Incremental Few Shot Semantic Segmentation via Class-agnostic Mask Proposal and Language-driven Classifier
MM '23: Proceedings of the 31st ACM International Conference on Multimedia(2023)
摘要
Incremental Few-Shot Semantic Segmentation (IFSS) aims to extend pre-trained segmentation models to new classes with limited annotated images without accessing old training data. During incrementally learning novel classes, the data distribution of old classes will be corrupted, leading to catastrophic forgetting. Meanwhile, the samples of the new class are limited, making it impossible for the model to learn a satisfactory representation of the new class. Previous IFSS methods are mainly based on distillation or storing old data. In this paper, we propose a new IFSS framework called CaLNet, i.e., Class-agnostic mask proposal and Language-driven classifier incremental few-shot semantic segmentation network. Specifically, CaLNet employs a class-agnostic mask proposal, and due to its class-agnostic nature, the capabilities of mask proposals can be easily extended from base classes to novel classes. As a result, incremental learning is only needed in the classifier part. Meanwhile, when incrementally learning novel classes, it is challenging for the classifier to learn a complete representation of the new classes due to the limited number of samples. Based on this, we combine the language embedding into the visual features, making the expression of the new class complete. Results on Pascal-VOC and COCO show that CaLNet achieves a new SOTA.
更多查看译文
AI 理解论文
溯源树
样例
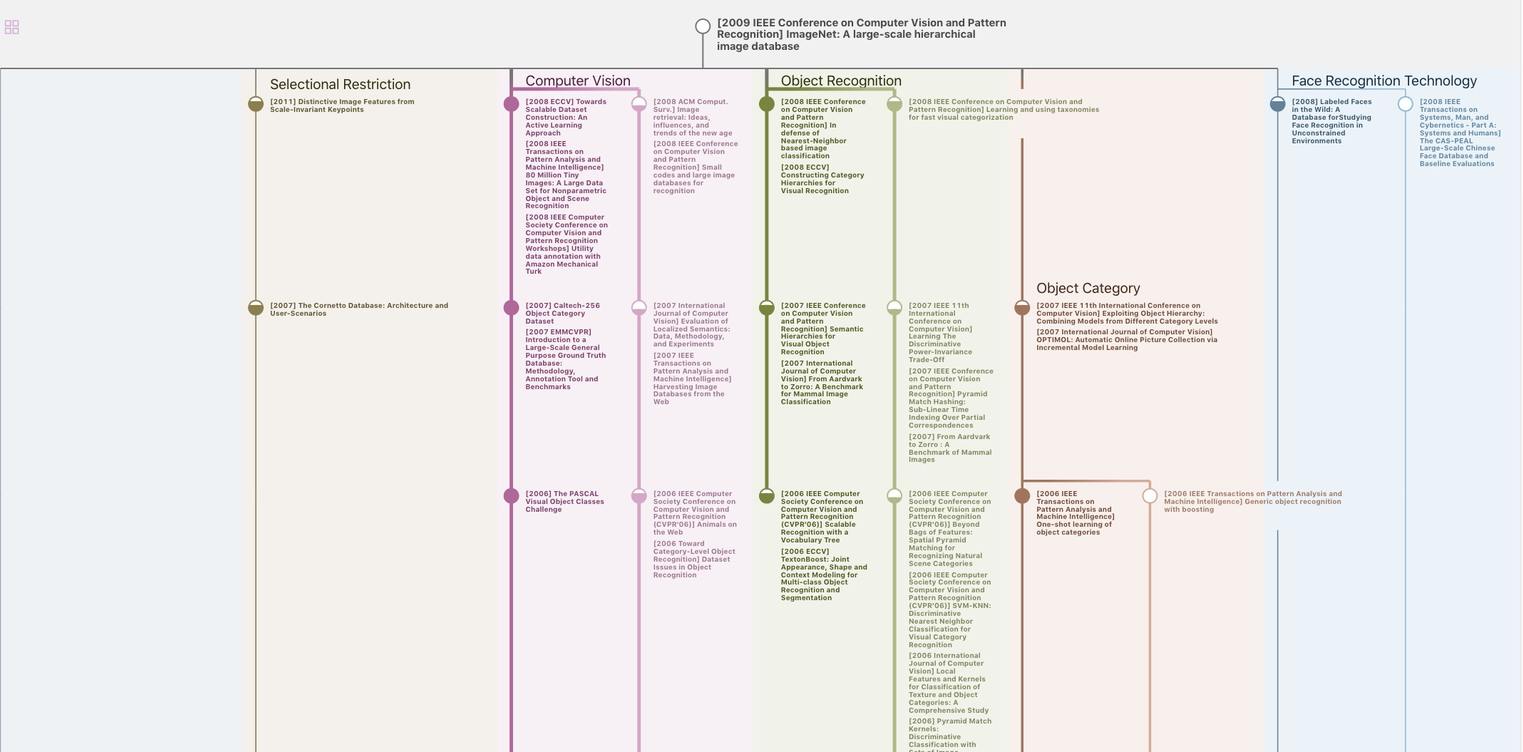
生成溯源树,研究论文发展脉络
Chat Paper
正在生成论文摘要