Numerical Analysis of a Self-Calibrating Time-Spatial Interleaving Photonic Convolutional Accelerator
2023 International Conference on Photonics in Switching and Computing (PSC)(2023)
摘要
Convolutional Neural Networks (CNNs) are fundamental machine learning tools to process image, speech, or audio signal inputs. The convolutional layer is the core building block of a CNN, and it is where most of the computation occurs. Here, we propose an integrated photonic convolutional accelerator based on time-spatial interleaving utilizing standard generic building blocks to reduce hardware complexity. The architecture is suitable for addressing both 2D and 1D convolutional kernels enabling scalability to more complex networks. Furthermore, a numerical simulation demonstrates the viability of a supervised online learning algorithm for loading the kernel weights both in amplitude and in phase taking in consideration fabrication tolerances and thermal cross-talk.
更多查看译文
关键词
Photonics,Computing,Convolution,Machine Learning
AI 理解论文
溯源树
样例
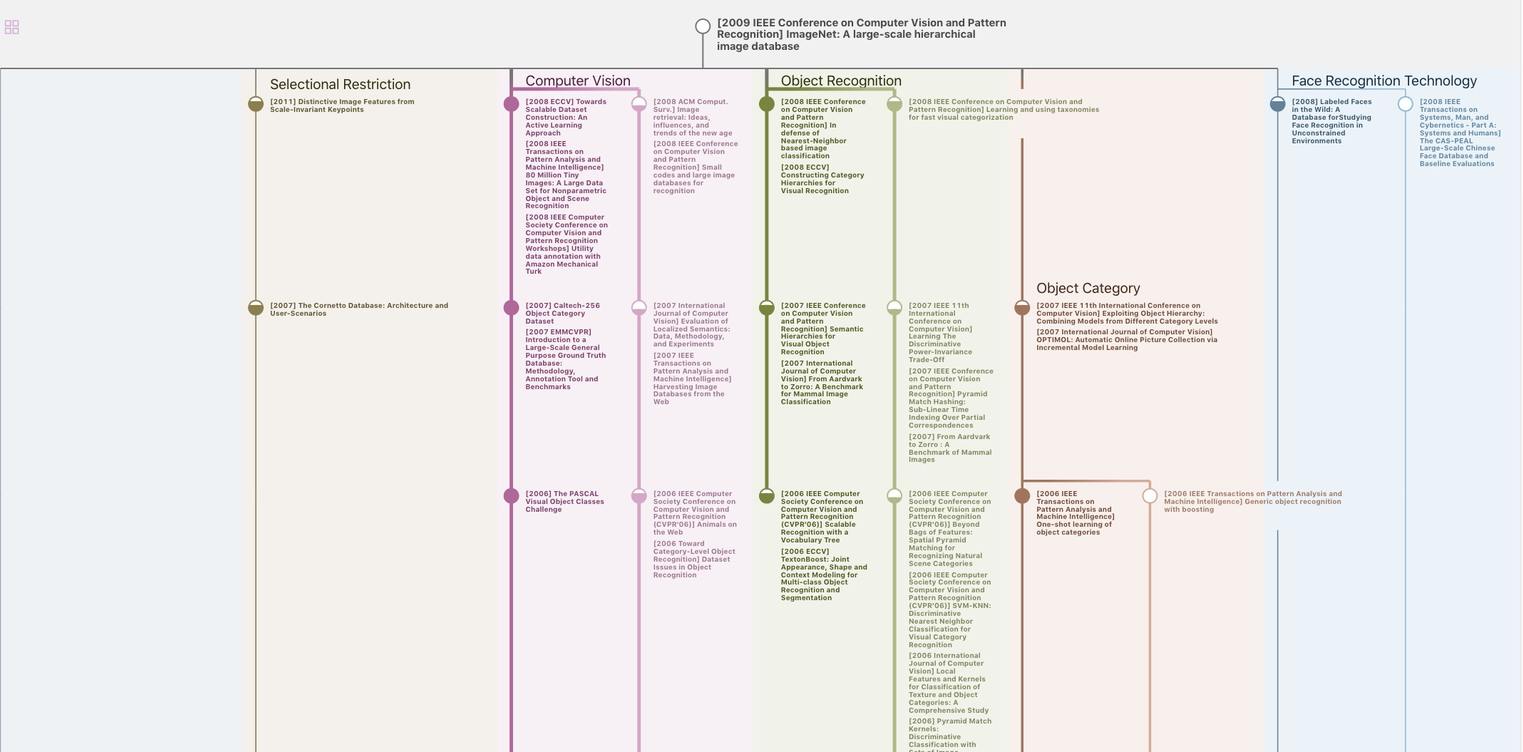
生成溯源树,研究论文发展脉络
Chat Paper
正在生成论文摘要