DeepSVC: Deep Scalable Video Coding for Both Machine and Human Vision
MM '23: Proceedings of the 31st ACM International Conference on Multimedia(2023)
摘要
Nowadays, end-to-end video coding for both machine and human vision has become an emerging research topic. In complicated systems such as large-scale internet of video things (IoVT), feature streams and video streams can be separately encoded and delivered for machine judgement and human viewing. In this paper, we propose a deep scalable video codec (DeepSVC) to support three-layer scalability from machine to human vision. First, we design a semantic layer that encodes semantic features extracted from the captured video for machine analysis. This layer employs a conditional semantic compression (CSC) method to remove redundancies between semantic features. Second, we design a structure layer that can be combined with semantic layer to predict the captured video at a low quality. This layer effectively estimates video frames based on semantic layer with an interlayer frame prediction (IFP) network. Third, we design a texture layer that can be combined with the above two layers to reconstruct high-quality video signals. This layer also takes advantage of the IFP network to improve its coding efficiency. In large-scale IoVT systems, DeepSVC can deliver semantic layer for regular use and transmit the other layers on demand. Experimental results indicate that the proposed DeepSVC outperforms popular codecs for machine and human vision. Compared with scalable extension of H.265/HEVC (SHVC), the proposed DeepSVC reduces average bit-per-pixel (bpp) by 25.51%/27.63%/59.87% at the same mAP/PSNR/MS-SSIM. Sourcecode is available at: https://github.com/LHB116/DeepSVC.
更多查看译文
AI 理解论文
溯源树
样例
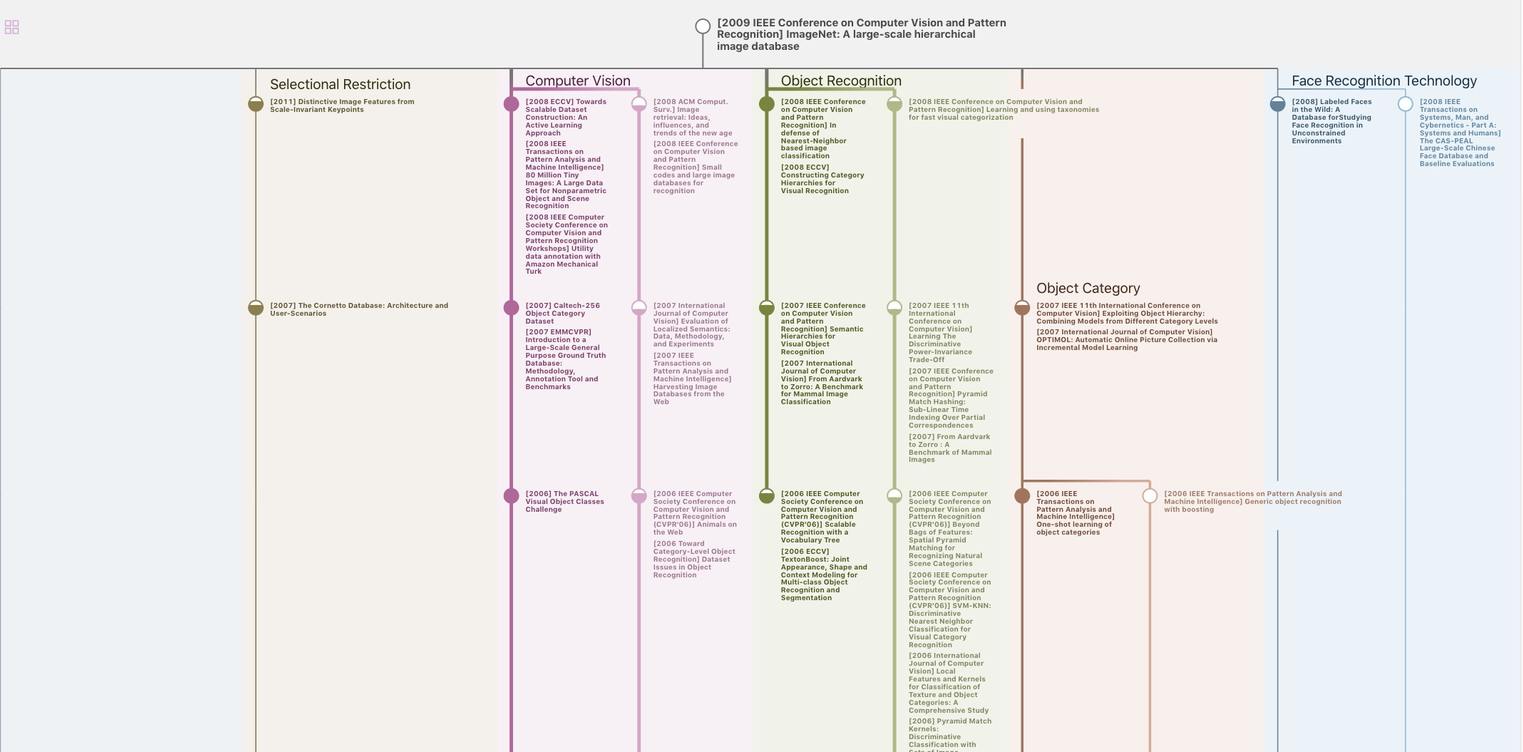
生成溯源树,研究论文发展脉络
Chat Paper
正在生成论文摘要