ELFIC: A Learning-based Flexible Image Codec with Rate-Distortion-Complexity Optimization
MM '23: Proceedings of the 31st ACM International Conference on Multimedia(2023)
摘要
Learning-based image coding has attracted increasing attentions for its higher compression efficiency than reigning image codecs. However, most existing learning-based codecs do not support variable rates with a single encoder; their decoders are also of fixed, high computational complexity. In this paper, we propose an End-to-end, Learning-based and Flexible Image Codec (ELFIC) that supports variable rate and flexible decoding complexity. First, we propose a general image codec with Nonlinear Feature Fusion Transform (NFFT) as nonlinear transforms to improve its Rate-Distortion (RD) performance. Second, we propose an Instance-aware Decoding Complexity Allocation (IDCA) approach, which exploits image contents for a tradeoff between reconstruction quality and computational complexity in the decoding process. Third, we propose an RD-Complexity (RDC) optimization algorithm, which maximizes the image quality under given rate and complexity constraints for the whole framework. Experimental results show that ELFIC achie-ves variable rate, flexible decoding complexity with the state-of-the-art RD performance. It also supports a more efficient decoding process by focusing on image contents. Source codes are available at https://github.com/Zhichen-Zhang/ELFIC-Image-Compression.
更多查看译文
AI 理解论文
溯源树
样例
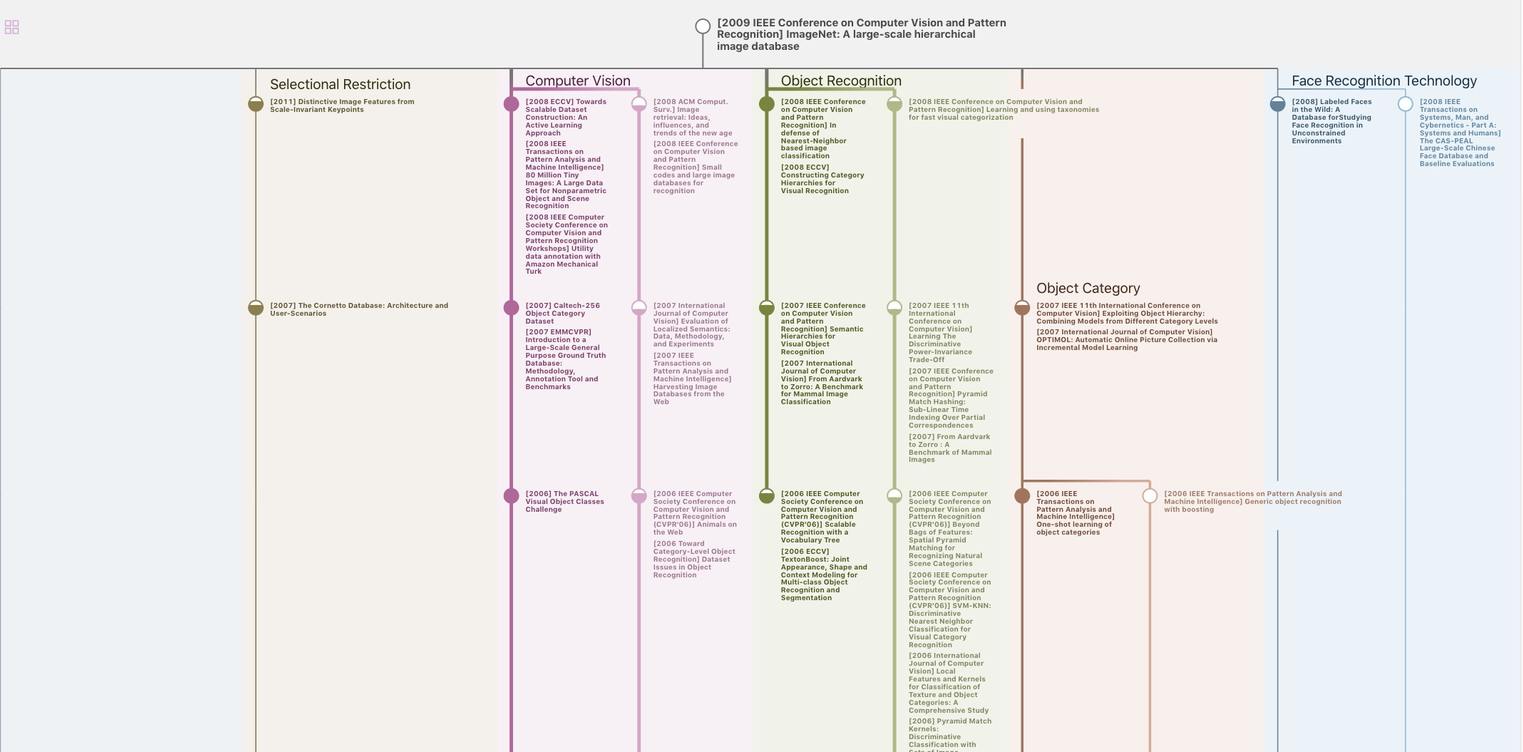
生成溯源树,研究论文发展脉络
Chat Paper
正在生成论文摘要