DuDoINet: Dual-Domain Implicit Network for Multi-Modality MR Image Arbitrary-scale Super-Resolution
MM '23: Proceedings of the 31st ACM International Conference on Multimedia(2023)
摘要
Compared to single-modality magnetic resonance (MR) image super-resolution (SR) methods, multi-modality MR image methods can utilize high-resolution reference modality (e.g., T1 modality) to provide valuable complementary information for low-resolution target modality (e.g., T2 modality) in SR reconstruction, which can further improve the quality of the SR images. Although they have achieved impressive results, these methods still suffer from the following drawbacks: (1) They can only handle fixed integer upsampling factors, such as 2X, 3X, and 4X, and require training and storing corresponding models for each upsampling factor, which is infeasible in clinical practice; (2) They only perform feature extraction and reconstruction in the image domain. However, the aliasing artifacts produced in the image domain are structural and non-local. Therefore, using only the image domain cannot effectively reconstruct high-quality aliasing-free SR images. To address these issues, we develop a brand-new Dual-Domain Implicit Network (DuDoINet) for multi-modality MR image arbitrary-scale SR. Specifically, we propose a dual-domain learning scheme for multi-modality MR image SR, which allows the network to sufficiently exploit the frequency and image domain information in MR images. In addition, we design implicit attention to achieve arbitrary-scale upsampling of MR images, which utilizes a continuously differentiable function that generates pixel values from pixel coordinates. Furthermore, we designed a deformable cross-modality attention mechanism that can adaptively transfer high-frequency details from the T1 to the T2 modality, better integrating valuable complementary information from the T1 modality. Extensive and comprehensive experiments on healthy subjects and patient datasets demonstrate that our DuDoINet outperforms SOTA methods, demonstrating its great potential for clinical practice.
更多查看译文
AI 理解论文
溯源树
样例
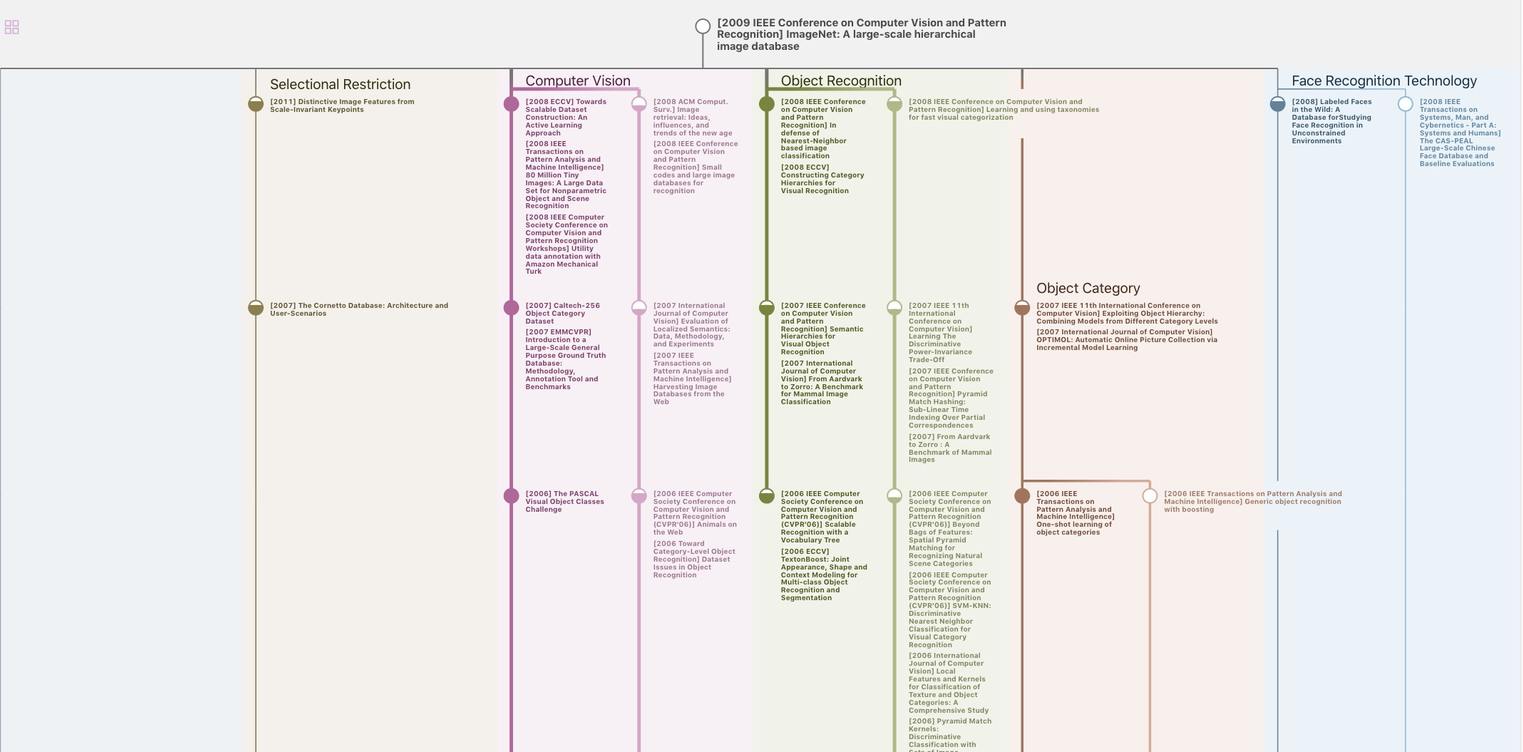
生成溯源树,研究论文发展脉络
Chat Paper
正在生成论文摘要