Computing Linkage Disequilibrium Aware Genome Embeddings using Autoencoders
biorxiv(2023)
摘要
Motivation The completion of the human genome has paved the way for genome-wide association studies (GWAS), which have already succeeded in explaining certain proportions of heritability. GWAS are not optimally suited to detect potential non-linear effects in disease risk, possibly hidden in non-additive interactions (epistasis). Alternative methods for epistasis detection using e.g. deep neural networks are currently under active development. Despite their promise to scale to high-dimensional data, deep neural networks (DNNs) are constrained by finite computational resources, which can be rapidly depleted due to increasing complexity with the sheer size of the genome. Besides, the curse of dimensionality complicates the task of capturing meaningful genetic patterns for DNNs; therefore calls for precaution in the form of dimensionality reduction.
Results We propose a method to compress genotyping data, involving single nucleotide polymorphisms (SNPs), while leveraging the LD structure in the genome and preserving non-linear relations among variants. This method involves clustering correlated SNPs into haplotype blocks and training per-block autoencoders that are able to learn a compressed representation of the block’s relevant genetic content. We provide an adjustable autoencoder design to accommodate diverse blocks and to bypass extensive hyperparameter tuning. We applied this method to genotyping data from Project MinE which involves a total of 23,209 ALS cases and 90,249 healthy controls. We compressed the haplotype blocks of an entire chromosome using our autoencoder-based approach, and show that this leads to more than 99% average test reconstruction accuracy - i.e. minimal information loss - while compressing the input to nearly 10% of the original size. We demonstrate that haplotype-block based autoencoders outperform their linear alternative Principal Component Analysis (PCA) by approximately 3% chromosome-wide accuracy of reconstructed variants. To the extent of our knowledge, our approach is the first to simultaneously leverage genome haplotype structure and deep neural networks for dimensionality reduction of genetic data.
Availability and Implementation Data used in this study are available for academic use through the Project MinE Consortium at . Data accessibility is contingent upon any terms or requirements specified by the source studies. Codes for data compression are available at .
### Competing Interest Statement
The authors have declared no competing interest.
更多查看译文
AI 理解论文
溯源树
样例
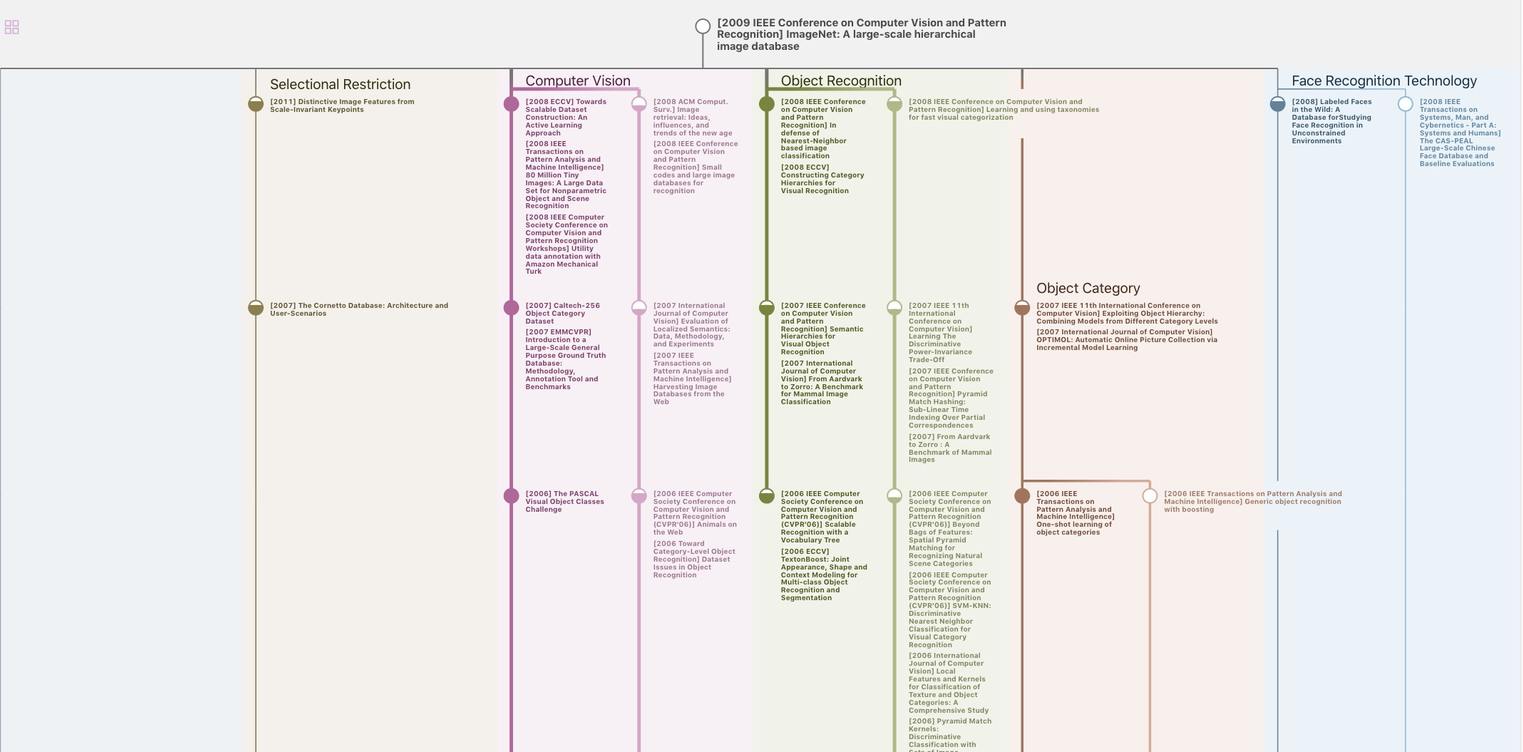
生成溯源树,研究论文发展脉络
Chat Paper
正在生成论文摘要