ENTRO: Tackling the Encoding and Networking Trade-off in Offloaded Video Analytics
MM '23: Proceedings of the 31st ACM International Conference on Multimedia(2023)
摘要
With the rapid advances of deep learning and the commercialization of high-definition cameras in mobile and embedded devices, the demands from latency-critical applications such as AR and XR for high-quality video analytics (HVA) are soaring. By the nature of HVA aiming at enabling detailed analytics even for small objects, its on-device implementation is suffering from thermal and battery issues, which makes offloaded HVA an attractive solution. This work provides unique observations on the tradeoff pertaining to offloaded HVA: the frame encoding time, the frame transmission time, and the HVA accuracy. Our observations pose a fundamental question: given a latency budget, how to choose the encoding option that properly combines between the encoding time and the transmission time to maximize the HVA accuracy. To answer this question, we propose an offloaded HVA system, ENTRO, which exploits this tradeoff in real-time to maximize the HVA accuracy under the latency budget. Our extensive evaluations with ENTRO implemented on Nvidia AGX Xavier and Samsung Galaxy S20 Ultra over WiFi networks show 8.8× improvement in latency without accuracy loss compared to DDS, the state-of-the-art offloaded video analytics. Our evaluation over commercial 5G and LTE networks also indicates that ENTRO flexibly adapts its encoding option under the tradeoff and enables the latency-bounded HVA with 4K frames.
更多查看译文
AI 理解论文
溯源树
样例
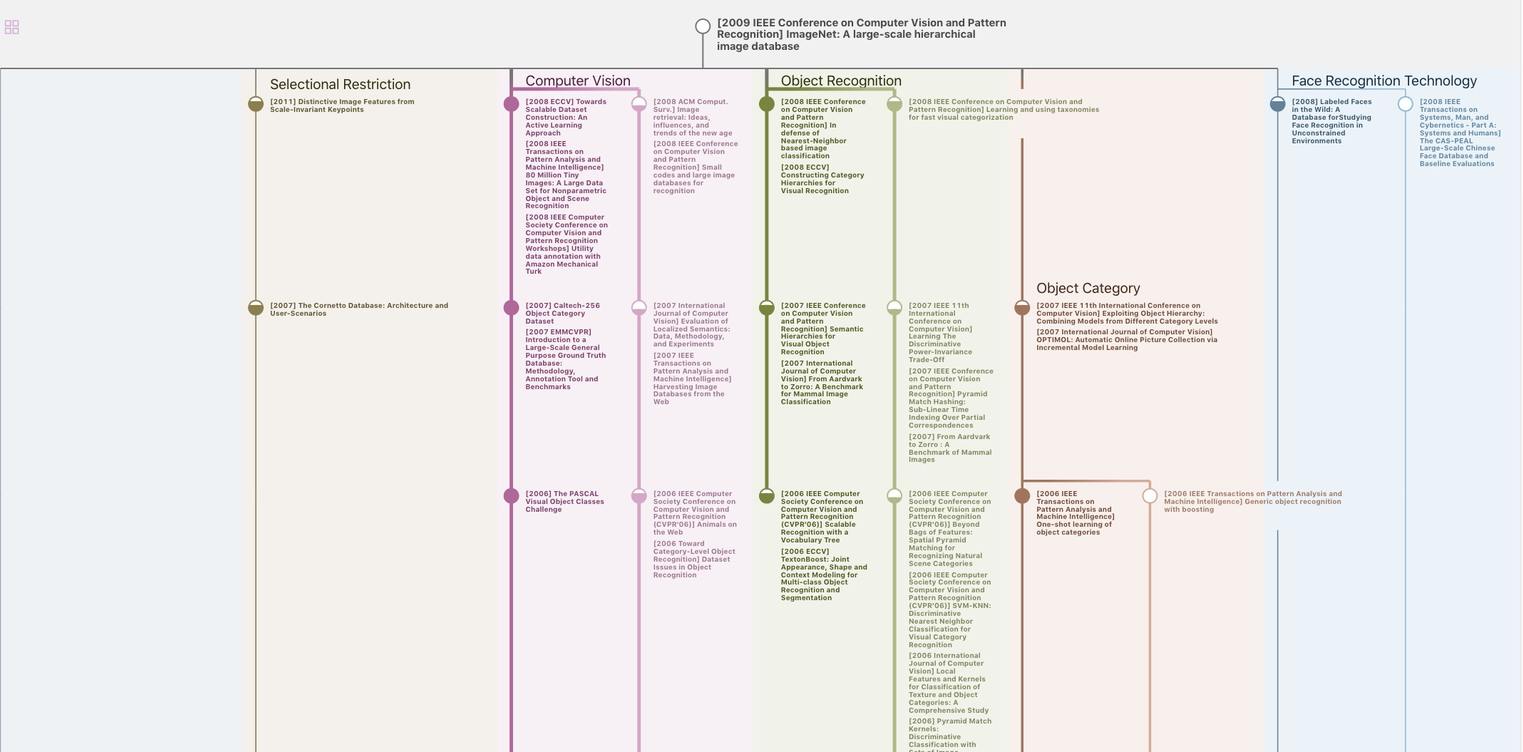
生成溯源树,研究论文发展脉络
Chat Paper
正在生成论文摘要