A Comparative Analysis of Sensor-, Geometry-, and Neural-Based Methods for Food Volume Estimation.
MADiMa '23: Proceedings of the 8th International Workshop on Multimedia Assisted Dietary Management(2023)
摘要
With the rapid advancements in artificial intelligence and computer vision within health and nutrition fields, image-based automatic dietary assessment is gaining popularity. This automation involves food segmentation, recognition, volume estimation, and estimation of nutritional content. While considerable progress has been made in food segmentation and recognition, accurate volume estimation remains challenging. Measuring food volume is crucial in many fields, even thought this is difficult to automate precisely. This is hampering progress, and is leading to continued reliance on time-consuming traditional methods, such as manual computation of food volume through water displacement. The manuscript presents a comparative analysis of sensor-, geometry-, and neural-based methods for computing food volume. We have performed multiple experiments using 20 meal images captured under different settings, with reliable measurements of ground-truth volume obtained by capturing 360-degree views of the food items and computing their volumes in a 3D space. An extensive analysis of our results then serves to identify the strengths and limitations of each approach, and offers valuable insights for selecting the most suitable method in specific settings. Moreover, we have made the collected data (including RGB images, ground-truth point clouds, volumes, etc.) open-source. We intend this as a contribution to the research community and to address the scarcity of food datasets with depth-related information.
更多查看译文
AI 理解论文
溯源树
样例
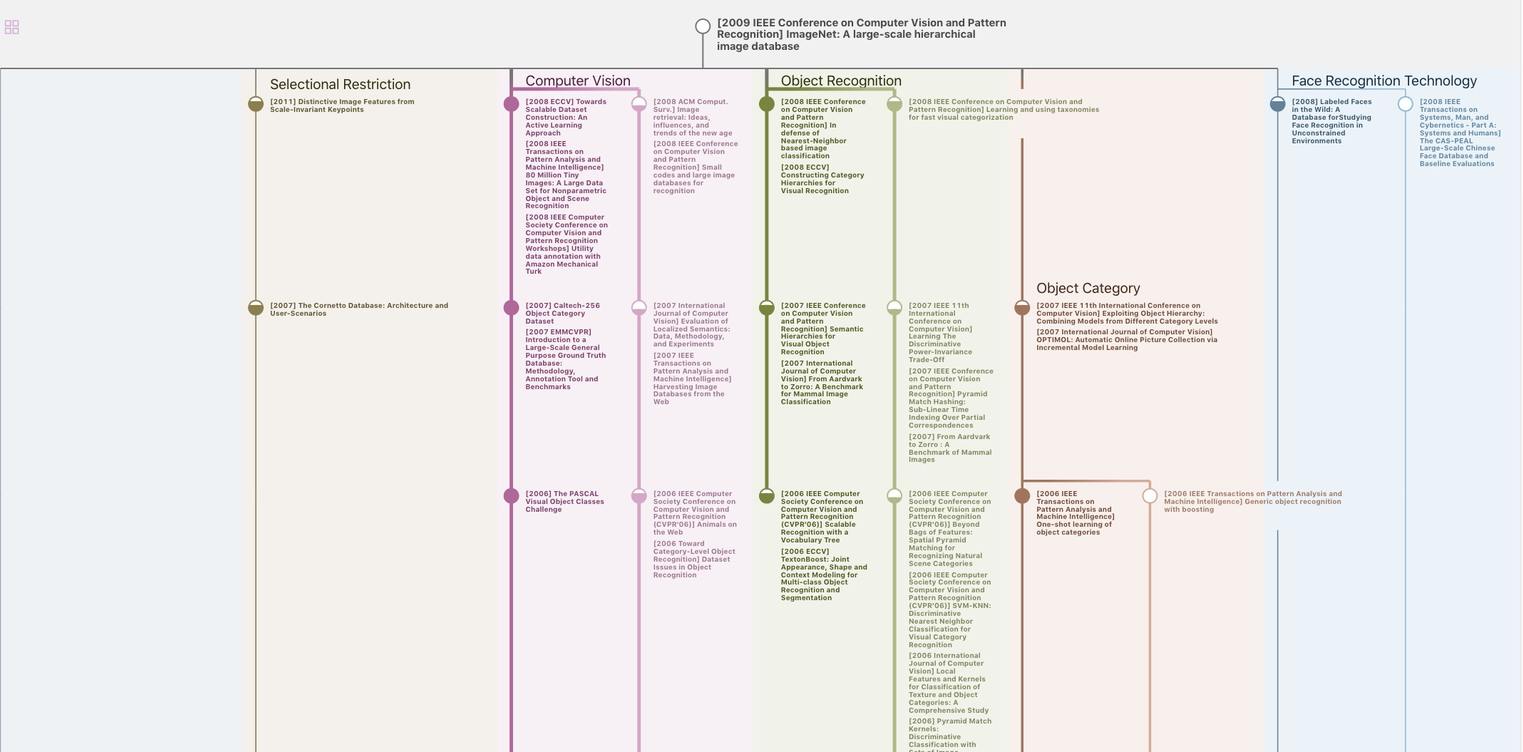
生成溯源树,研究论文发展脉络
Chat Paper
正在生成论文摘要