Causal Intervention for Sparse-View Gait Recognition
MM '23: Proceedings of the 31st ACM International Conference on Multimedia(2023)
摘要
Gait recognition aims at identifying individuals by unique walking patterns at a long distance. However, prevailing methods suffer from a large degradation when applied to large-scale surveillance systems. We find a significant cause of this issue is that previous methods heavily rely on full-view person annotations to reduce view differences by pulling closer the anchor to positive samples from different viewpoints. But, subjects under in-the-wild scenarios usually have only a limited number of sequences from different viewpoints. As a result, the available viewpoints of each subject are sparse compared to the whole dataset, and simply minimizing intra-identity differences cannot well reducing the view differences in the whole dataset. In this work, we formulate this overlooked problem as Sparse-View Gait Recognition and provide a comprehensive analysis of it by a Structural Causal Model for causalities among latent features, view distribution, and labels. Based on our analysis, we propose a simple yet effective method that enables networks to learn a more robust representation among different views. Specifically, our method consists of two parts: 1) an effective metric learning algorithmic implementation based on the backdoor adjustment, which improves the consistency of representations among different views; 2) an unsupervised view cluster algorithm to discover and identify the most influential view contexts. We evaluate the effectiveness of our method on popular GREW, Gait3D, CASIA-B, and OU-MVLP, showing that our method consistently outperforms baselines and achieves state-of-the-art performance. The code will be available at https://github.com/wj1tr0y/GaitCSV.
更多查看译文
AI 理解论文
溯源树
样例
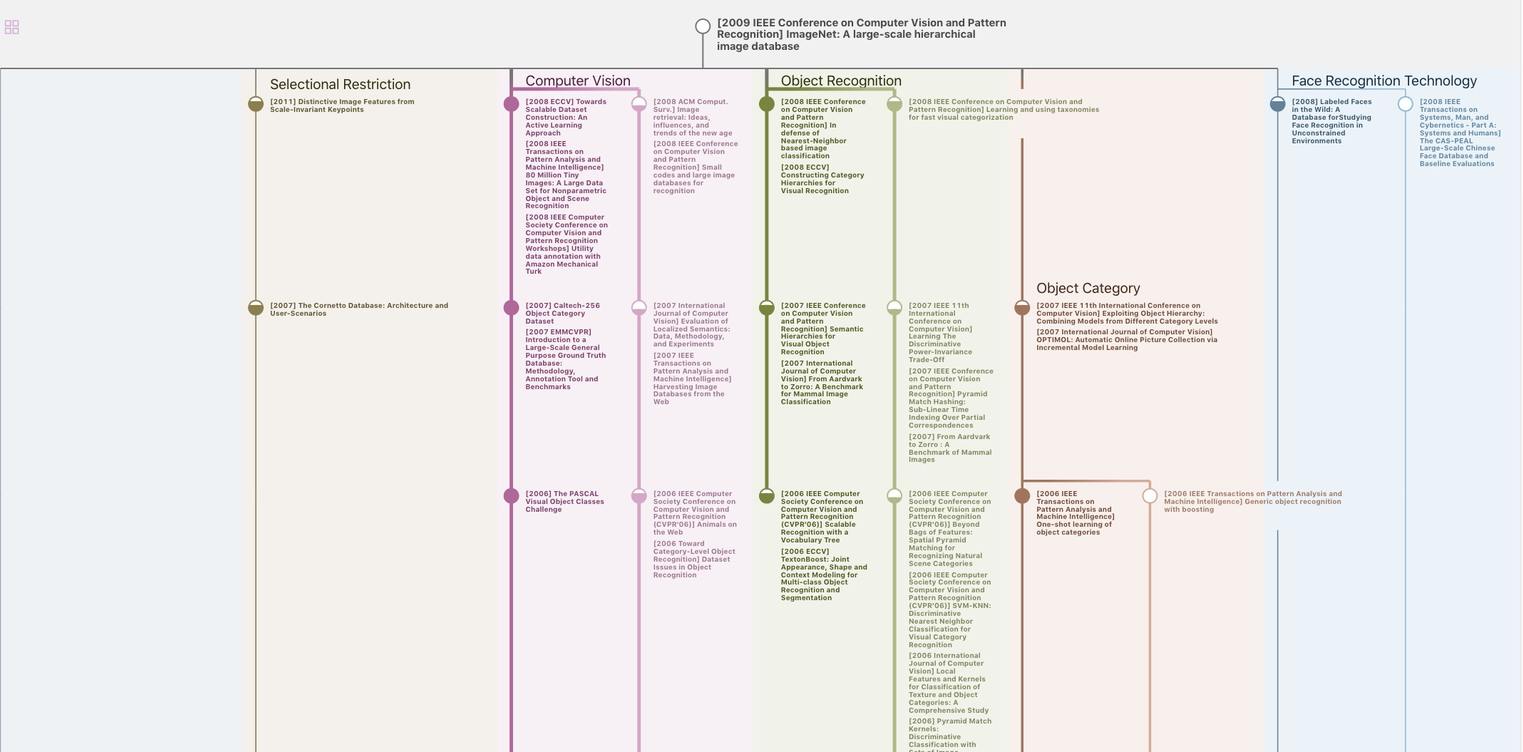
生成溯源树,研究论文发展脉络
Chat Paper
正在生成论文摘要