Electric submersible pump anomaly monitoring based on unsupervised multivariate streaming data analysis
2023 CAA Symposium on Fault Detection, Supervision and Safety for Technical Processes (SAFEPROCESS)(2023)
摘要
Electric Submersible Pump is an essential artificial lift equipment in the petroleum industry, and its anomaly detection is of great significance for maintaining system performance and ensuring normal production. In this paper, we propose a novel unsupervised multivariate streaming data analysis approach that directly uses the raw structured data as input. It eliminates the need for complex modeling and parameter optimization. Then, metrics that can reflect the state of the system are built through feature mapping and fusion. Based on the fused metric, the smoothness of the metric is monitored in real-time by the control chart detection method, which does not require pre-training of known label samples or any domain knowledge. Finally, the feature selection algorithm ANOVA is introduced into the algorithm to locate the most relevant features to anomalies. We evaluate the algorithm using the ESP signals from three regions in Penglai, Bohai Oilfield, China. The experimental results show that the algorithm proposed in this paper can capture system performance anomalies in time and realize the location of suspicious features. Moreover, the inexpensive computational costs and the second-level inspection speed make it ideal for on-site deployment.
更多查看译文
关键词
Electric submersible pump,Anomaly detection,Unsupervised,Streaming data,Fault location
AI 理解论文
溯源树
样例
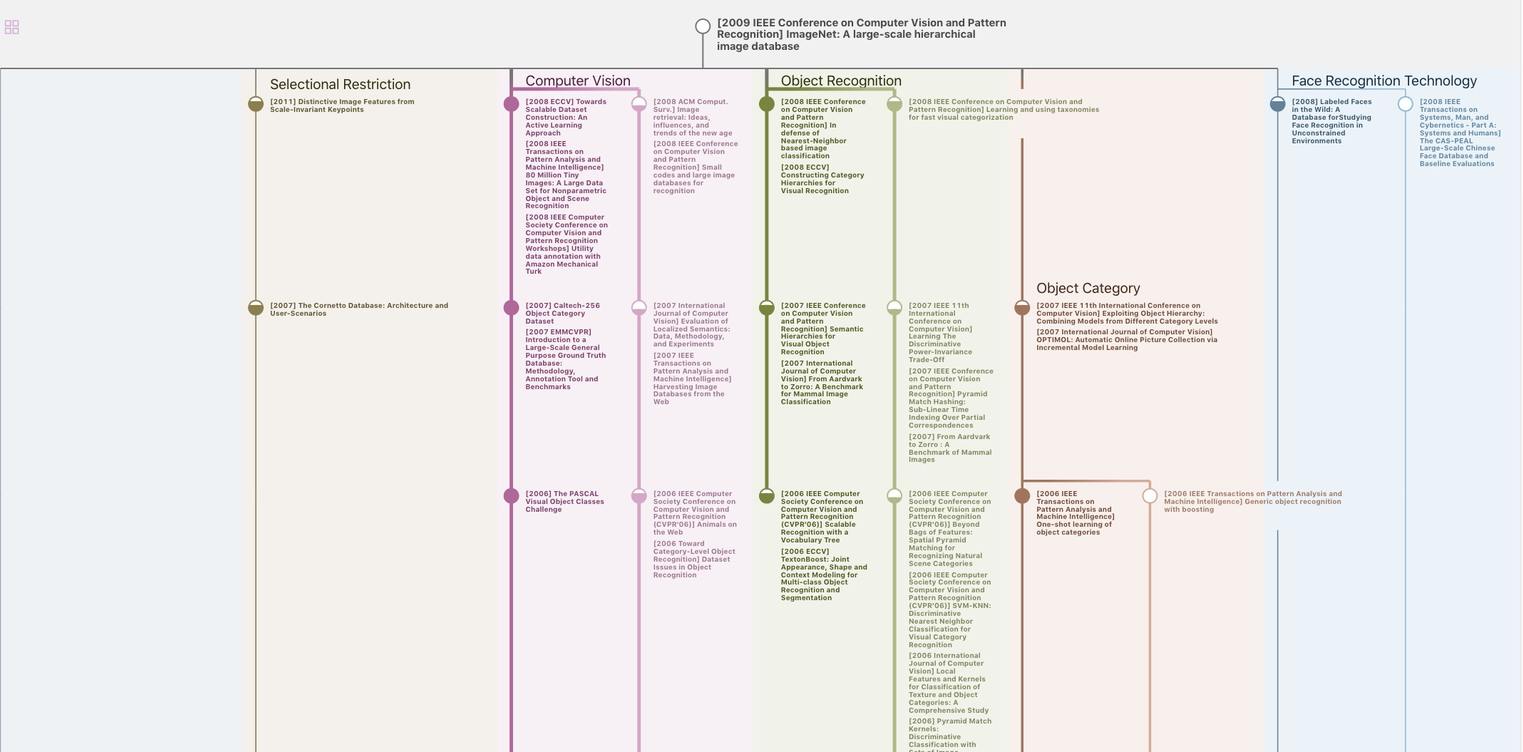
生成溯源树,研究论文发展脉络
Chat Paper
正在生成论文摘要