A Lightweight Collective-attention Network for Change Detection
MM '23: Proceedings of the 31st ACM International Conference on Multimedia(2023)
摘要
Change detection of multi-temporal remote sensing images is mushrooming with the innovations of neural networks, whose daunting challenge lies in locating sporadically distributed spatial-temporal changes given sophisticated scenes and various imaging conditions. Unfortunately, instead of devoting full attention to changes, most existing solutions often expend unnecessary resources yet derive task-irrelevant features. To relieve this issue, we propose a collective-attention network, which enjoys lightweight model architecture yet guarantees high performance. Specifically, an inter-temporal collective-attention module is developed for efficient interaction of bi-temporal features, in which a shared attention distribution is derived via the multiplication of temporal-concatenated queries and spatial-subtracted keys. Additionally, we present a non-change consistency-constraint, enforcing a change-oriented attention distribution and a noise-suppressed treatment. With the learned interaction features, bi-temporal differences are captured simply using the operations of spatial absolute error and temporal concatenation. Finally, decoding multi-scale differences is accomplished by lightweight temporal self-attention and spatial self-attention. Experiments on four datasets demonstrate that our model achieves state-of-the-art performance, yet requires only 1.71M parameters and 1.98G FLOPs.
更多查看译文
AI 理解论文
溯源树
样例
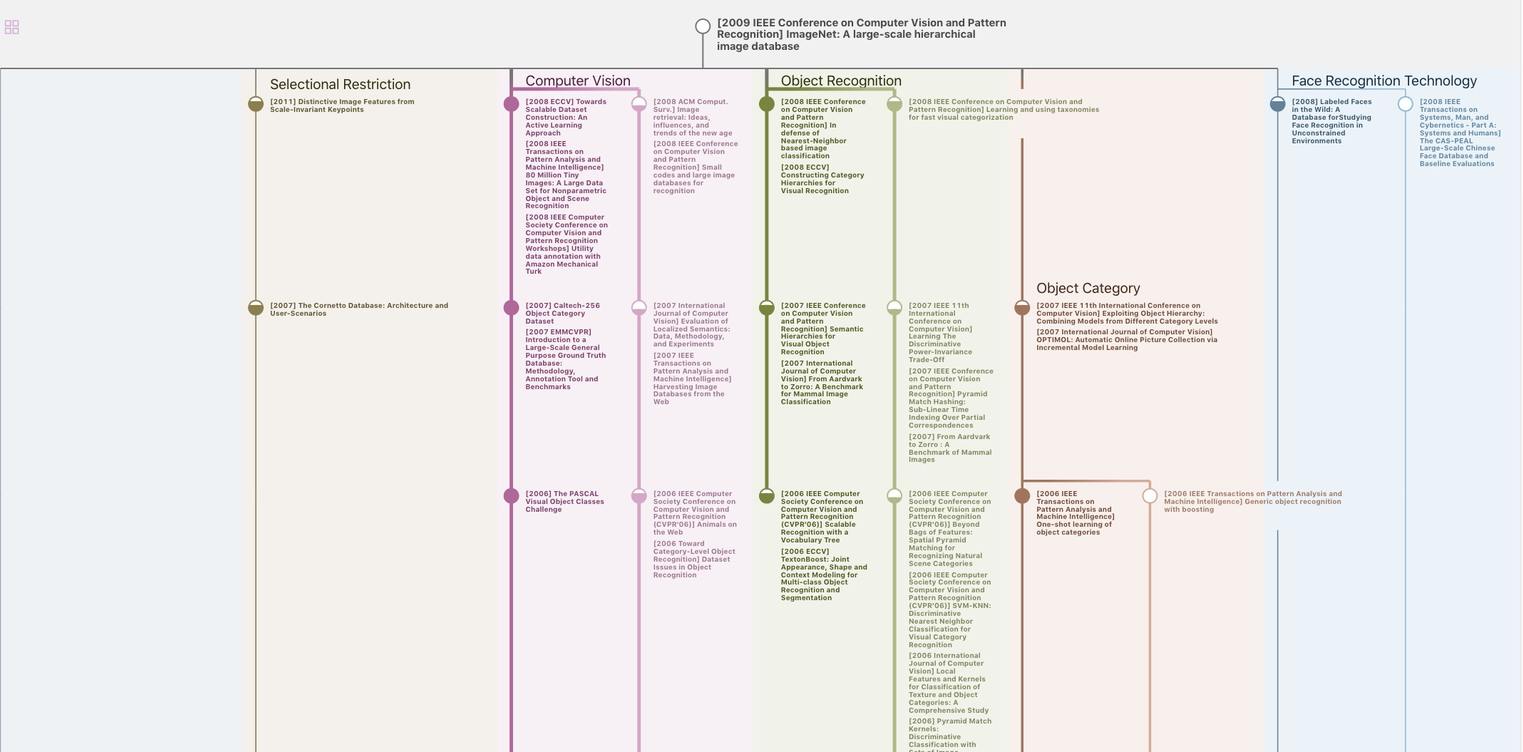
生成溯源树,研究论文发展脉络
Chat Paper
正在生成论文摘要