Search-based Test Case Selection for PLC Systems Using Functional Block Diagram Programs
2023 IEEE 34TH INTERNATIONAL SYMPOSIUM ON SOFTWARE RELIABILITY ENGINEERING, ISSRE(2023)
关键词
programmable logic controller,functional block diagram,test case selection,regression testing,search-based testing
AI 理解论文
溯源树
样例
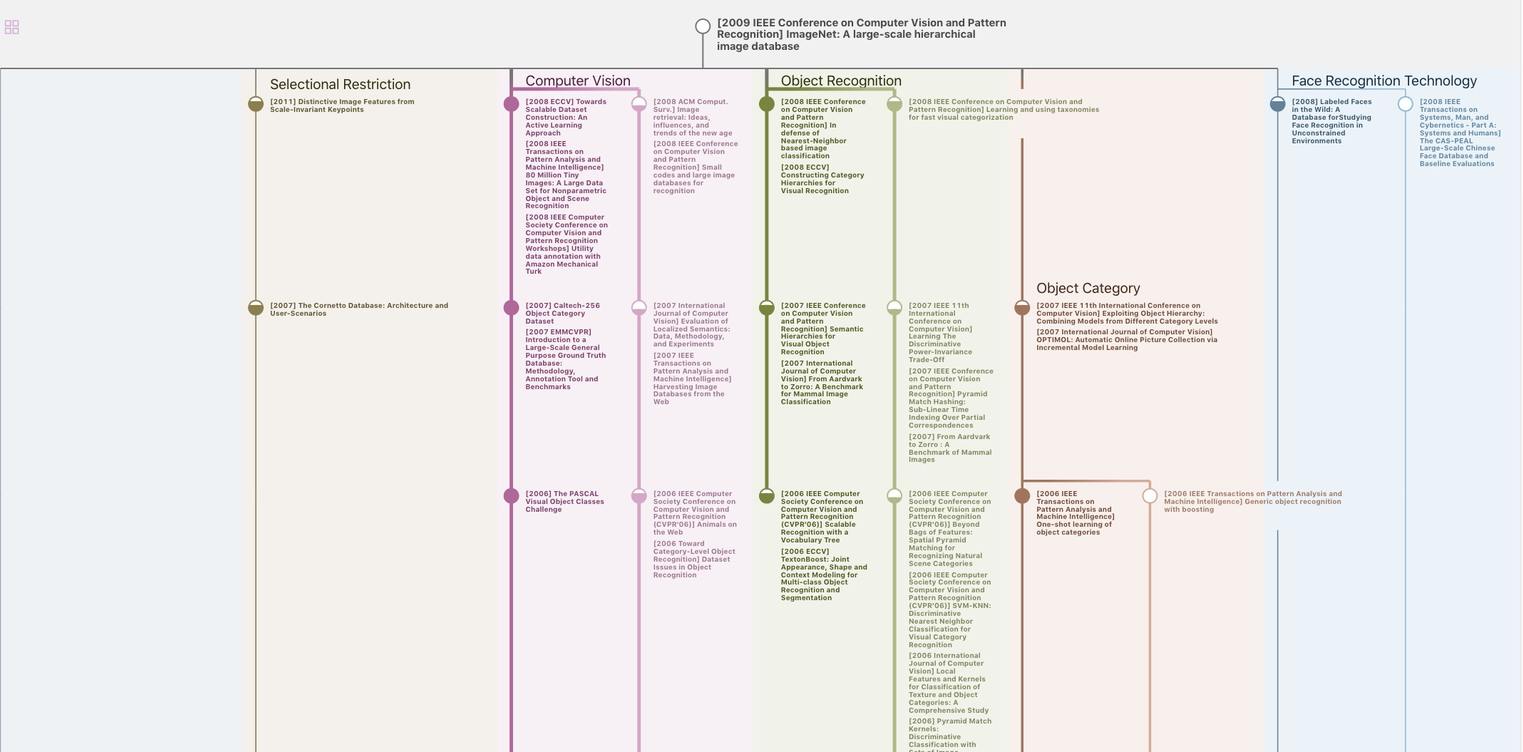
生成溯源树,研究论文发展脉络
Chat Paper
正在生成论文摘要