Detecting Temporal Inconsistency in Biased Datasets for Android Malware Detection
2023 38th IEEE/ACM International Conference on Automated Software Engineering Workshops (ASEW)(2023)
摘要
Machine learning (ML) has exhibited great potential in Android malware detection. Yet, the reliability of these ML models, as well as the fairness of their evaluation, hinge significantly on the quality of the datasets used. A significant issue compromising these aspects is the presence of temporal inconsistencies within datasets, which could lead to overestimated detection performance. While previous research has acknowledged the impact of temporal inconsistencies, the proposed detection approaches often falter in accuracy and practicality. Previous studies have had limitations when it comes to dealing with complex cases of temporal inconsistencies. Additionally, their approaches require knowledge of a dataset's temporal attributes, which is often not realistic in real-world applications. In response to these challenges, we propose a novel ML-based approach to comprehensively and effectively detect temporal inconsistencies in Android malware datasets, regardless of the magnitude of these inconsistencies. Distinguishing itself from prior attempts, our approach accurately identifies inconsistencies in unknown datasets, without making any assumptions about their temporal attributes. Moreover, we introduce a new benchmark dataset of 78,000 diverse Android samples, spanning malware to benign samples from 2010 to 2022, for exploring temporal inconsistency. A rigorous evaluation of our approach using this dataset reveals its proficiency in managing temporal inconsistencies, achieving a remarkable 98.3% detection accuracy. We further validate the efficacy of our feature selection procedure and demonstrate the robustness of our approach when applied to unknown datasets. Collectively, our findings pioneer a novel performance standard in Android malware detection assessments, contributing to the enhancement of reliability in ML-based techniques.
更多查看译文
AI 理解论文
溯源树
样例
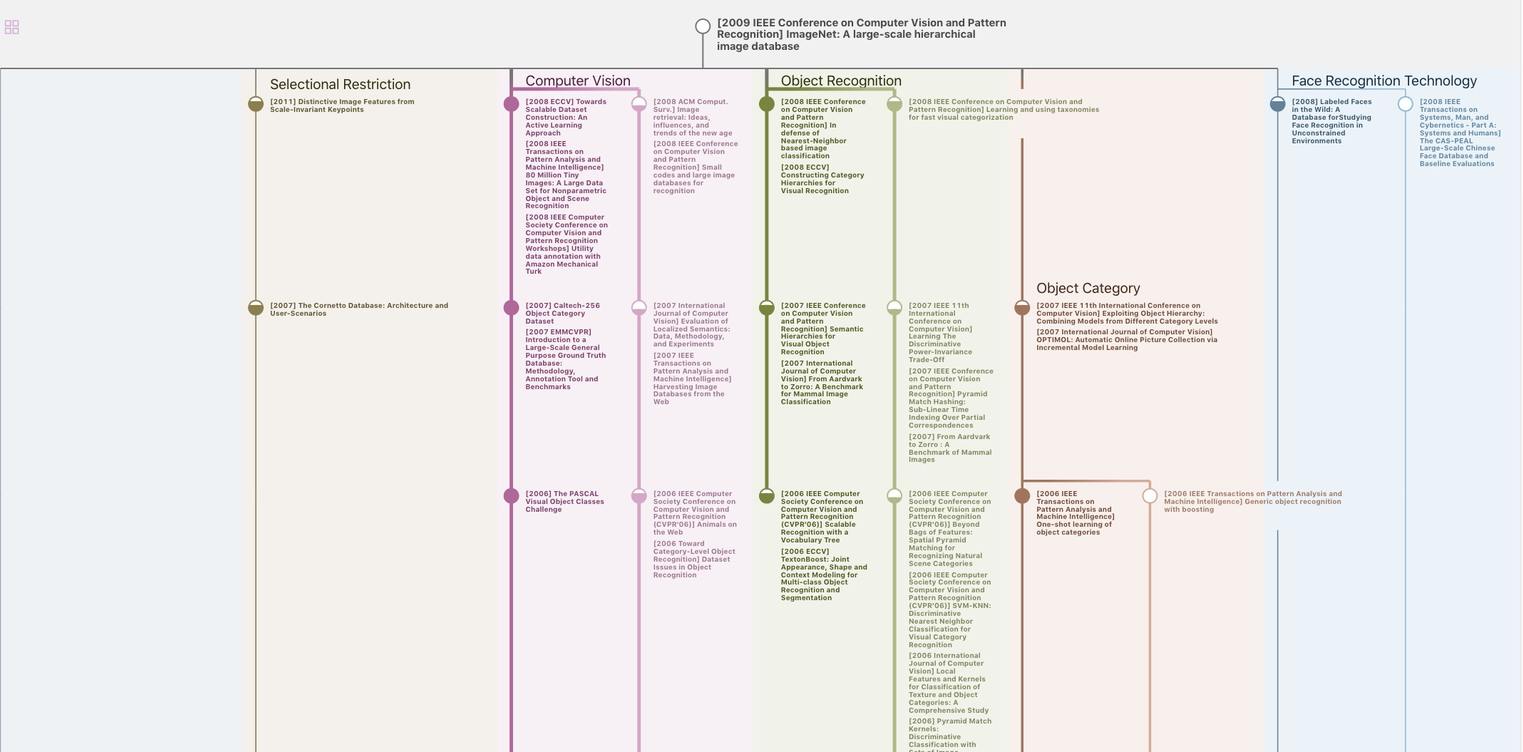
生成溯源树,研究论文发展脉络
Chat Paper
正在生成论文摘要