Attentional State Classification Using Amplitude and Phase Feature Extraction Method Based on Filter Bank and Riemannian Manifold
IEEE transactions on neural systems and rehabilitation engineering : a publication of the IEEE Engineering in Medicine and Biology Society(2023)
摘要
As a significant aspect of cognition, attention has been extensively studied and numerous measurements have been developed based on brain signal processing. Although existing attentional state classification methods have achieved good accuracy by extracting a variety of handcrafted features, spatial features have not been fully explored. This paper proposes an attentional state classification method based on Riemannian manifold to utilize spatial information. Based on the concept of Riemannian manifold of symmetric positive definite (SPD) matrix, the proposed method exploits the structure of covariance matrix to extract spatial features instead of using spatial filters. Specifically, Riemannian distances from intra-class Riemannian means are extracted as features for their robustness. To fully extend the potential of electroencephalograph (EEG) signal, both amplitude and phase information is utilized. In addition, to solve the variance of frequency bands, a filter bank is employed to process the signal of different frequency bands separately. Finally, features are fed into a support vector machine with a polynomial kernel to obtain classification results. The proposed attentional state classification using amplitude and phase feature extraction method based on filter bank and Riemannian manifold (AP-FBRM) method is evaluated on two open datasets including EEG data of 29 and 26 subjects. According to the experimental results, the optimal set of filter bank and the optimal technique to extract features containing both amplitude and phase information are determined. The proposed method respectively achieves accuracies of 88.06% and 80.00% and outperforms 8 baseline methods, which manifests that the proposed method creates an efficient way to recognize attentional state.
更多查看译文
关键词
Feature extraction,Manifolds,Electroencephalography,Filter banks,Covariance matrices,Symmetric matrices,Cognition,Attentional state classification,brain-computer interfaces (BCI),Riemannian manifold,EEG,filter bank
AI 理解论文
溯源树
样例
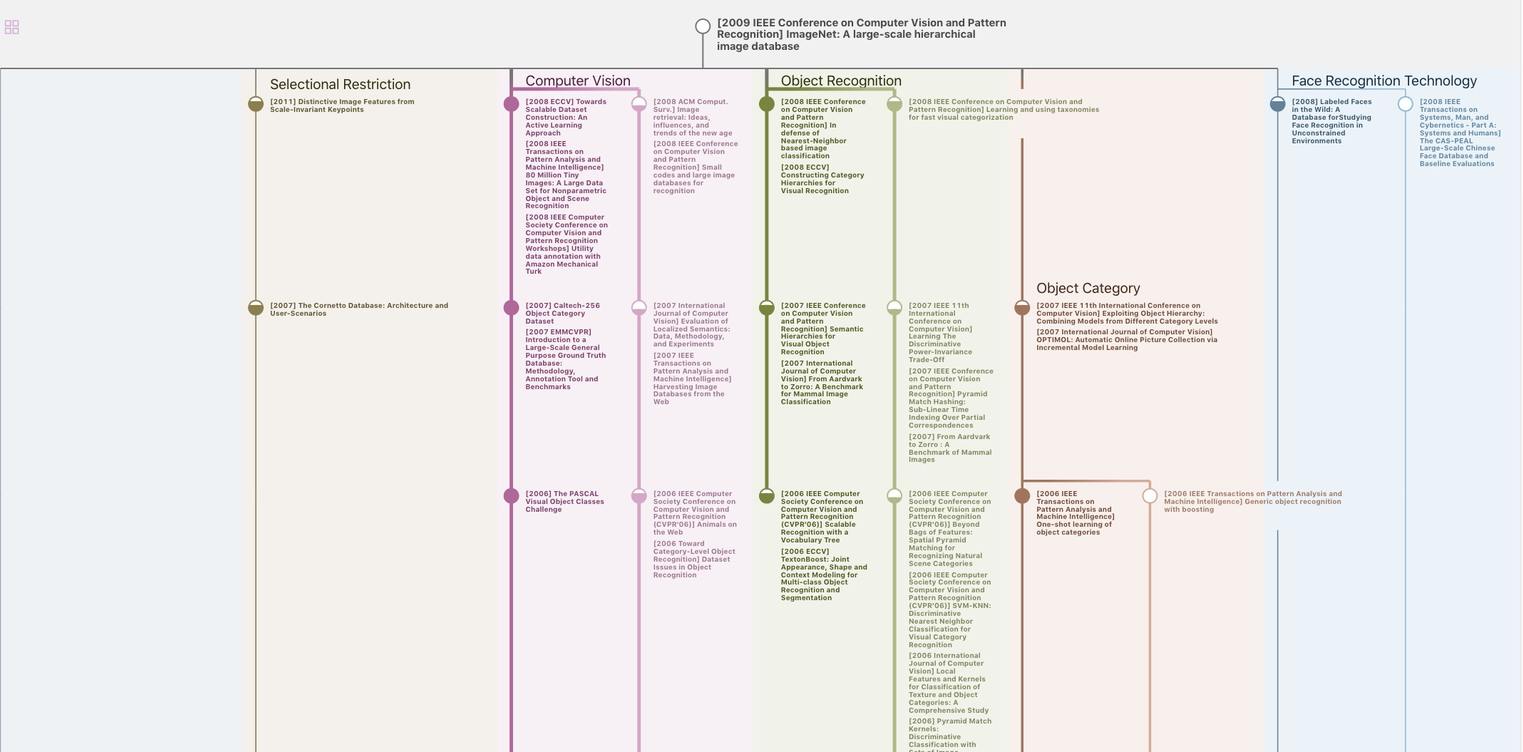
生成溯源树,研究论文发展脉络
Chat Paper
正在生成论文摘要