Exploring the Power of Cross-Contextual Large Language Model in Mimic Emotion Prediction
MuSe '23: Proceedings of the 4th on Multimodal Sentiment Analysis Challenge and Workshop: Mimicked Emotions, Humour and Personalisation(2023)
摘要
utilize multimodal data to predict the intensity of three emotional categories. In our work, we discovered that integrating multiple dimensions, modalities, and levels enhances the effectiveness of emotional judgment. In terms of feature extraction, we utilize over a dozen types of medium backbone networks, including W2V-MSP, GLM, and FAU, which are representative of audio, text, and video modalities, respectively. Additionally, we utilize the LoRA framework and employ various domain adaptation methods to effectively adapt to the task at hand. Regarding model design, apart from the RNN model in the baseline, we have extensively incorporated our transformer variant and multi-modal fusion model. Finally, we propose a Hyper-parameter Search Strategy (HPSS) for late fusion to further enhance the effectiveness of the fusion model. For the MuSe-MIMIC, our method achieves Pearson's Correlation Coefficient of 0.7753, 0.7647, and 0.6653 for Approval, Disappointment, and Uncertainty, respectively, outperforming the baseline system by a large margin (i.e., 0.5536, 0.5139, and 0.3395) on the test set. The final mean pearson is 0.7351, surpassing all other participants and ranking Top 1.
更多查看译文
AI 理解论文
溯源树
样例
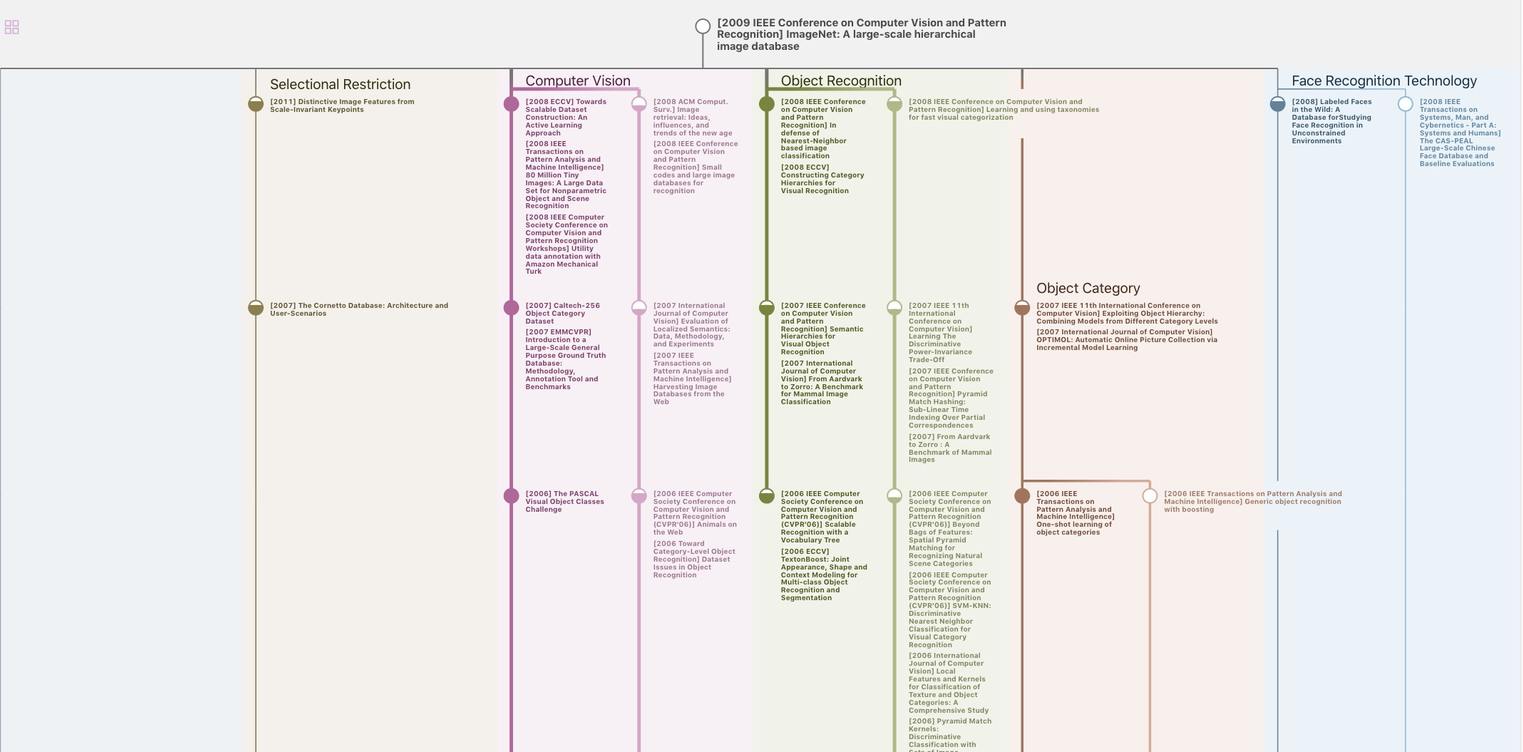
生成溯源树,研究论文发展脉络
Chat Paper
正在生成论文摘要