Filling the Information Gap between Video and Query for Language-Driven Moment Retrieval
MM '23: Proceedings of the 31st ACM International Conference on Multimedia(2023)
摘要
This paper addresses the challenging task of language-driven moment retrieval. Previous methods are typically trained to localize the target moment corresponding to a single sentence query in a complicated video. However, this specific moment generally delivers richer contents than the query, i.e., the semantics of one query may miss certain object details or actions in the complex foreground-background visual contents. Such information imbalance between two modalities makes it difficult to finely align their representations. To this end, instead of training with a single query, we propose to utilize the diversity and complementarity among different queries corresponding to the same video moment for enriching the textual semantics. Specifically, we develop a Teacher-Student Moment Retrieval (TSMR) framework to fill this cross-modal information gap. A teacher model is trained to not only encode a certain query but also capture extra complementary queries to aggregate contextual semantics for obtaining more comprehensive moment-related query representations. Since the additional queries are inaccessible during inference, we further introduce an adaptive knowledge distillation mechanism to train a student model with a single query input by selectively absorbing the knowledge from the teacher model. In this manner, the student model is more robust to the cross-modal information gap during the moment retrieval guided by a single query. Experimental results on two benchmarks demonstrate the effectiveness of our proposed method.
更多查看译文
AI 理解论文
溯源树
样例
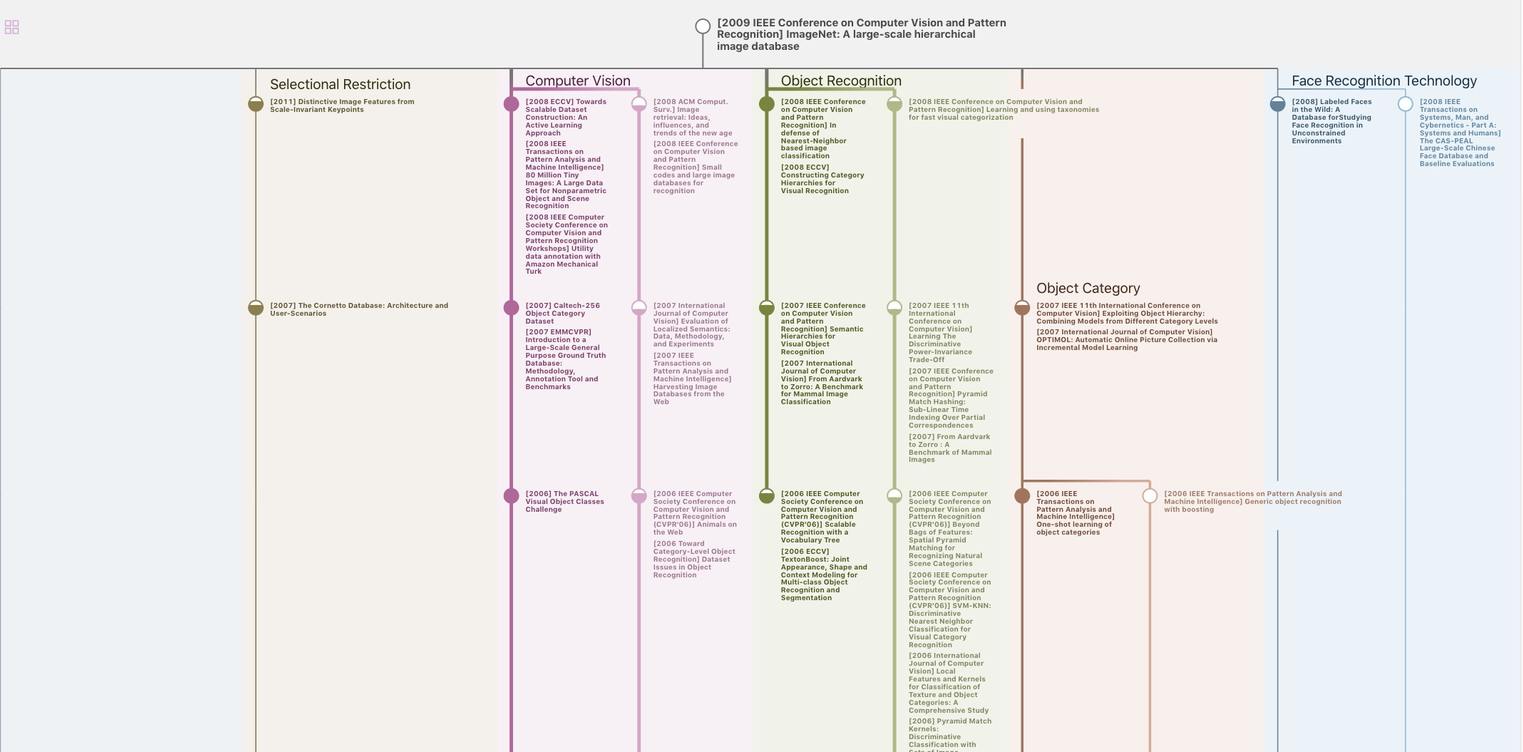
生成溯源树,研究论文发展脉络
Chat Paper
正在生成论文摘要