Box-supervised dynamical instance segmentation for in-field cotton
COMPUTERS AND ELECTRONICS IN AGRICULTURE(2023)
摘要
Crop growing information is important for precision agriculture. Instance segmentation can be employed to extract not only the location and quantity information of objects from images, but also the individual phenotype information of each target. However, when it is applied on real in-field images, complex environment increases the cost of annotating. Besides, it is hard for instance segmentation network with complicated structure to be deployed in real application scenarios. To cost-efficiently extract data used for precision management from field images, this paper proposes a novel model based on dense prediction and conditionally parameterized convolution termed Box-supervised dynamical regression instance segmentation net(BDRISNet) for segmenting individuals from image in a simple way, using box-level supervision. By formulating segmentation into dense regression from each pixel to the location of its instance indicators, mask features can be generated without redundant convolution branch. The compact construction of proposed network achieves efficient inference for segmentation. Robustness and accuracy of proposed method are evaluated on an in-field cotton boll image dataset we provide. Comparison experiments with other state-of-the-arts demonstrate that our method has an average improvement of 3.13%, 5.55%, 7.08% in AP, AP50 and AP75. Furthermore, predictions of proposed method have desirable and balanced recall and precision.
更多查看译文
关键词
Cotton,Box-supervised instance segmentation,Conditionally parameterized convolution
AI 理解论文
溯源树
样例
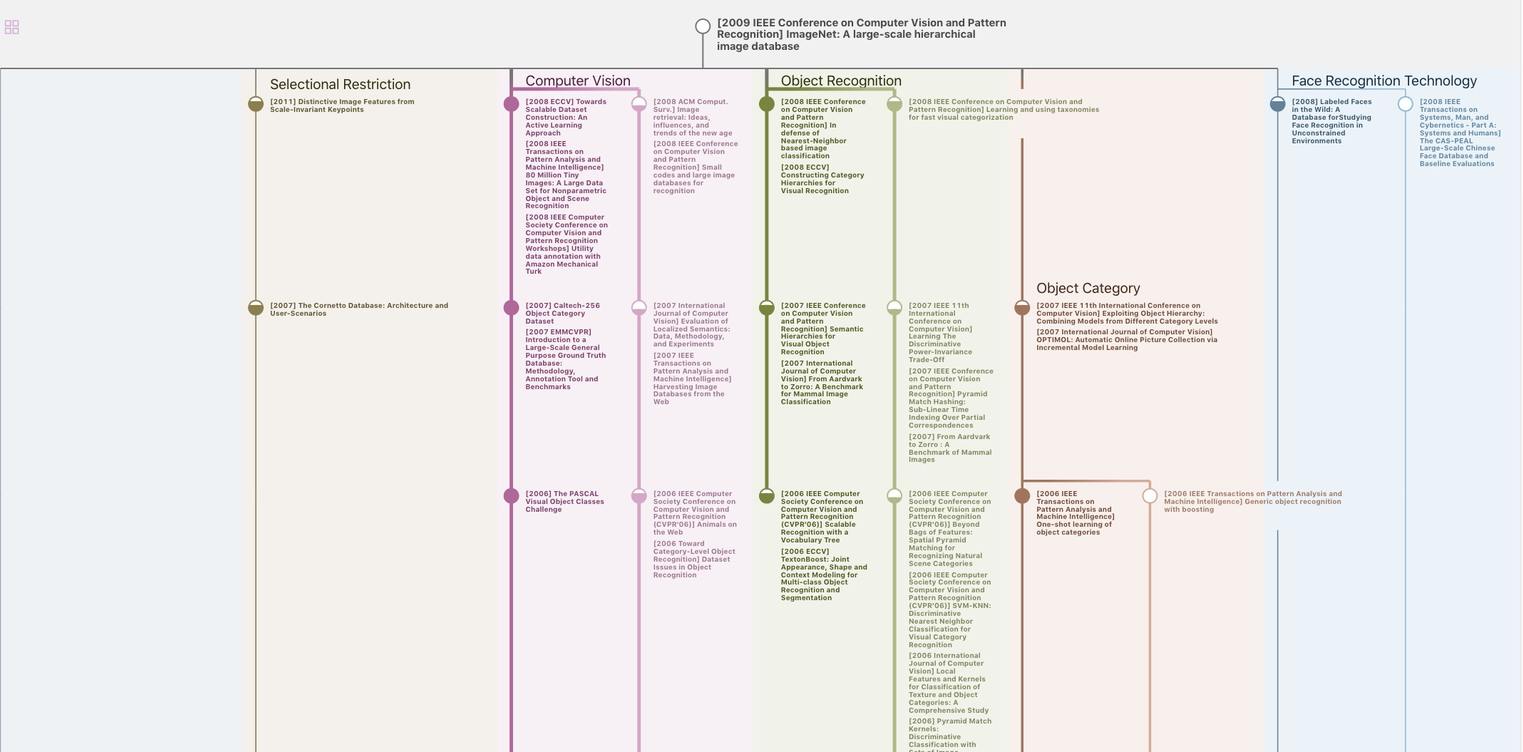
生成溯源树,研究论文发展脉络
Chat Paper
正在生成论文摘要