Contrastive Learning for Target Speaker Extraction With Attention-Based Fusion
IEEE-ACM TRANSACTIONS ON AUDIO SPEECH AND LANGUAGE PROCESSING(2024)
摘要
Given a reference speech clip from the target speaker, Target Speaker Extraction (TSE) is a challenging task that involves extracting the signal of the target speaker from a multi-speaker environment. TSE networks typically comprise a main network and an auxiliary network. The former utilizes the obtained target speaker embedding to generate an appropriate mask for isolating the signal of the target speaker from those of other speakers, while the latter aims to learn deep discriminative embeddings from the signal of the target speaker. However, the TSE networks often face performance degradation when dealing with unseen speakers or speeches with short references. In this article, we propose a novel approach that leverages contrastive learning in the auxiliary network to obtain better representations of unseen speakers or speeches with short references. Specifically, we employ contrastive learning to bridge the gap between short and long speech features. In this case, the auxiliary network with the input of a short speech generates feature embeddings that are as rich as those obtained from a long speech. Therefore, improving the recognition of unseen speakers or short speeches. Moreover, we introduce an attention-based fusion method that integrates the speaker embedding into the main network in an adaptive manner for enhancing mask generation. Experimental results demonstrate the effectiveness of our proposed method in improving the performance of TSE tasks in realistic open scenarios.
更多查看译文
关键词
Feature extraction,Task analysis,Visualization,Speech enhancement,Decoding,Degradation,Transformers,Speaker extraction,self-supervised learning,contrastive learning,attention
AI 理解论文
溯源树
样例
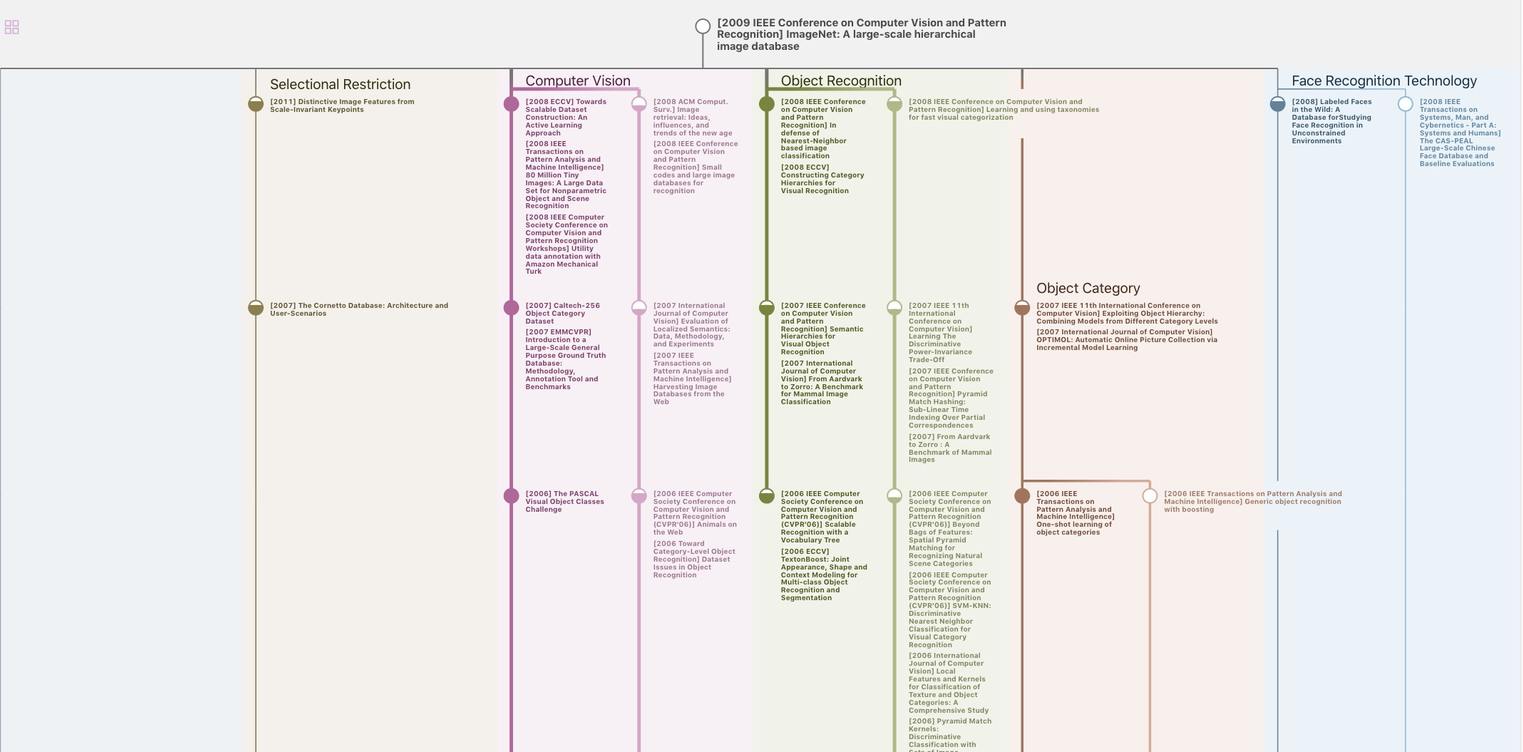
生成溯源树,研究论文发展脉络
Chat Paper
正在生成论文摘要