-order inter-label and inter-group dependencies. Comprehensive experiments and analyses on several benchmark datasets show that our method significantly outperforms the state-of-the-art methods, well demonstrating its superiority and effectiveness. Our code will be available at https://github.com/jameslahm/HSPNet.
更多查看译文
AI 理解论文
溯源树
样例
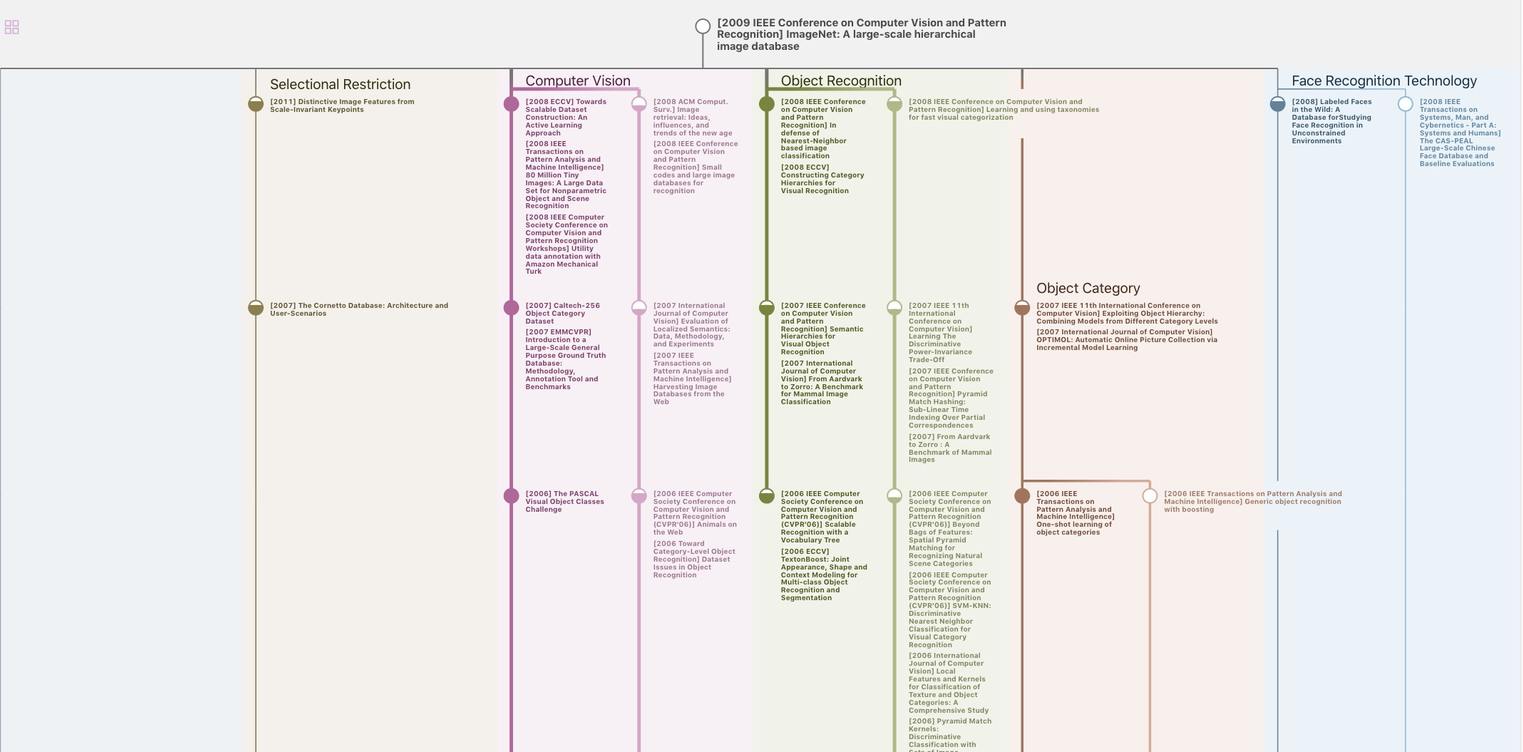
生成溯源树,研究论文发展脉络
Chat Paper
正在生成论文摘要